BARS: Towards Open Benchmarking for Recommender Systems
SIGIR '22: Proceedings of the 45th International ACM SIGIR Conference on Research and Development in Information Retrieval(2022)
摘要
The past two decades have witnessed the rapid development of personalized recommendation techniques. Despite the significant progress made in both research and practice of recommender systems, to date, there is a lack of a widely-recognized benchmarking standard in this field. Many of the existing studies perform model evaluations and comparisons in an ad-hoc manner, for example, by employing their own private data splits or using a different experimental setting. However, such conventions not only increase the difficulty in reproducing existing studies, but also lead to inconsistent experimental results among them. This largely limits the credibility and practical value of research results in this field. To tackle these issues, we present an initiative project aimed for open benchmarking for recommender systems. In contrast to some earlier attempts towards this goal, we take one further step by setting up a standardized benchmarking pipeline for reproducible research, which integrates all the details about datasets, source code, hyper-parameter settings, running logs, and evaluation results. The benchmark is designed with comprehensiveness and sustainability in mind. It spans both matching and ranking tasks, and also allows anyone to easily follow and contribute. We believe that our benchmark could not only reduce the redundant efforts of researchers to re-implement or re-run existing baselines, but also drive more solid and reproducible research on recommender systems.
更多查看译文
关键词
Recommender systems, benchmarking, CTR prediction, item matching, collaborative filtering
AI 理解论文
溯源树
样例
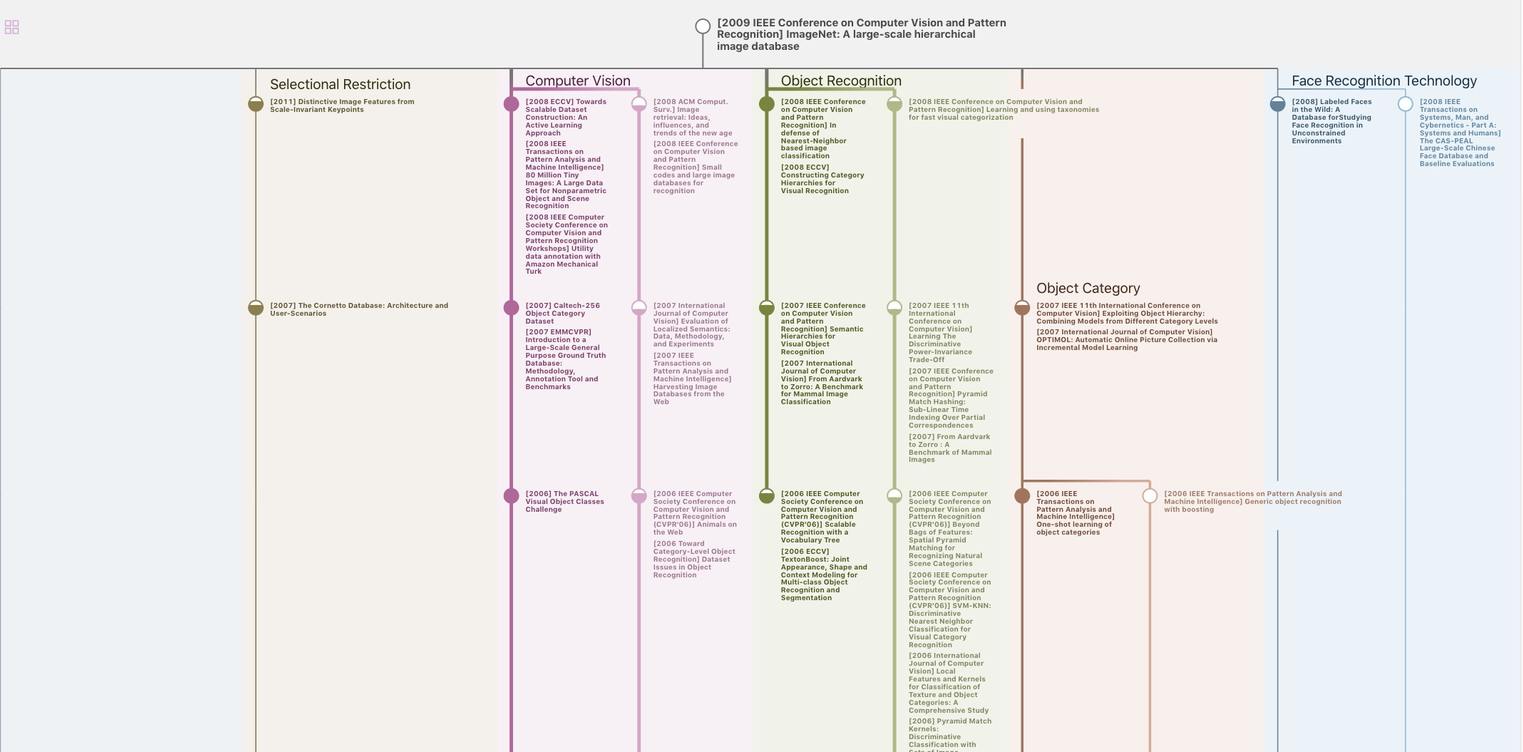
生成溯源树,研究论文发展脉络
Chat Paper
正在生成论文摘要