A supervised subtype differentiation learning for building invariant features of non-small cell lung cancer in a latent space of a Variational Autoencoder
17th International Symposium on Medical Information Processing and Analysis(2021)
摘要
This work presents a novel quantification of the cancer extension using a latent space embedded metrics of a variational autoencoder which captures the invariant patterns of the disease and projects them into a smaller latent space where data relations are linear, making it possible to apply simple metrics to quantify complicated relations. Selected patches of non-small cell lung cancer are projected to such latent space and a logistic regression model assigns an Euclidean distance between the patches projected in space. A simple grouping strategy quantitatively stratifies the characteristic patterns of the most representative patches for both adenocarcinoma and squamous cell lung cancer classes but it also estimates the composition of a mixture of patterns. This approach is fully interpretable, integrable with a pathology work flow and an objective characterization of diseases with complex patterns.
更多查看译文
关键词
Lung Cancer,Latent Space,Variational Autoencoder,Metric Learning,Digital Pathology
AI 理解论文
溯源树
样例
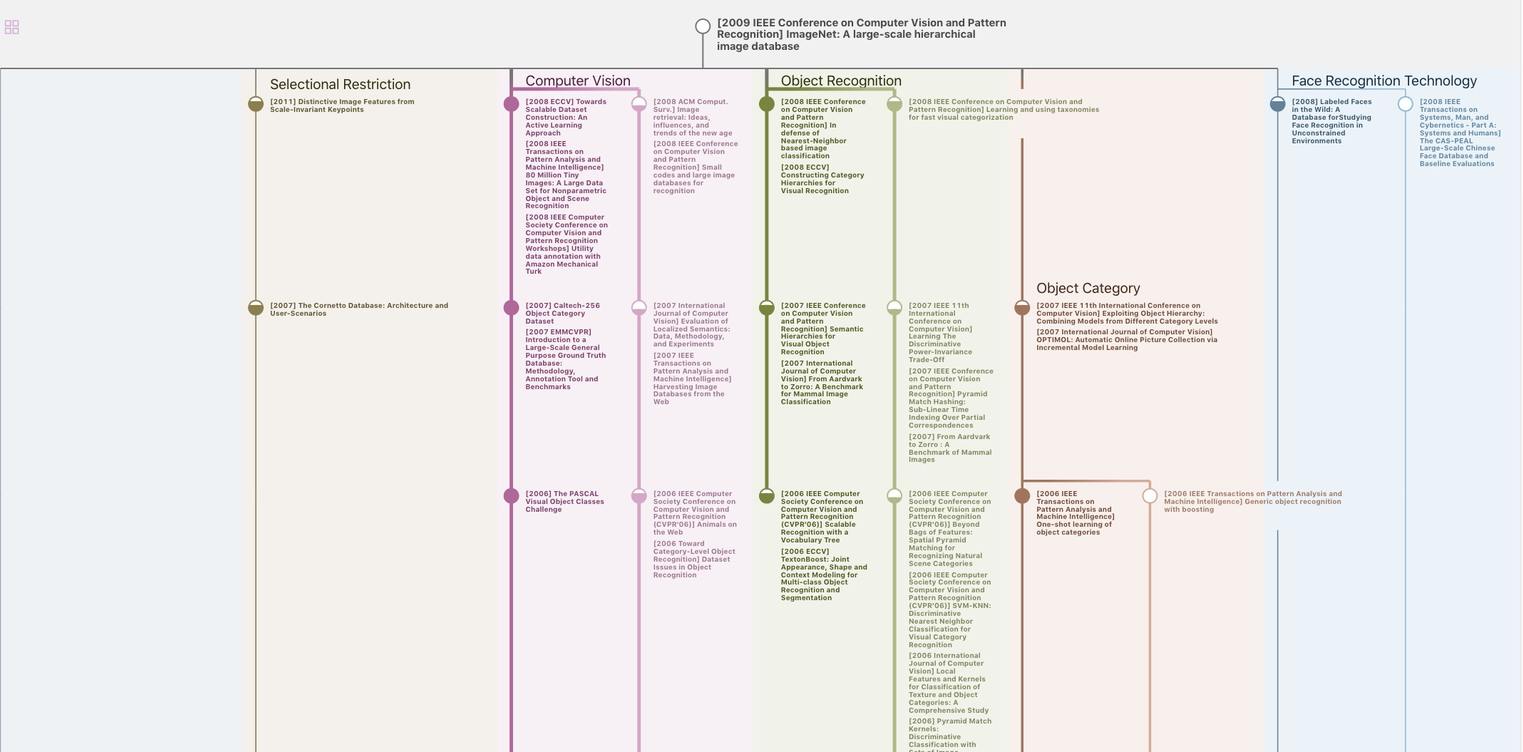
生成溯源树,研究论文发展脉络
Chat Paper
正在生成论文摘要