Perceptual metric-guided human image generation
INTEGRATED COMPUTER-AIDED ENGINEERING(2022)
摘要
Pose transfer, which synthesizes a new image of a target person in a novel pose, is valuable in several applications. Generative adversarial networks (GAN) based pose transfer is a new way for person re-identification (re-ID). Typical perceptual metrics, like Detection Score (DS) and Inception Score (IS), were employed to assess the visual quality after generation in pose transfer task. Thus, the existing GAN-based methods do not directly benefit from these metrics which are highly associated with human ratings. In this paper, a perceptual metrics guided GAN (PIGGAN) framework is proposed to intrinsically optimize generation processing for pose transfer task. Specifically, a novel and general model-Evaluator that matches well the GAN is designed. Accordingly, a new Sort Loss (SL) is constructed to optimize the perceptual quality. Morevover, PIGGAN is highly flexible and extensible and can incorporate both differentiable and indifferentiable indexes to optimize the attitude migration process. Extensive experiments show that PIGGAN can generate photo-realistic results and quantitatively outperforms state-of-the-art (SOTA) methods.
更多查看译文
关键词
Deep learning,GAN,pose transfer,attention,human pose
AI 理解论文
溯源树
样例
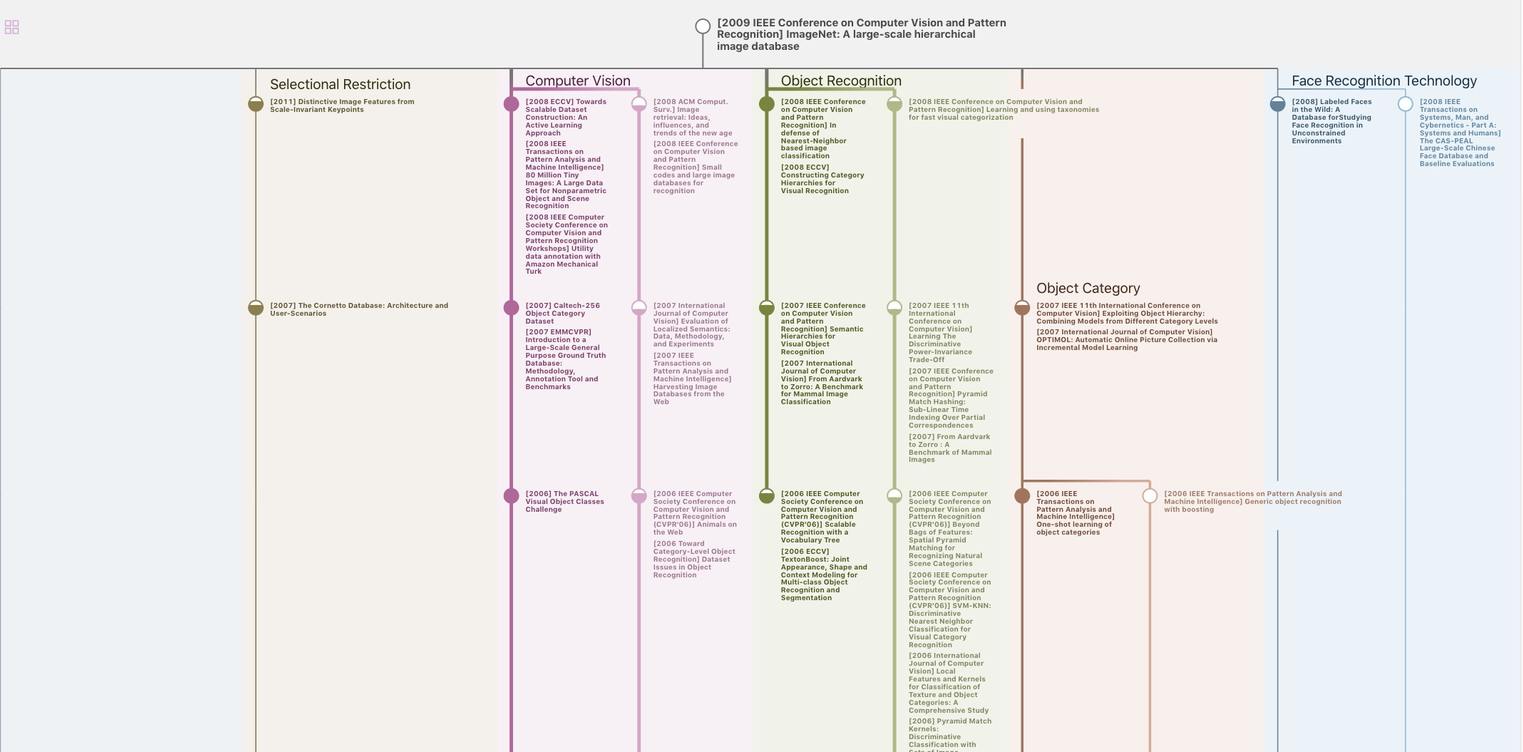
生成溯源树,研究论文发展脉络
Chat Paper
正在生成论文摘要