Mean-field nonparametric estimation of interacting particle systems.
Annual Conference on Computational Learning Theory(2022)
摘要
This paper concerns the nonparametric estimation problem of the distribution-state dependent drift vector field in an interacting $N$-particle system. Observing single-trajectory data for each particle, we derive the mean-field rate of convergence for the maximum likelihood estimator (MLE), which depends on both Gaussian complexity and Rademacher complexity of the function class. In particular, when the function class contains $\alpha$-smooth H{ö}lder functions, our rate of convergence is minimax optimal on the order of $N^{-\frac{\alpha}{d+2\alpha}}$. Combining with a Fourier analytical deconvolution estimator, we derive the consistency of MLE for the external force and interaction kernel in the McKean-Vlasov equation.
更多查看译文
关键词
estimation,mean-field
AI 理解论文
溯源树
样例
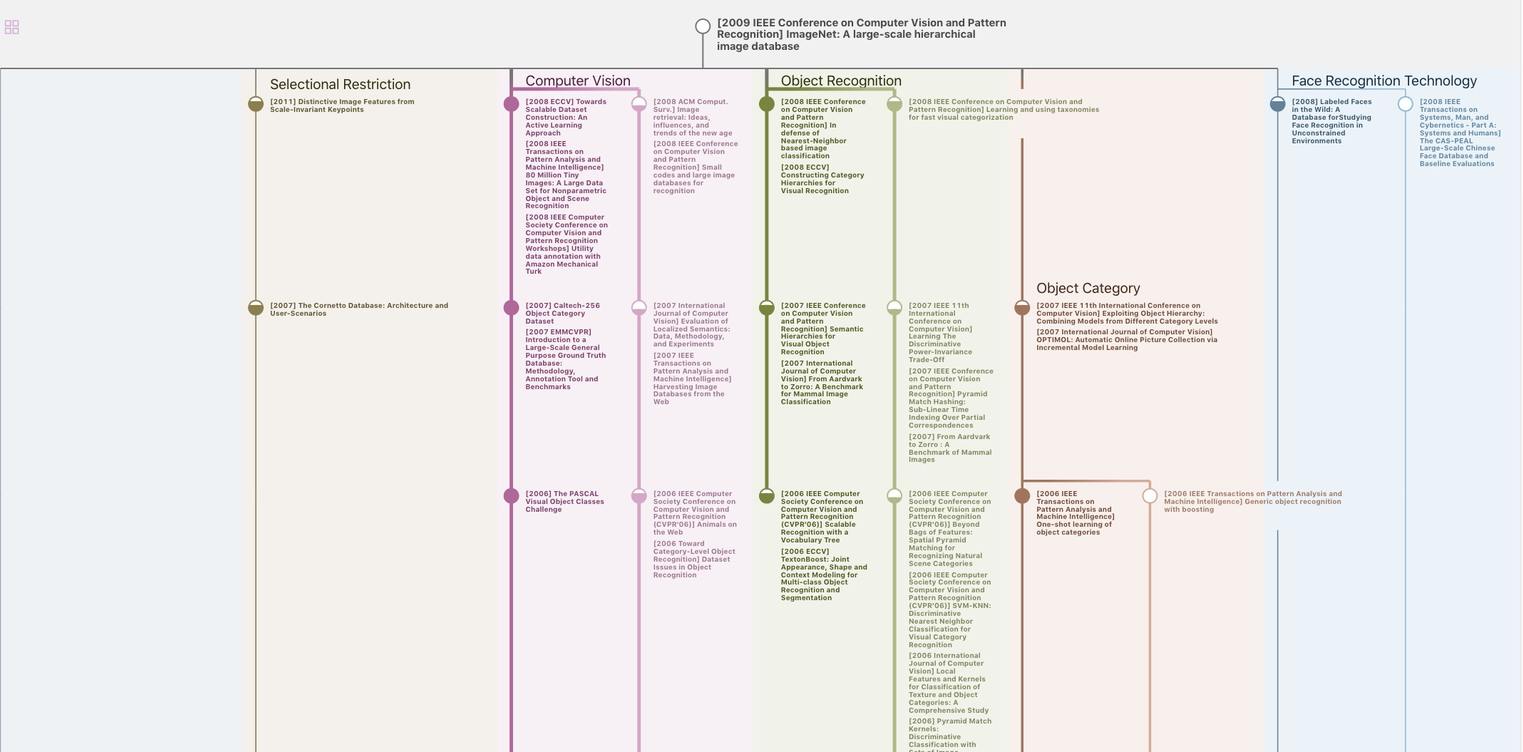
生成溯源树,研究论文发展脉络
Chat Paper
正在生成论文摘要