Fair and green hyperparameter optimization via multi-objective and multiple information source Bayesian optimization
Machine Learning(2024)
Abstract
It has been recently remarked that focusing only on accuracy in searching for optimal Machine Learning models amplifies biases contained in the data, leading to unfair predictions and decision supports. Recently, multi-objective hyperparameter optimization has been proposed to search for Machine Learning models which offer equally Pareto-efficient trade-offs between accuracy and fairness. Although these approaches proved to be more versatile than fairness-aware Machine Learning algorithms—which instead optimize accuracy constrained to some threshold on fairness—their carbon footprint could be dramatic, due to the large amount of energy required in the case of large datasets. We propose an approach named FanG-HPO: fair and green hyperparameter optimization (HPO), based on both multi-objective and multiple information source Bayesian optimization. FanG-HPO uses subsets of the large dataset to obtain cheap approximations (aka information sources) of both accuracy and fairness, and multi-objective Bayesian optimization to efficiently identify Pareto-efficient (accurate and fair) Machine Learning models. Experiments consider four benchmark (fairness) datasets and four Machine Learning algorithms, and provide an assessment of FanG-HPO against both fairness-aware Machine Learning approaches and two state-of-the-art Bayesian optimization tools addressing multi-objective and energy-aware optimization.
MoreTranslated text
Key words
Fair Machine Learning,Green Machine Learning,Multi-objective optimization,Multiple information source Bayesian optimization
AI Read Science
Must-Reading Tree
Example
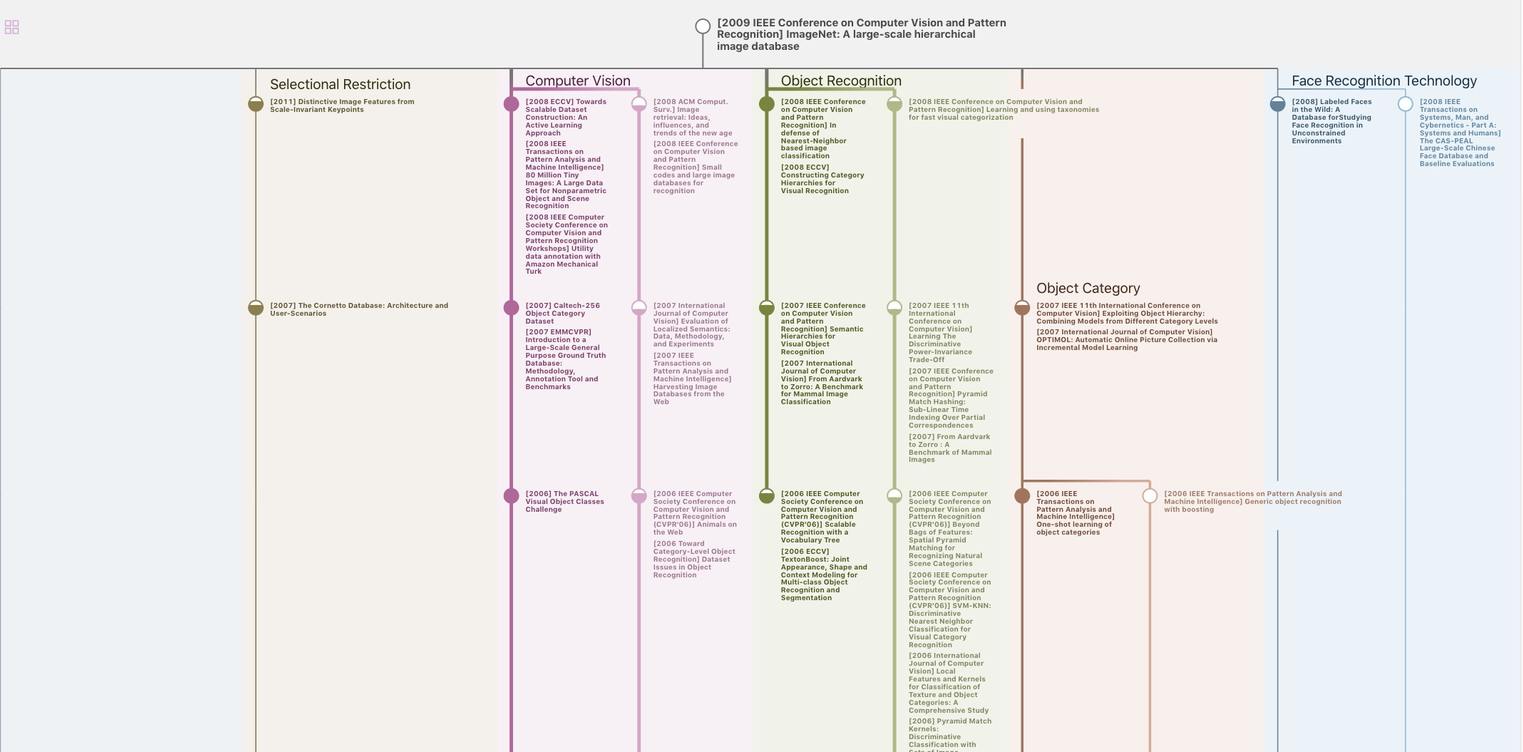
Generate MRT to find the research sequence of this paper
Chat Paper
Summary is being generated by the instructions you defined