Control variates with a dimension reduced bayesian monte carlo sampler
International Journal for Uncertainty Quantification(2022)
Abstract
Evaluating expectations of random functions is an important task in many fields of science and engineering. In practice, such an expectation is often evaluated with the Monte Carlo methods that approximate the sought expectation with sample average. It is well known that the Monte Carlo methods typically suffer from slow convergence, making it especially unfriendly to problems where generating samples requires expensive computer simulations. An alternative to the standard Monte Carlo method is the so-called Bayesian Monte Carlo (BMC) algorithm, which formulates the expectation estimation as a Bayesian inference problem. As has been demonstrated in the literature, BMC is more efficient than standard Monte Carlo in a range of problems. However, a major limitation of BMC is that it models the integrand function as a Gaussian process which cannot handle problems of high dimension. In this work, we propose a method to address this issue. Specifically, we incorporate the BMC framework with a likelihood-based dimension reduction technique, which allows us to evaluate the expectation of functions with very high dimension. Then based on the dimension-reduced BMC, we propose a control variate scheme for problems where a direct use of dimension reduced BMC is not sufficiently accurate. We verify the effectiveness of the proposed method with both mathematical and practical examples.
MoreTranslated text
Key words
Bayesian Monte Carlo, control variate, dimension reduction, likelihood-acquired direction, Monte Carlo, Gaussian process, numerical integration
AI Read Science
Must-Reading Tree
Example
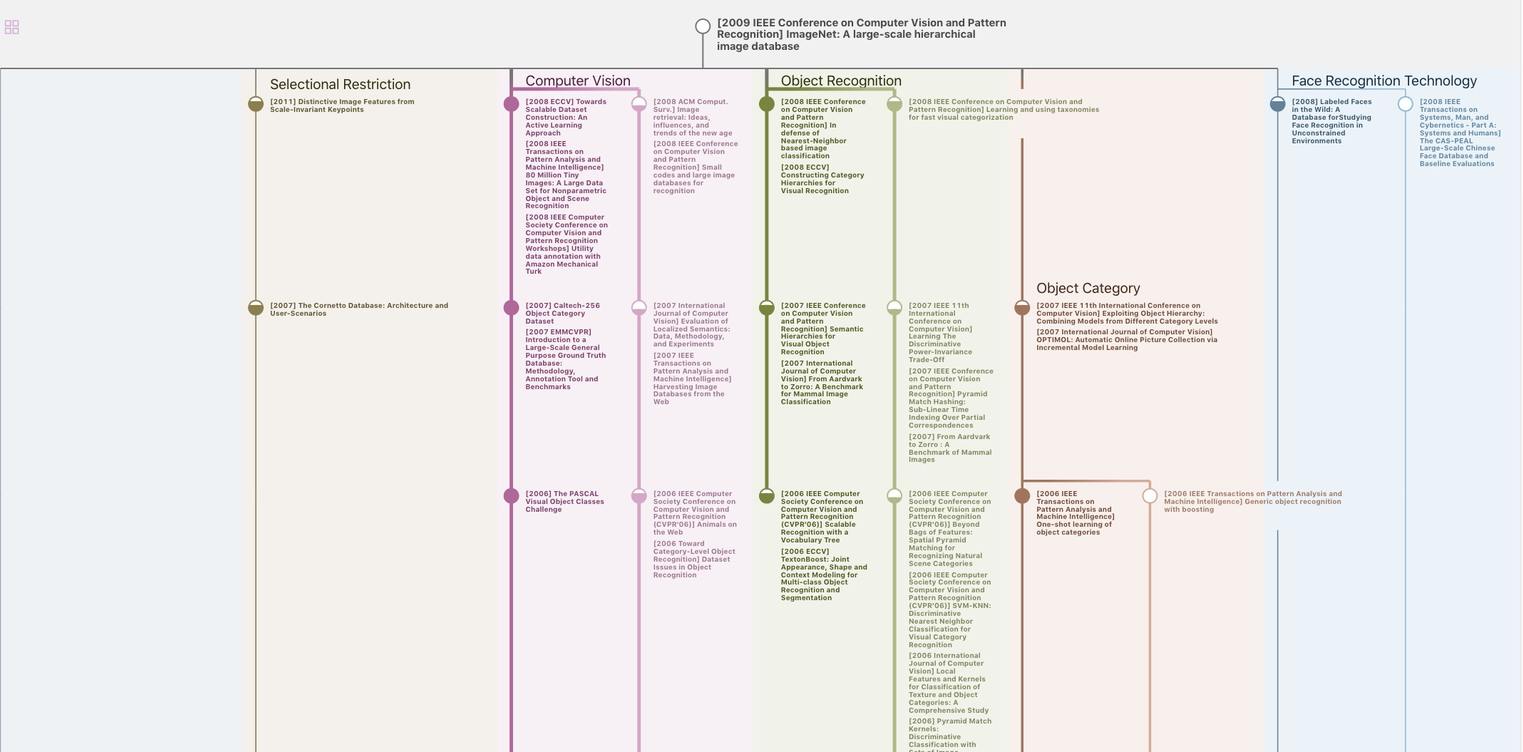
Generate MRT to find the research sequence of this paper
Chat Paper
Summary is being generated by the instructions you defined