Learning Efficient Diverse Communication for Cooperative Heterogeneous Teaming.
International Joint Conference on Autonomous Agents and Multi-agent Systems(2022)
摘要
High-performing teams learn intelligent and efficient communication and coordination strategies to maximize their joint utility. These teams implicitly understand the different roles of heterogeneous team members and adapt their communication protocols accordingly. Multi-Agent Reinforcement Learning (MARL) seeks to develop computational methods for synthesizing such coordination strategies, but formulating models for heterogeneous teams with different state, action, and observation spaces has remained an open problem. Without properly modeling agent heterogeneity, as in prior MARL work that leverages homogeneous graph networks, communication becomes less helpful and can even deteriorate the cooperativity and team performance. We propose Heterogeneous Policy Networks (HetNet) to learn efficient and diverse communication models for coordinating cooperative heterogeneous teams. Building on heterogeneous graph-attention networks, we show that HetNet not only facilitates learning heterogeneous collaborative policies per existing agent-class but also enables end-to-end training for learning highly efficient binarized messaging. Our empirical evaluation shows that HetNet sets a new state of the art in learning coordination and communication strategies for heterogeneous multi-agent teams by achieving an 8.1% to 434.7% performance improvement over the next-best baseline across multiple domains while simultaneously achieving a 200X reduction in the required communication bandwidth.
更多查看译文
AI 理解论文
溯源树
样例
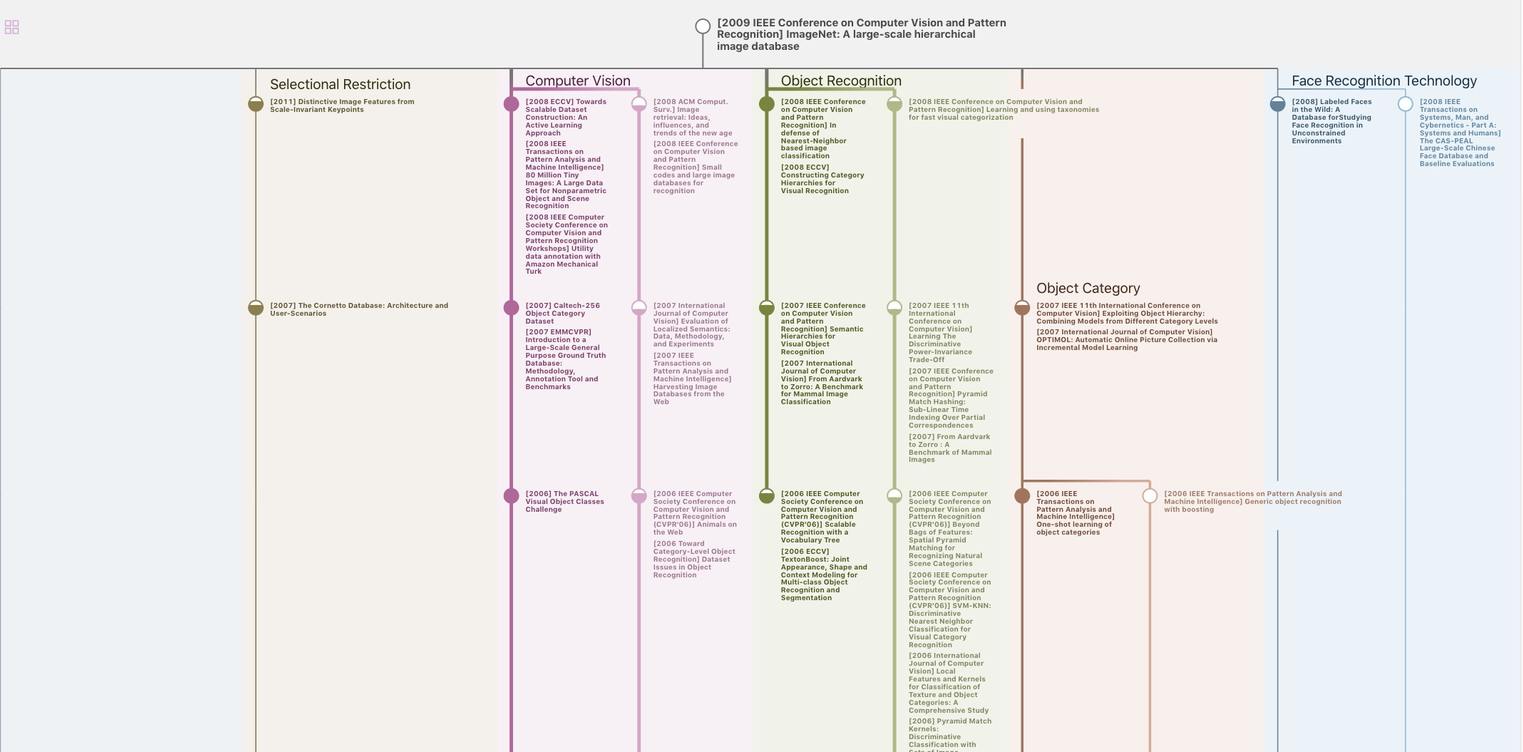
生成溯源树,研究论文发展脉络
Chat Paper
正在生成论文摘要