A Cerebellum-Inspired Network Model and Learning Approaches for Solving Kinematic Tracking Control of Redundant Manipulators
IEEE Transactions on Cognitive and Developmental Systems(2023)
摘要
Tracking control of redundant manipulators is always a basic and important issue in robotics. Existing studies have indicated that the pivotal region of the brain associated with human motion control is the cerebellum. This motivates us to devise a model-based cerebellum-inspired (MBCI) scheme and a model-free cerebellum-inspired (MFCI) scheme for the tracking control of redundant manipulators in this article. The MBCI scheme solves the inverse kinematics problem with a cerebellum model. By using the parameters and Jacobian matrix of the manipulator, the task space error is transformed into joint space error, which is taken as the teaching signal to train the cerebellum model designed based on the echo state network. The MFCI scheme is formed by coupling a cerebellum model and a multilayer perceptron (MLP). The MLP is able to generate approximate joint angle commands to the manipulator and the cerebellum model is utilized to fine-tune the MLP controller, thereby improving the tracking accuracy. In addition, leaky integrator neurons (LINs) are integrated into the cerebellum model to further improve the performance of the proposed schemes. Finally, comparative simulations and physical experiments on different types of redundant manipulators are conducted to verify the efficacy and merits of the proposed cerebellum-inspired schemes.
更多查看译文
关键词
Cerebellum inspired,model based,model free,redundant manipulator,tracking control
AI 理解论文
溯源树
样例
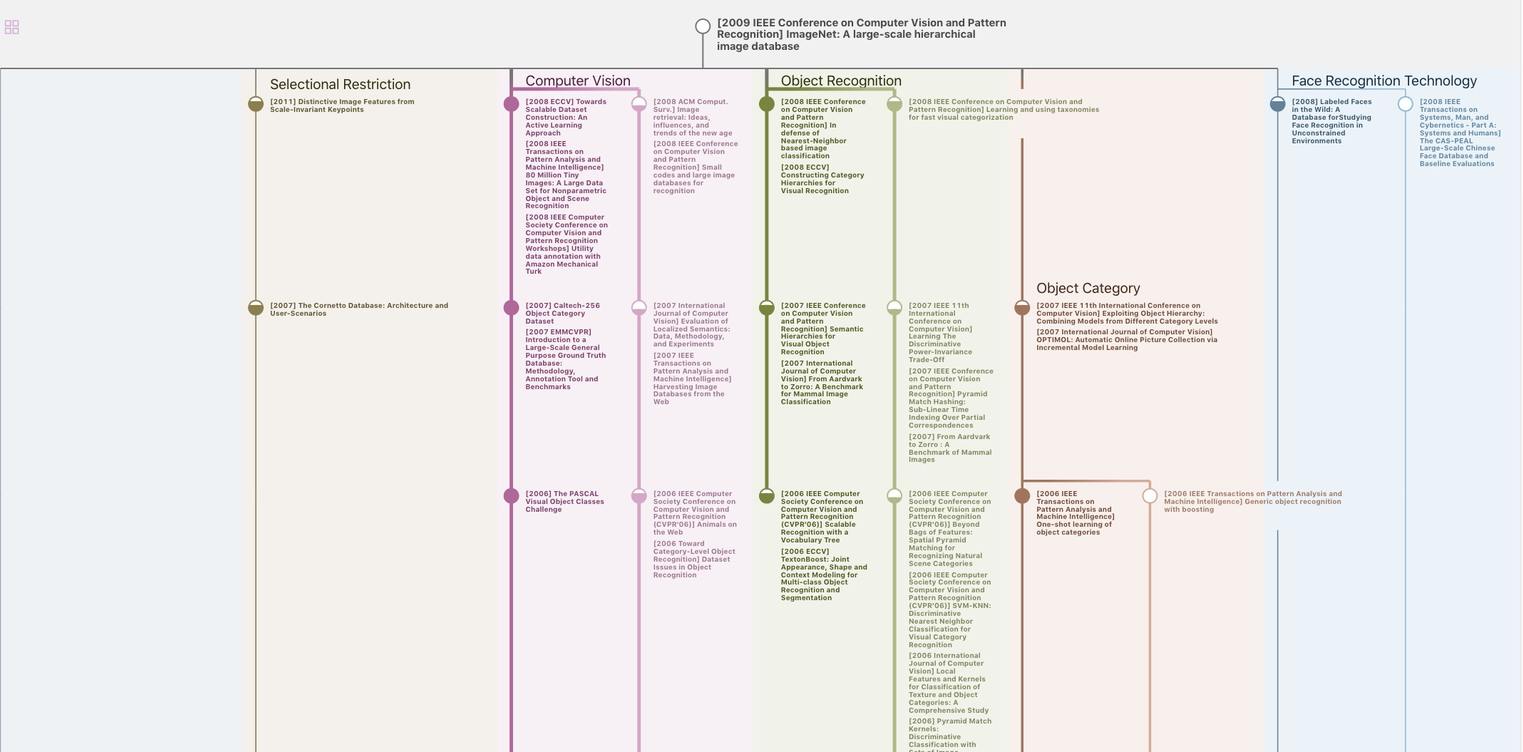
生成溯源树,研究论文发展脉络
Chat Paper
正在生成论文摘要