Improving Micro-video Recommendation via Contrastive Multiple Interests
SIGIR '22: Proceedings of the 45th International ACM SIGIR Conference on Research and Development in Information Retrieval(2022)
摘要
With the rapid increase of micro-video creators and viewers, how to make personalized recommendations from a large number of candidates to viewers begins to attract more and more attention. However, existing micro-video recommendation models rely on expensive multi-modal information and learn an overall interest embedding that cannot reflect the user's multiple interests in micro-videos. Recently, contrastive learning provides a new opportunity for refining the existing recommendation techniques. Therefore, in this paper, we propose to extract contrastive multi-interests and devise a micro-video recommendation model CMI. Specifically, CMI learns multiple interest embeddings for each user from his/her historical interaction sequence, in which the implicit orthogonal micro-video categories are used to decouple multiple user interests. Moreover, it establishes the contrastive multi-interest loss to improve the robustness of interest embeddings and the performance of recommendations. The results of experiments on two micro-video datasets demonstrate that CMI achieves state-of-the-art performance over existing baselines.
更多查看译文
关键词
Micro-video recommendation, Contrastive learning, Multi-interest learning
AI 理解论文
溯源树
样例
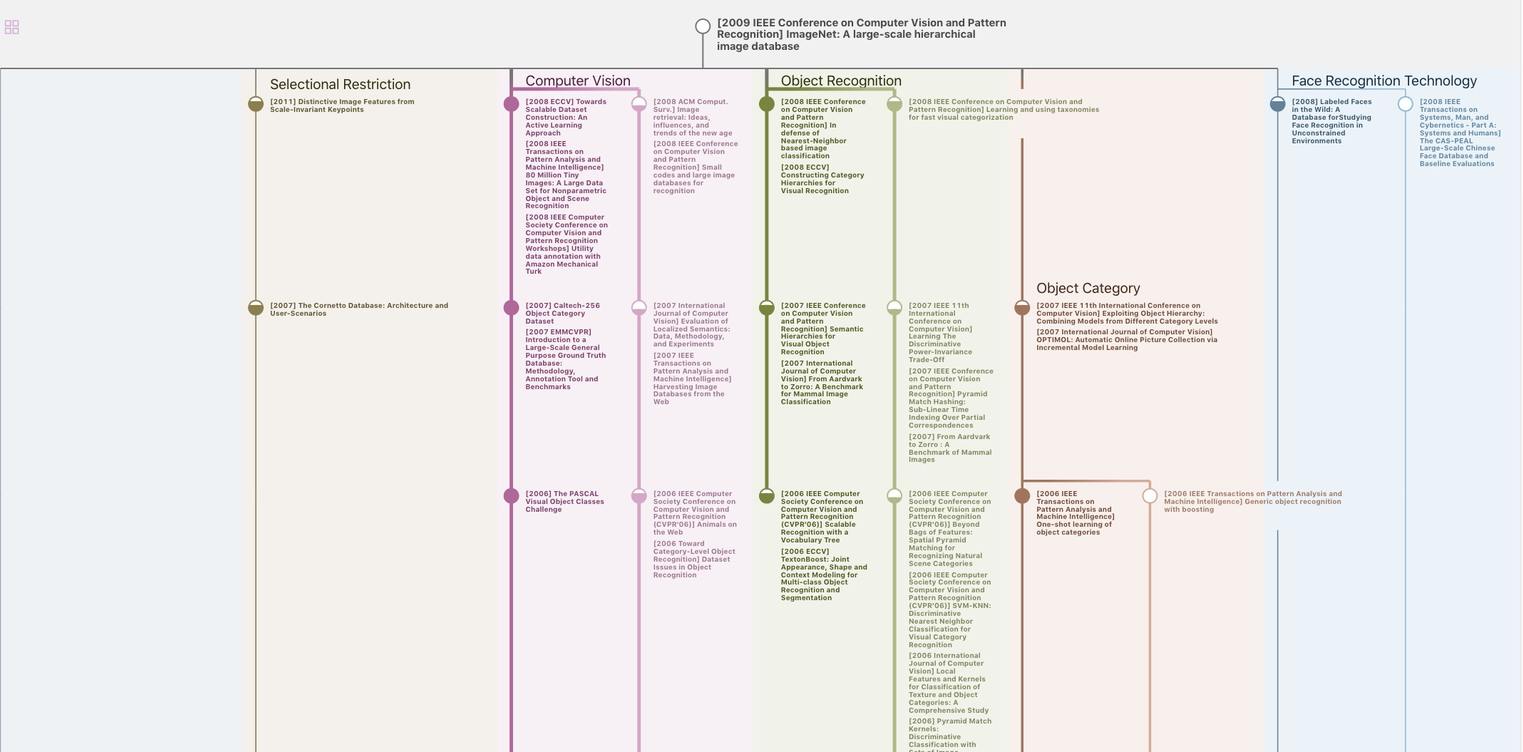
生成溯源树,研究论文发展脉络
Chat Paper
正在生成论文摘要