Neural ODE Control for Trajectory Approximation of Continuity Equation
IEEE CONTROL SYSTEMS LETTERS(2022)
摘要
We consider the controllability problem for the continuity equation, corresponding to neural ordinary differential equations (ODEs), which describes how a probability measure is pushedforward by the flow. We show that the controlled continuity equation has very strong controllability properties. Particularly, a given solution of the continuity equation corresponding to a bounded Lipschitz vector field defines a trajectory on the set of probability measures. For this trajectory, we show that there exist piecewise constant training weights for a neural ODE such that the solution of the continuity equation corresponding to the neural ODE is arbitrarily close to it. As a corollary to this result, we establish that the continuity equation of the neural ODE is approximately controllable on the set of compactly supported probability measures that are absolutely continuous with respect to the Lebesgue measure.
更多查看译文
关键词
Neural networks, Controllability, Trajectory, Training, Topology, Particle measurements, Estimation, Distributed parameter systems, machine learning, neural networks
AI 理解论文
溯源树
样例
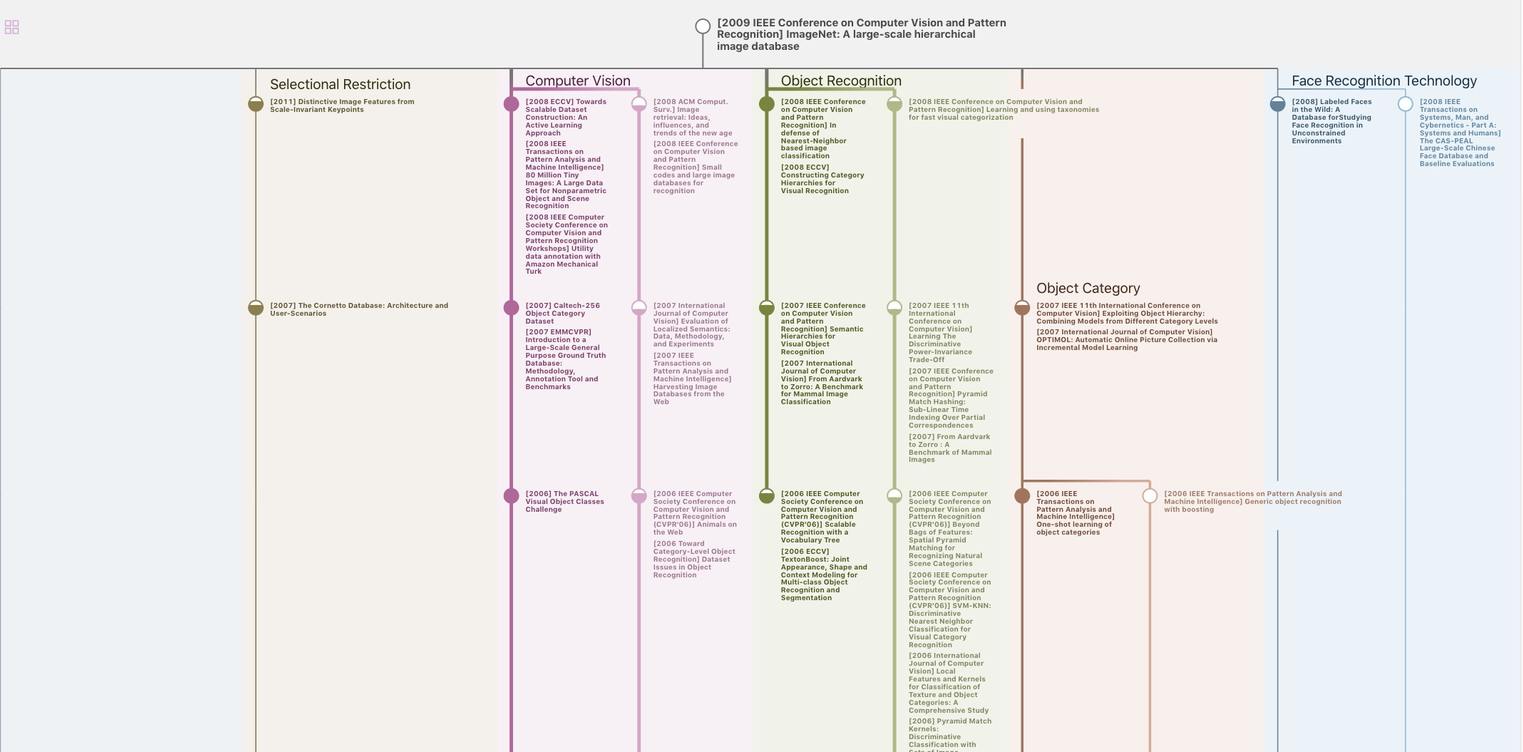
生成溯源树,研究论文发展脉络
Chat Paper
正在生成论文摘要