Poster Abstract: Data-Driven Estimation of Collision Risks for Autonomous Vehicles with Formal Guarantees*
Cyber-physical Systems(2022)
摘要
This work proposes a compositional data-driven approach for the formal estimation of collision risks for autonomous vehicles (AVs) with black-box dynamics acting in stochastic multi-agent environments. The proposed technique is based on the construction of sub-barrier certificates via a set of data collected from trajectories of each stochastic agent while providing a-priori guaranteed confidence on the data-driven estimation. In our proposed setting, we first cast the original collision risk problem of each agent as a robust optimization program (ROP) and provide a scenario optimization program (SOP) corresponding to the original ROP by collecting finite numbers of data from trajectories of each agent. We then establish a probabilistic bridge between the optimal value of SOP and that of ROP, and accordingly, formally construct a sub-barrier certificate for each unknown agent based on number of data and a required level of confidence. We eventually propose a compositional technique based on small-gain reasoning to quantify the collision risk for multi-agent AVs with some guaranteed confidence based on data-driven sub-barrier certificates of individual agents.
更多查看译文
关键词
Collision risks, Autonomous vehicles, Formal methods, Data-driven optimization, Compositional techniques
AI 理解论文
溯源树
样例
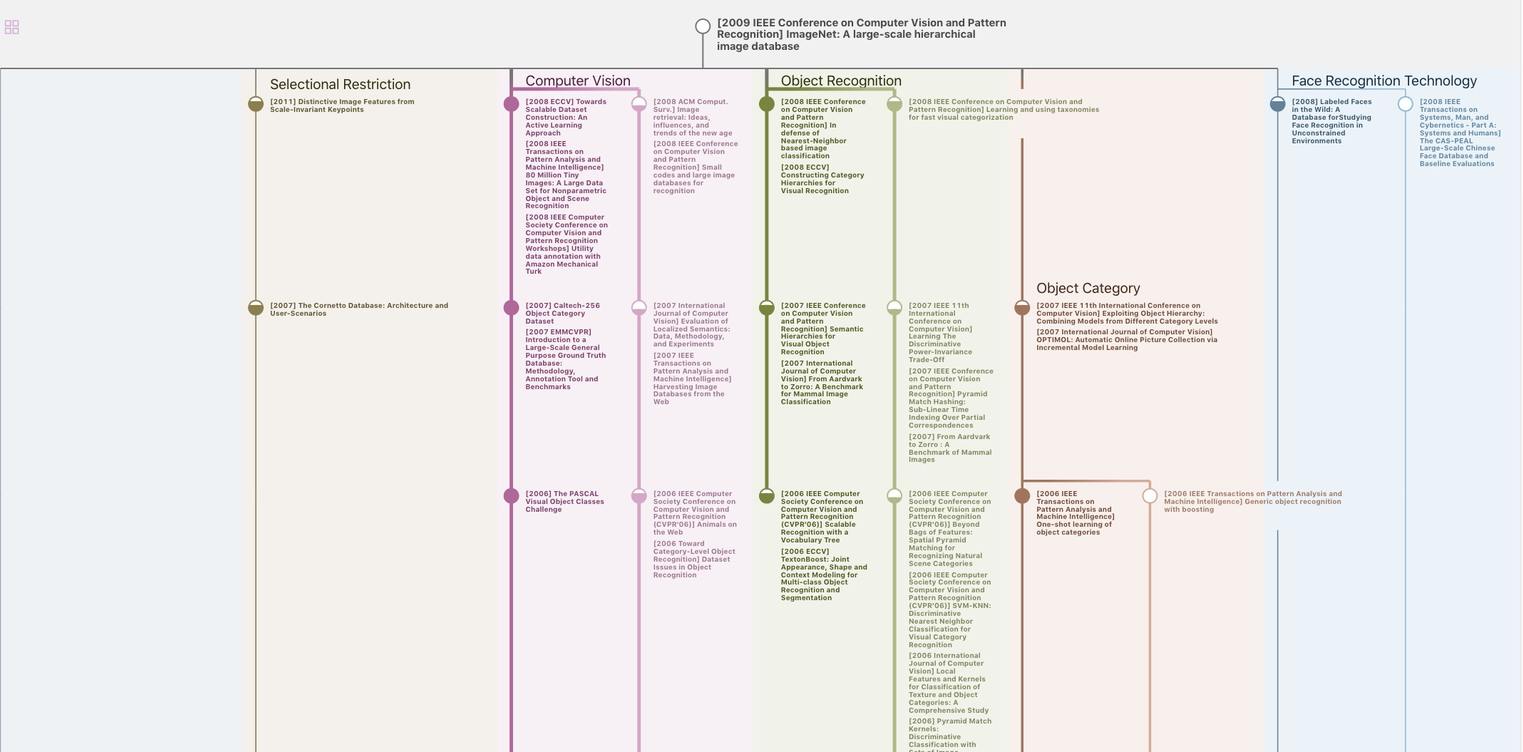
生成溯源树,研究论文发展脉络
Chat Paper
正在生成论文摘要