Addressing Resource and Privacy Constraints in Semantic Parsing Through Data Augmentation
FINDINGS OF THE ASSOCIATION FOR COMPUTATIONAL LINGUISTICS (ACL 2022)(2022)
摘要
We introduce a novel setup for low-resource task-oriented semantic parsing which incorporates several constraints that may arise in real-world scenarios: (1) lack of similar datasets/models from a related domain, (2) inability to sample useful logical forms directly from a grammar, and (3) privacy requirements for unlabeled natural utterances. Our goal is to improve a low-resource semantic parser using utterances collected through user interactions. In this highly challenging but realistic setting, we investigate data augmentation approaches involving generating a set of structured canonical utterances corresponding to logical forms, before simulating corresponding natural language and filtering the resulting pairs. We find that such approaches are effective despite our restrictive setup: in a low-resource setting on the complex SMCalFlow calendaring dataset (Andreas et al., 2020), we observe 33% relative improvement over a non-data-augmented baseline in top-1 match.
更多查看译文
关键词
semantic parsing through
AI 理解论文
溯源树
样例
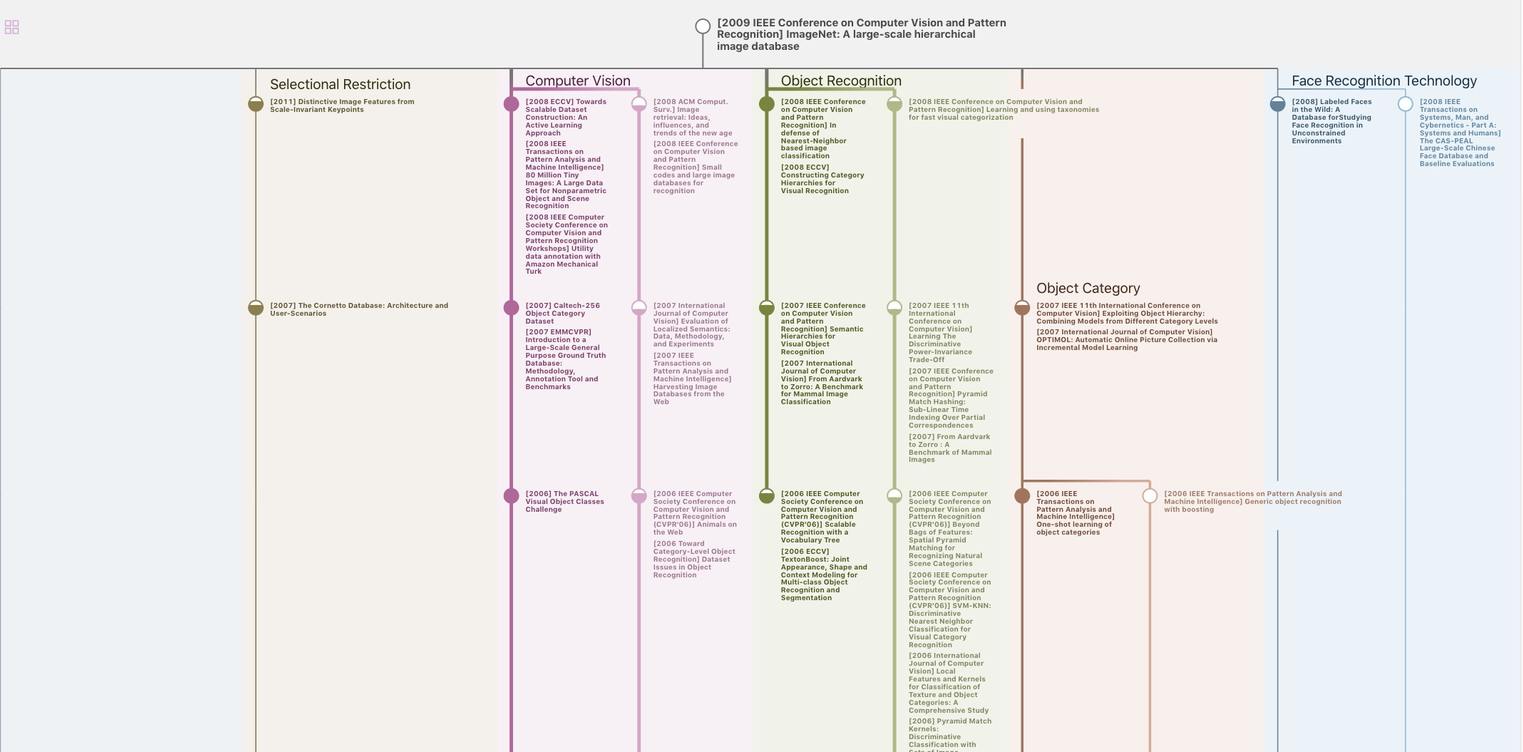
生成溯源树,研究论文发展脉络
Chat Paper
正在生成论文摘要