MixFlows: principled variational inference via mixed flows
arxiv(2023)
摘要
This work presents mixed variational flows (MixFlows), a new variational family that consists of a mixture of repeated applications of a map to an initial reference distribution. First, we provide efficient algorithms for i.i.d. sampling, density evaluation, and unbiased ELBO estimation. We then show that MixFlows have MCMC-like convergence guarantees when the flow map is ergodic and measure-preserving, and provide bounds on the accumulation of error for practical implementations where the flow map is approximated. Finally, we develop an implementation of MixFlows based on uncorrected discretized Hamiltonian dynamics combined with deterministic momentum refreshment. Simulated and real data experiments show that MixFlows can provide more reliable posterior approximations than several black-box normalizing flows, as well as samples of comparable quality to those obtained from state-of-the-art MCMC methods.
更多查看译文
关键词
variational inference,mixed mixflows
AI 理解论文
溯源树
样例
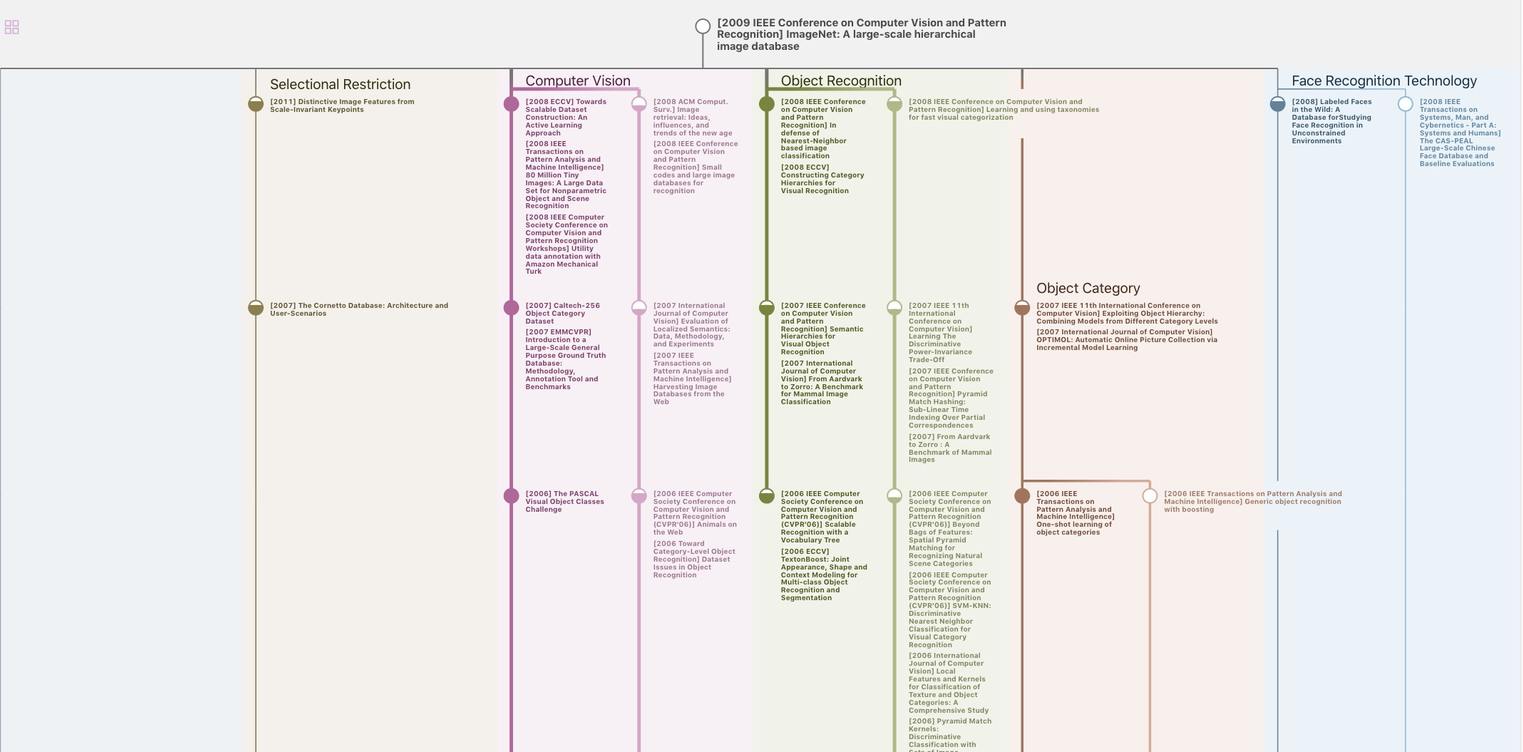
生成溯源树,研究论文发展脉络
Chat Paper
正在生成论文摘要