MD-Unet: a deformable network for nasal cavity and paranasal sinus tumor segmentation
Signal, Image and Video Processing(2022)
Abstract
In recent years, with the rapid development of deep learning, medical image segmentation has brought breakthrough progress. U-Net has become the most prominent and popular deep network architecture in the field. Despite its overall excellent performance in medical image segmentation, we found that the classical U-Net architecture was insensitive to image details and had the problem of local and global consistency separation through experiments on nasal and paranasal sinus tumor datasets. To solve these problems, we propose an improved multi-scale neural network based on deformable convolution, called Multi-scale Deformable U-Net (MD-Unet). According to the spatial deformation characteristics of nasal cavity and paranasal sinus tumors, deformable convolution can be used to adaptively obtain the receiving field according to the shape of objects and extract features of different scales for fusion, so as to fully learn image details and improve feature extraction ability. We also use Tversky loss function to solve the problem of sample imbalance in the dataset, and obtain high sensitivity and generalization ability. Experimental results show that the proposed algorithm can effectively improve the segmentation accuracy of nasal and paranasal sinus tumors by 5.75%, 3.30%, 1.22% and 0.56% compared with U-Net, Res-Unet, Attention U-Net, and UNet++, respectively.
MoreTranslated text
Key words
Tumor of nasal cavity and paranasal sinus segmentation,U-Net,Deformable convolution,Tversky loss function
AI Read Science
Must-Reading Tree
Example
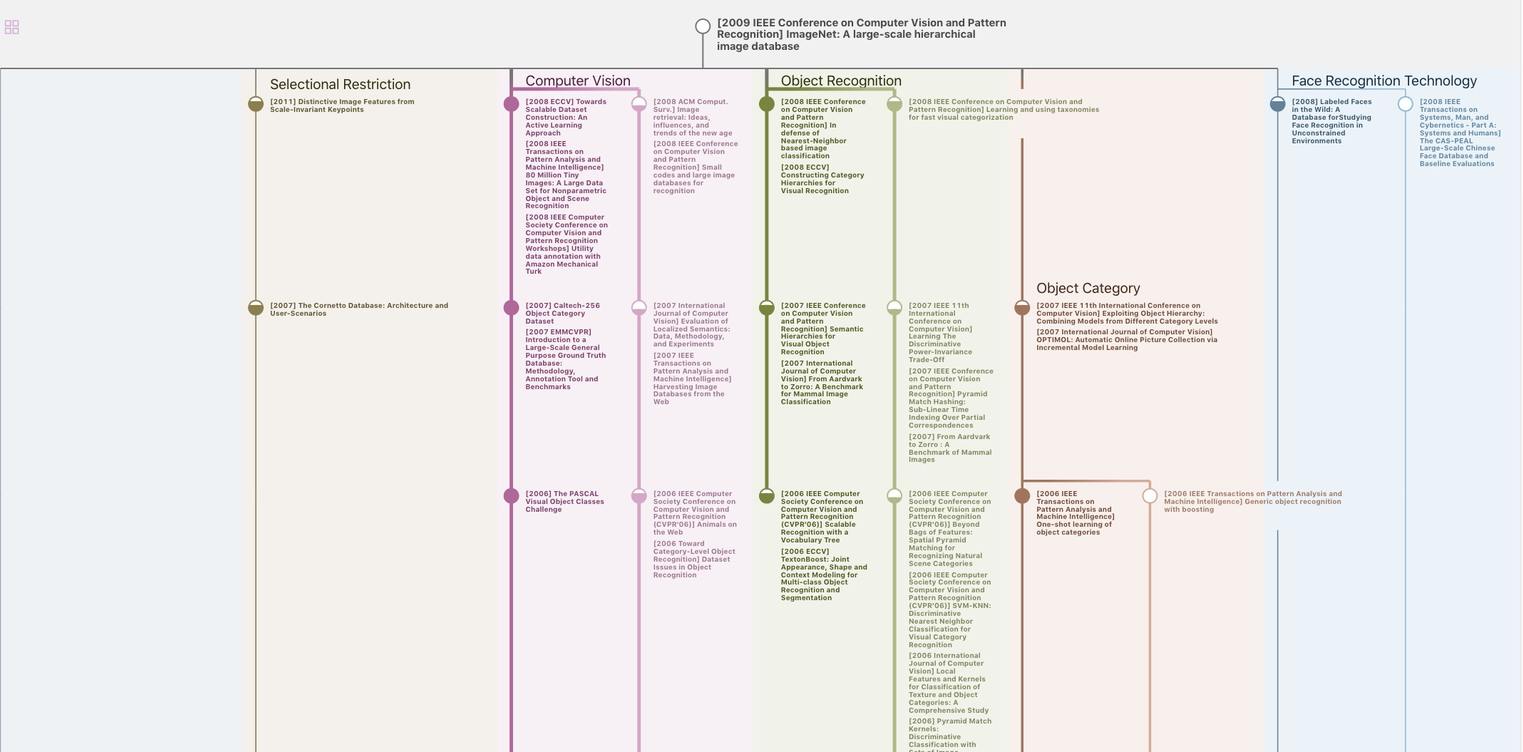
Generate MRT to find the research sequence of this paper
Chat Paper
Summary is being generated by the instructions you defined