Continuous Adaptation with Online Meta-Learning for Non-Stationary Target Regression Tasks
Signals(2022)
摘要
Most environments change over time. Being able to adapt to such non-stationary environments is vital for real-world applications of many machine learning algorithms. In this work, we propose CORAL, a computationally efficient regression algorithm capable of adapting to a non-stationary target. CORAL is based on Bayesian linear regression with a sliding window and offline/online meta-learning. The sliding window makes our model focus on the recently received data and ignores older observations. The meta-learning approach allows us to learn the prior distribution of the model parameters. It speeds up the model adaptation, complements the sliding window’s drawback, and enhances the performance. We evaluate CORAL on two tasks: a toy problem and a more complex blood glucose level prediction task. Our approach improves the prediction accuracy for the non-stationary target significantly while also performing well for the stationary target. We show that the two components of our method work in a complementary fashion to achieve this.
更多查看译文
关键词
Bayesian meta-learning,concept drift adaptation,linear regression
AI 理解论文
溯源树
样例
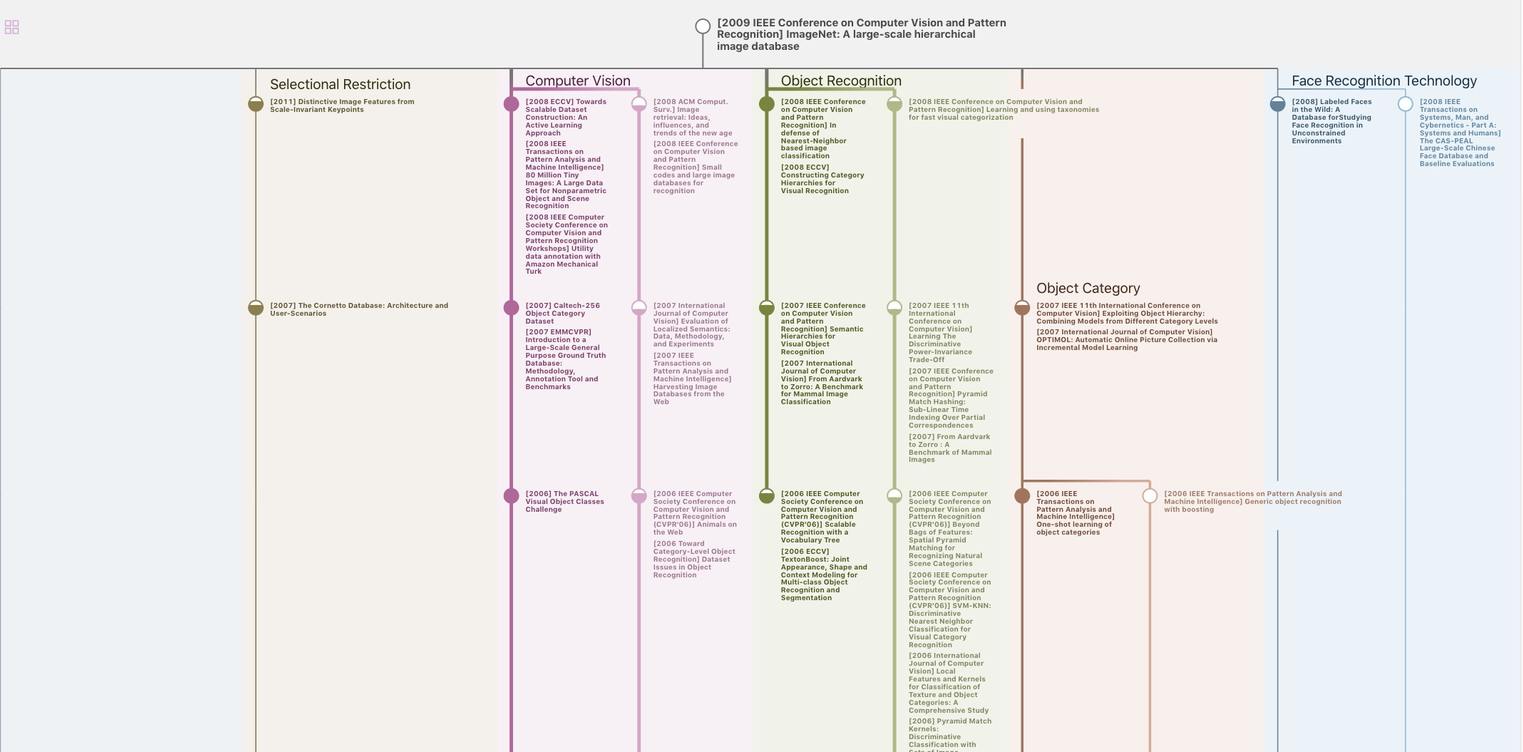
生成溯源树,研究论文发展脉络
Chat Paper
正在生成论文摘要