Phoneme transcription of endangered languages: an evaluation of recent ASR architectures in the single speaker scenario
FINDINGS OF THE ASSOCIATION FOR COMPUTATIONAL LINGUISTICS (ACL 2022)(2022)
摘要
Transcription is often reported as the bottleneck in endangered language documentation, requiring large efforts from scarce speakers and transcribers. In general, automatic speech recognition (ASR) can be accurate enough to accelerate transcription only if trained on large amounts of transcribed data. However, when a single speaker is involved, several studies have reported encouraging results for phonetic transcription even with small amounts of training. Here we expand this body of work on speaker-dependent transcription by comparing four ASR approaches, notably recent transformer and pretrained multilingual models, on a common dataset of 11 languages. To automate data preparation, training and evaluation steps, we also developed a phoneme recognition setup which handles morphologically complex languages and writing systems for which no pronunciation dictionary exists. We find that fine-tuning a multilingual pretrained model yields an average phoneme error rate (PER) of 15% for 6 languages with 99 minutes or less of transcribed data for training. For the 5 languages with between 100 and 192 minutes of training, we achieved a PER of 8.4% or less. These results on a number of varied languages suggest that ASR can now significantly reduce transcription efforts in the speaker-dependent situation common in endangered language work.
更多查看译文
关键词
endangered languages,transcription,recent asr architectures,single speaker scenario
AI 理解论文
溯源树
样例
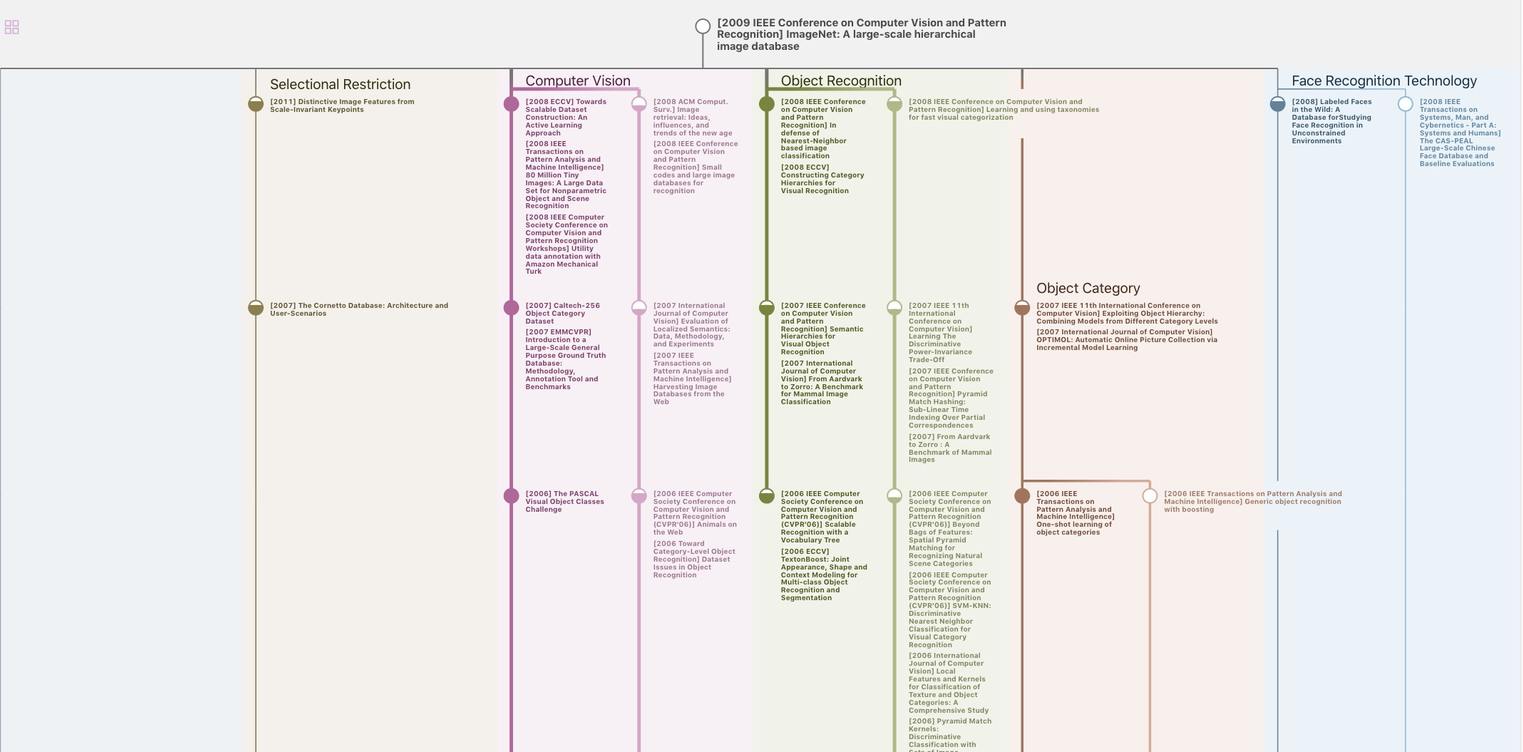
生成溯源树,研究论文发展脉络
Chat Paper
正在生成论文摘要