Reader reaction to "Outcome-adaptive lasso: Variable selection for causal inference" by Shortreed and Ertefaie (2017).
Biometrics(2023)
摘要
Shortreed and Ertefaie introduced a clever propensity score variable selection approach for estimating average causal effects, namely, the outcome adaptive lasso (OAL). OAL aims to select desirable covariates, confounders, and predictors of outcome, to build an unbiased and statistically efficient propensity score estimator. Due to its design, a potential limitation of OAL is how it handles the collinearity problem, which is often encountered in high-dimensional data. As seen in Shortreed and Ertefaie, OAL's performance degraded with increased correlation between covariates. In this note, we propose the generalized OAL (GOAL) that combines the strengths of the adaptively weighted L penalty and the elastic net to better handle the selection of correlated covariates. Two different versions of GOAL, which differ in their procedure (algorithm), are proposed. We compared OAL and GOAL in simulation scenarios that mimic those examined by Shortreed and Ertefaie. Although all approaches performed equivalently with independent covariates, we found that both GOAL versions were more performant than OAL in low and high dimensions with correlated covariates.
更多查看译文
关键词
adaptive elastic net,causal inference,high-dimensional data,propensity score,variable selection
AI 理解论文
溯源树
样例
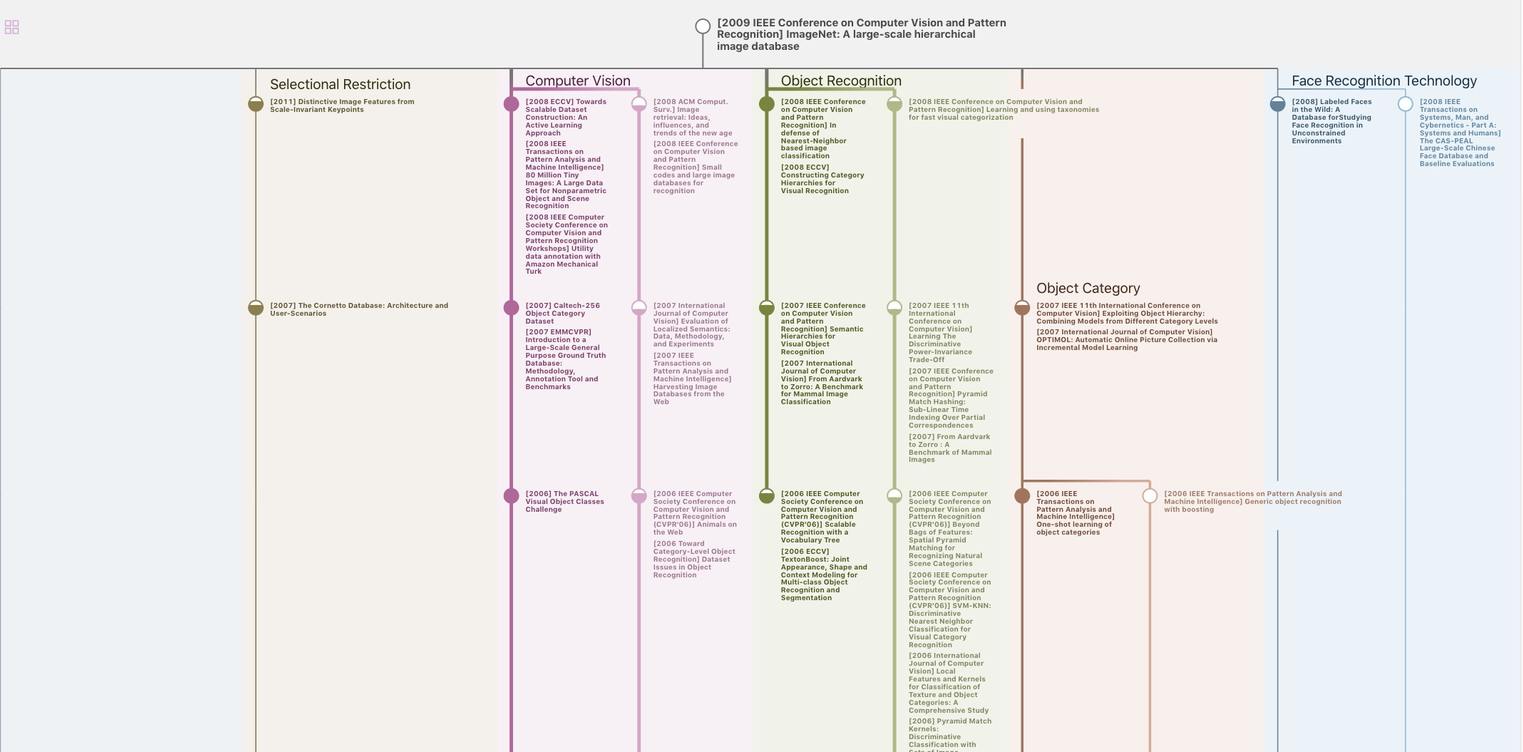
生成溯源树,研究论文发展脉络
Chat Paper
正在生成论文摘要