A Boosting Algorithm for Positive-Unlabeled Learning
arxiv(2022)
摘要
Positive-unlabeled (PU) learning deals with binary classification problems when only positive (P) and unlabeled (U) data are available. Many recent PU methods are based on neural networks, but little has been done to develop boosting algorithms for PU learning, despite boosting algorithms' strong performance on many fully supervised classification problems. In this paper, we propose a novel boosting algorithm, AdaPU, for PU learning. Similarly to AdaBoost, AdaPU aims to optimize an empirical exponential loss, but the loss is based on the PU data, rather than on positive-negative (PN) data. As in AdaBoost, we learn a weighted combination of weak classifiers by learning one weak classifier and its weight at a time. However, AdaPU requires a very different algorithm for learning the weak classifiers and determining their weights. This is because AdaPU learns a weak classifier and its weight using a weighted positive-negative (PN) dataset with some negative data weights $-$ the dataset is derived from the original PU data, and the data weights are determined by the current weighted classifier combination, but some data weights are negative. Our experiments showed that AdaPU outperforms neural networks on several benchmark PU datasets, including a large-scale challenging cyber security dataset.
更多查看译文
关键词
boosting algorithm,learning,positive-unlabeled
AI 理解论文
溯源树
样例
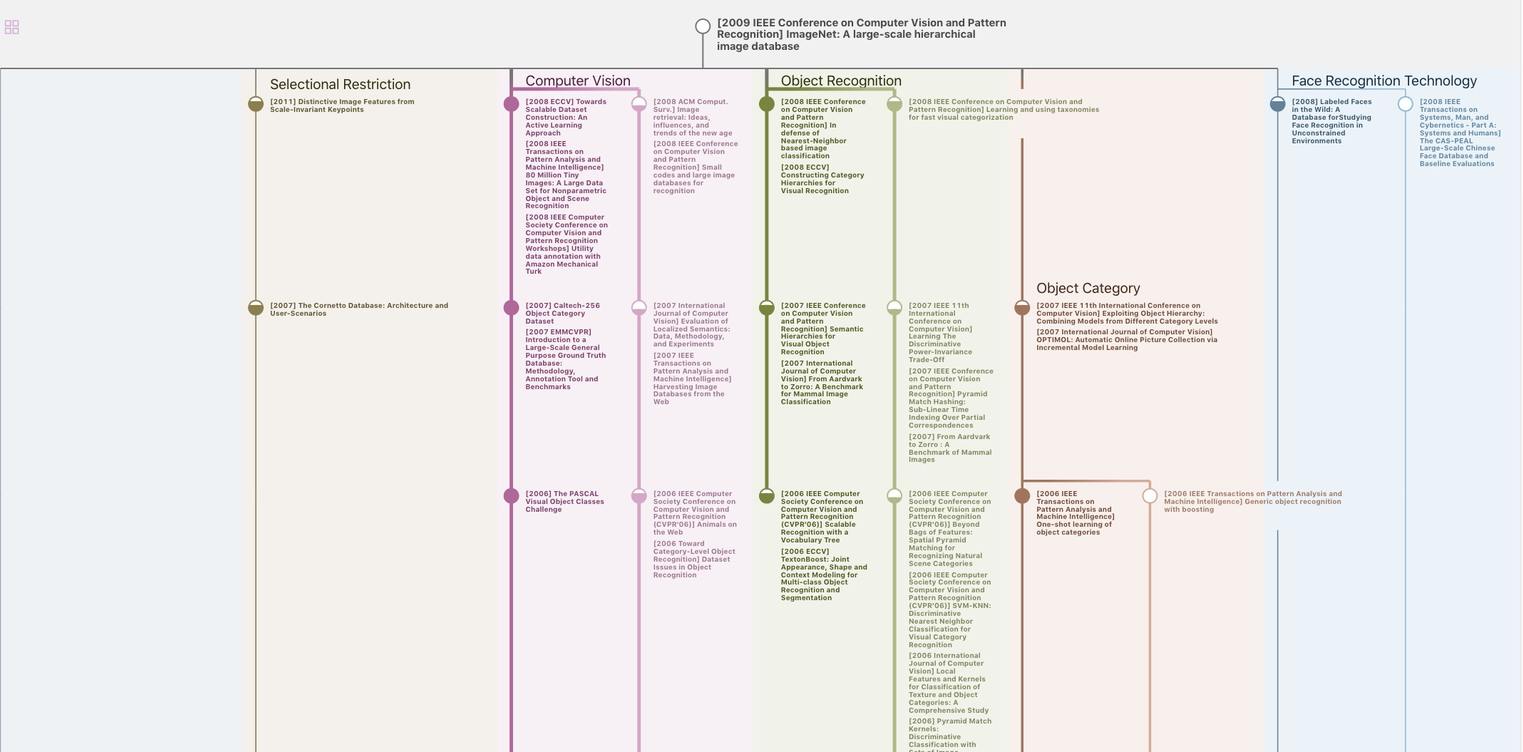
生成溯源树,研究论文发展脉络
Chat Paper
正在生成论文摘要