Random and Systematic Variation in Nanoscale Hf0.5Zr0.5O2 Ferroelectric FinFETs: Physical Origin and Neuromorphic Circuit Implications
Frontiers in nanotechnology(2022)
摘要
This work presents 2-bits/cell operation in deeply scaled ferroelectric finFETs (Fe-finFET) with a 1 µs write pulse of maximum ±5 V amplitude and WRITE endurance above 10 9 cycles. Fe-finFET devices with single and multiple fins have been fabricated on an SOI wafer using a gate first process, with gate lengths down to 70 nm and fin width 20 nm. Extrapolated retention above 10 years also ensures stable inference operation for 10 years without any need for re-training. Statistical modeling of device-to-device and cycle-to-cycle variation is performed based on measured data and applied to neural network simulations using the CIMulator software platform. Stochastic device-to-device variation is mainly compensated during online training and has virtually no impact on training accuracy. On the other hand, stochastic cycle-to-cycle threshold voltage variation up to 400 mV can be tolerated for MNIST handwritten digits recognition. A substantial inference accuracy drop with systematic retention degradation was observed in analog neural networks. However, quaternary neural networks (QNNs) and binary neural networks (BNNs) with Fe-finFETs as synaptic devices demonstrated excellent immunity toward the cumulative impact of stochastic and systematic variations.
更多查看译文
关键词
hafnium oxide,ferroelectric finFET,non-volatile memory,variation,neural networks
AI 理解论文
溯源树
样例
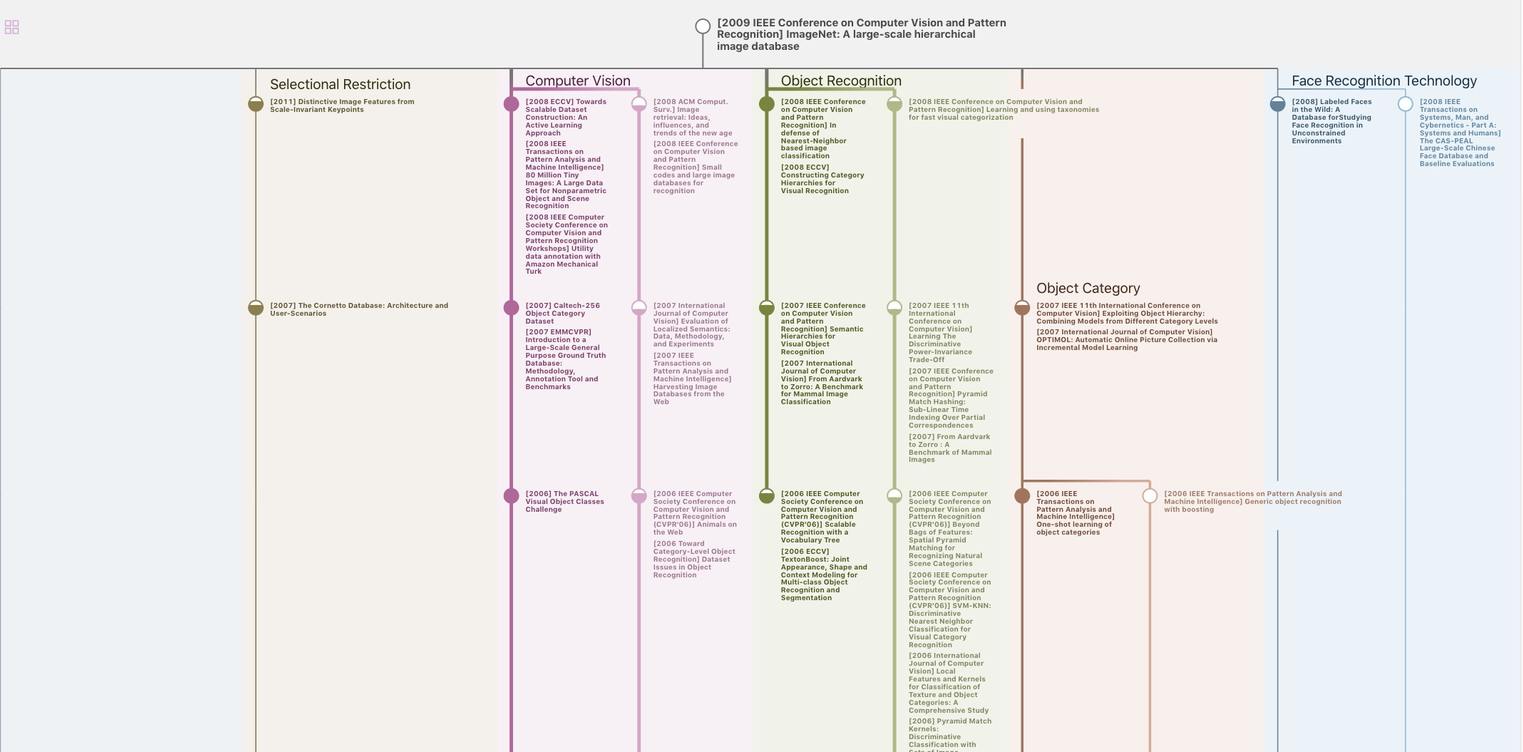
生成溯源树,研究论文发展脉络
Chat Paper
正在生成论文摘要