Data Augmentation to Address Out-of-Vocabulary Problem in Low-Resource Sinhala-English Neural Machine Translation
arxiv(2022)
摘要
Out-of-Vocabulary (OOV) is a problem for Neural Machine Translation (NMT). OOV refers to words with a low occurrence in the training data, or to those that are absent from the training data. To alleviate this, word or phrase-based Data Augmentation (DA) techniques have been used. However, existing DA techniques have addressed only one of these OOV types and limit to considering either syntactic constraints or semantic constraints. We present a word and phrase replacement-based DA technique that consider both types of OOV, by augmenting (1) rare words in the existing parallel corpus, and (2) new words from a bilingual dictionary. During augmentation, we consider both syntactic and semantic properties of the words to guarantee fluency in the synthetic sentences. This technique was experimented with low resource Sinhala-English language pair. We observe with only semantic constraints in the DA, the results are comparable with the scores obtained considering syntactic constraints, and is favourable for low-resourced languages that lacks linguistic tool support. Additionally, results can be further improved by considering both syntactic and semantic constraints.
更多查看译文
关键词
translation,neural,out-of-vocabulary,low-resource,sinhala-english
AI 理解论文
溯源树
样例
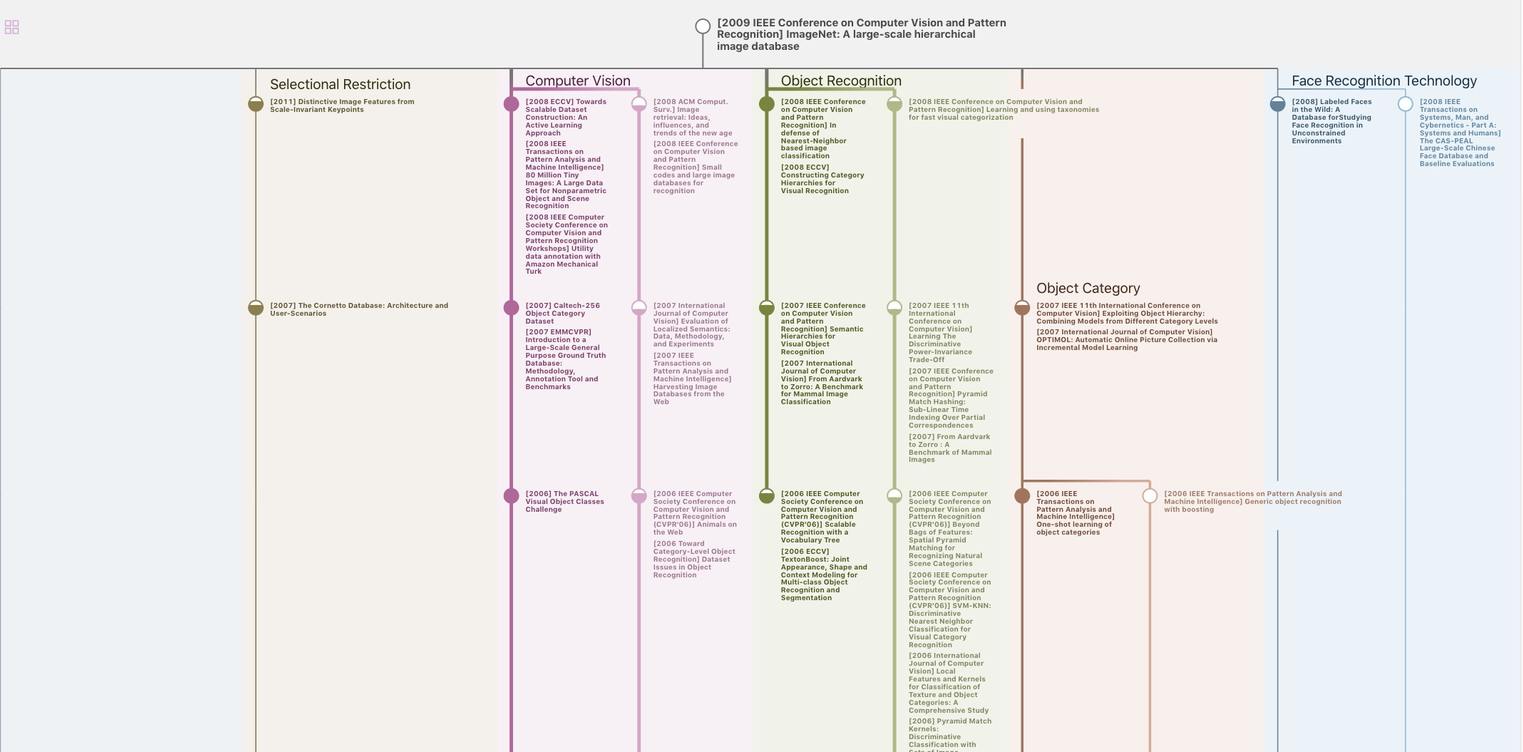
生成溯源树,研究论文发展脉络
Chat Paper
正在生成论文摘要