A Hybrid Method to Measure Distribution Consistency of Mixed-Attribute Data Sets
IEEE transactions on artificial intelligence(2022)
摘要
Random sample partition (RSP) is a newly-developed data management and processing model for big data processing and analysis. To apply the RSP model for big data computation tasks, it is very important to measure the distribution consistency of different data sets. Existing measurement methods for continuous-attribute and discrete-attribute data sets cannot directly deal with mixed-attribute data sets. In this paper, we design a hybrid method, abbreviated as MLELM-GMMD, to measure the distribution consistency among different mixed-attribute data sets by using a multi-layer extreme learning machine (MLELM) and the generalized maximum mean discrepancy (GMMD) criterion. MLELM is first used to transform original mixed-attribute data sets into corresponding deep encoding data sets. Then, the GMMD criterion is applied to check the distribution consistency of the deep encoding data sets. Four experiments have been done to validate the feasibility and effectiveness of MLELM-GMMD, i.e., the impact of MLELM on the amount of information during mixed-attribute data transformation, the impact of MLELM on distributions of mixed-attribute data, the distribution consistencies of RSP and non-RSP data blocks, and the comparison with other measurement methods. Experimental results show that the proposed MLELM-GMMD method can measure the distribution consistency of mixed-attribute data sets more accurately than one-hot encoding-based methods.
更多查看译文
关键词
Deep encoding (DE),distribution consistency,generalized maximum mean discrepancy (GMMD),mixed-attribute dataset,multilayer extreme learning machine (MLELM),one-hot encoding (OE)
AI 理解论文
溯源树
样例
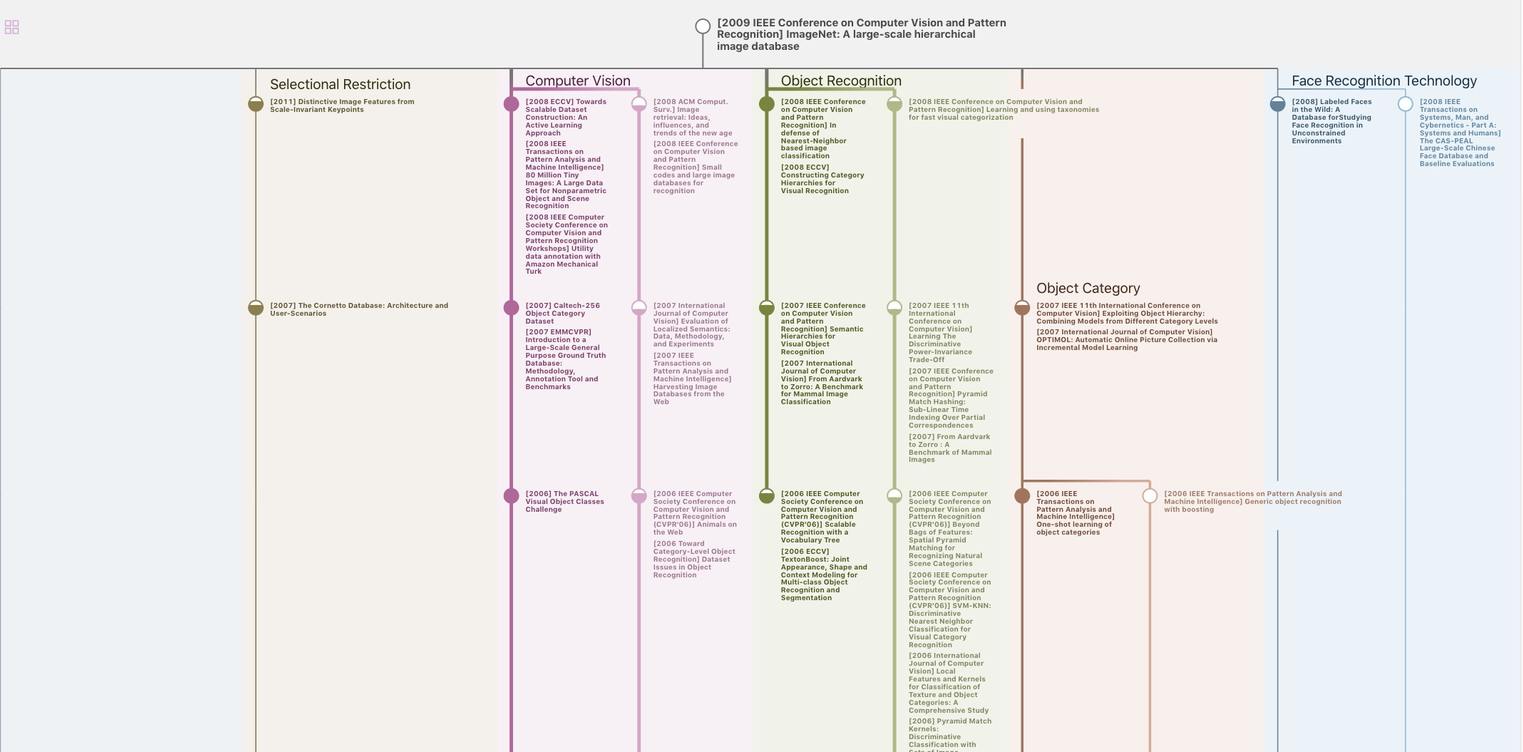
生成溯源树,研究论文发展脉络
Chat Paper
正在生成论文摘要