OUP accepted manuscript
Bioinformatics(2022)
摘要
Sequence models based on deep neural networks have achieved state-of-the-art performance on regulatory genomics prediction tasks, such as chromatin accessibility and transcription factor binding. But despite their high accuracy, their contributions to a mechanistic understanding of the biology of regulatory elements is often hindered by the complexity of the predictive model and thus poor interpretability of its decision boundaries. To address this, we introduce seqgra, a deep learning pipeline that incorporates the rule-based simulation of biological sequence data and the training and evaluation of models, whose decision boundaries mirror the rules from the simulation process.We show that seqgra can be used to (1) generate data under the assumption of a hypothesized model of genome regulation, (2) identify neural network architectures capable of recovering the rules of said model, and (3) analyze a model's predictive performance as a function of training set size and the complexity of the rules behind the simulated data.The source code of the seqgra package is hosted on GitHub (https://github.com/gifford-lab/seqgra). seqgra is a pip-installable Python package. Extensive documentation can be found at https://kkrismer.github.io/seqgra.Supplementary data are available at Bioinformatics online.
更多查看译文
关键词
genomics,neural network,prediction,principled selection
AI 理解论文
溯源树
样例
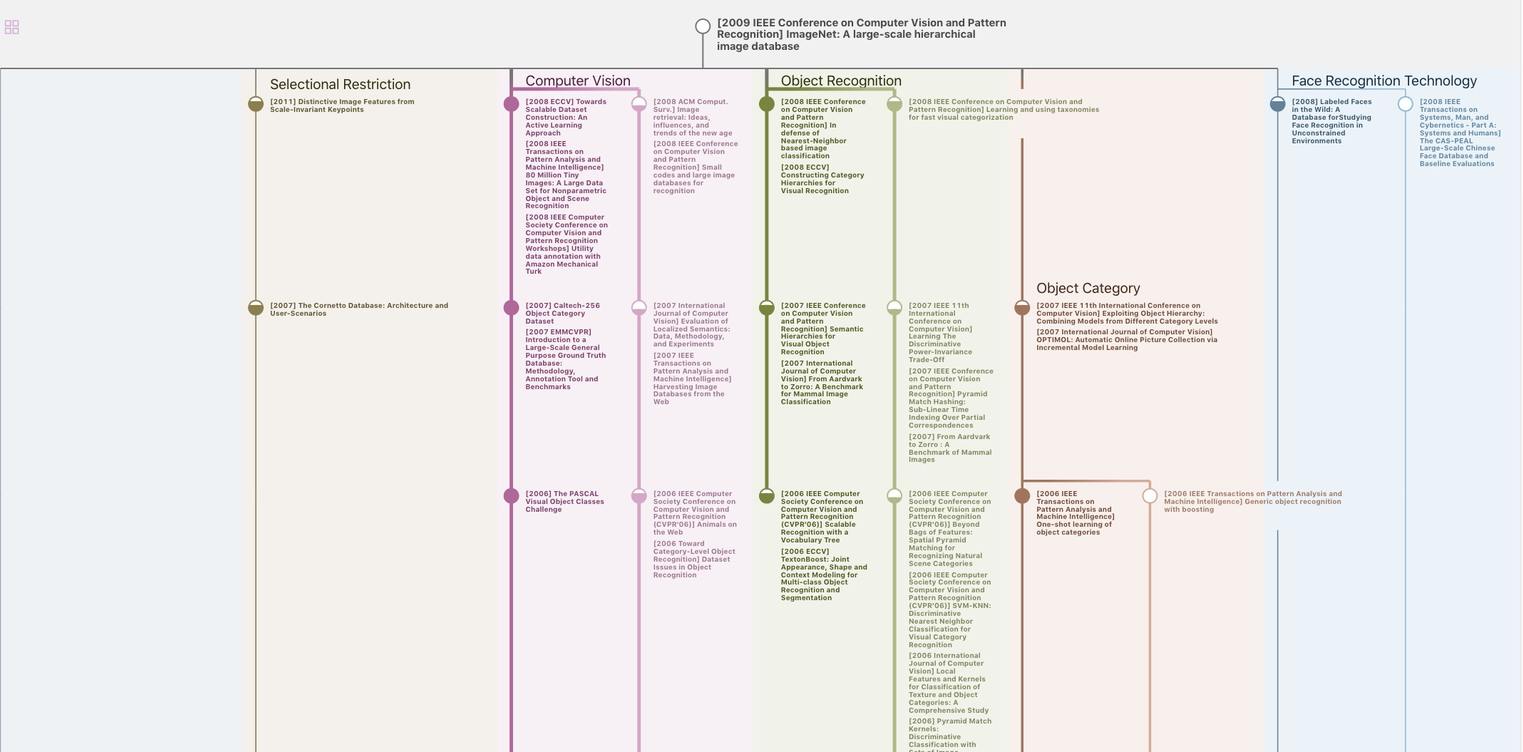
生成溯源树,研究论文发展脉络
Chat Paper
正在生成论文摘要