Prediction of milling yield in wheat with the use of spectral, colour, shape, and morphological features
BIOSYSTEMS ENGINEERING(2022)
摘要
Image and spectral data captured from 996 wheat samples were used to construct predictive models of flour milling yield (MY)%. The data consisted of multispectral images of individual wheat grains, as well as near-infrared reflectance spectra of corresponding bulk grain. Samples were characterized by sets of features extracted from the data, broadly grouped into spectral features, and image features (mean and standard deviations of sample grain features), including the colour, shape, and morphology of the grains. Image feature extraction included the use of deep learning for classification of grain orientation, estimation of the relative size of the embryo and endosperm, and a method for estimation of grain crease-depth. Extracted features were used as independent variables for the selection of regression models of MY%. The models included multiple linear regression, support vector regression, and Gaussian process regression (GPR). Along with MY%, physical grain traits related to MY%, including test weight (TW), and grain weight (GWT), were modelled to compare the performance of the feature and regression model combinations across traits. The combination of all features as input variables for a GPR model was found to give the best accuracy, with adjusted-R-2 cross-validation values of 0.81, 0.94, and 0.61, for TW, GWT, and MY, respectively. The corresponding cross-validation RMSE of the MY% model was 1.24%. Crown Copyright (C) 2021 Published by Elsevier Ltd on behalf of IAgrE. All rights reserved.
更多查看译文
关键词
Machine learning,Deep learning,Gaussian process regression,Near infrared spectroscopy,Multispectral imaging,Chemometrics
AI 理解论文
溯源树
样例
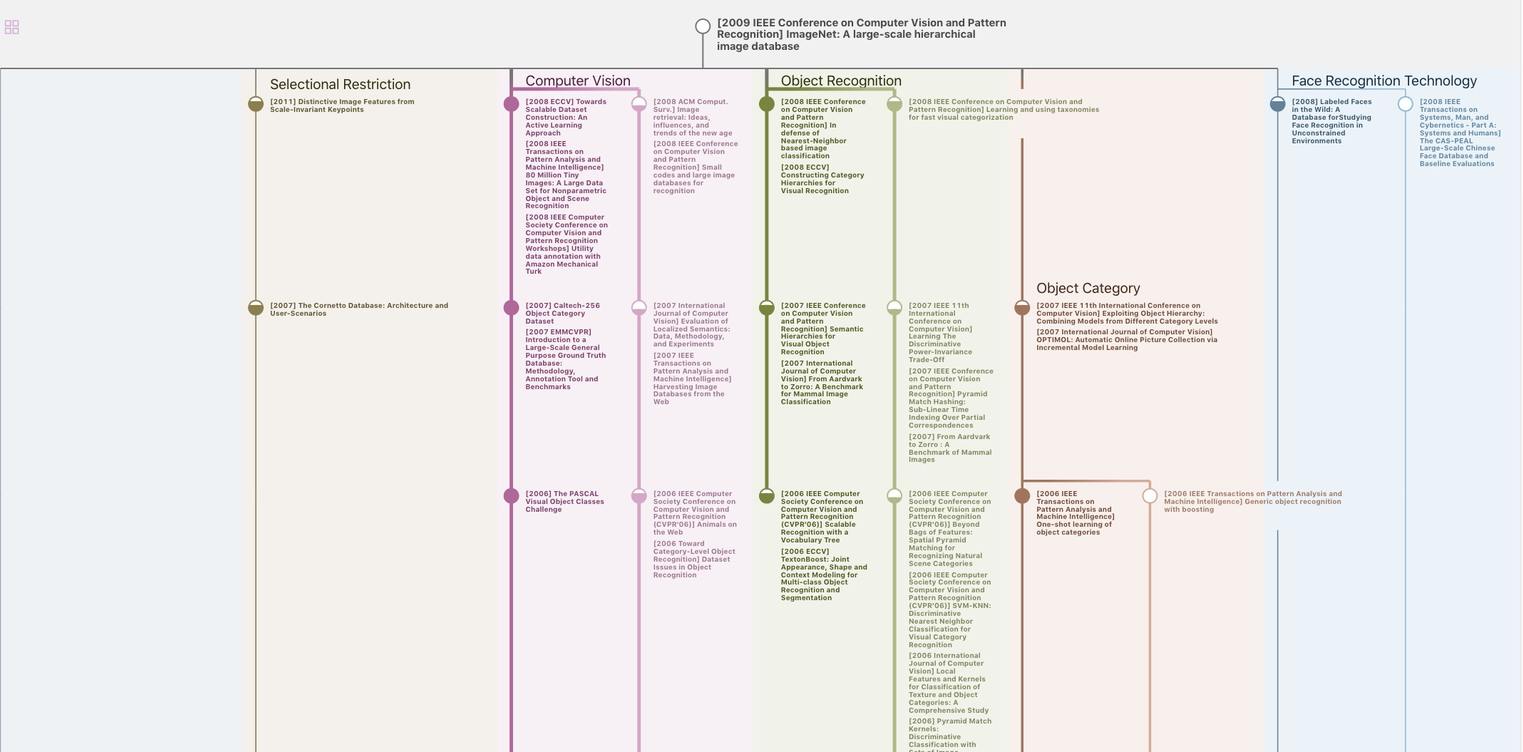
生成溯源树,研究论文发展脉络
Chat Paper
正在生成论文摘要