GANAN: GAN-based for Anomaly Detection on Attributed Networks
2021 3rd International Academic Exchange Conference on Science and Technology Innovation (IAECST)(2021)
摘要
Anomaly detection on attributed networks is crucial for many applications such as social networks security and fraud detection. Existing models utilize deep autoencoder to reconstruct structure and attribute and measure reconstruction errors to obtain anomaly nodes. However, there are problems such as over-smoothing and node’s representation learning. In order to solve these problems, in this paper, we propose a GAN-based for Anomaly Detection on Attributed Networks (GANAN). The fake graph network is generated by autoencoder. We use two encoders map both fake and real graph to latent space. The structure and attribute are used as the input of the discriminator. The game method can alleviate the over-smoothing and help encoder learn node representation. With the decoder output, the measurement considers the reconstruction error of nodes from both structure and attribute perspectives. Extensive experiments on real-world datasets demonstrate that our proposed model has better performance than various existing mothed.
更多查看译文
关键词
component,anomaly detection,attributed network,graph autoencoder,generative adversarial network,graph convolution network
AI 理解论文
溯源树
样例
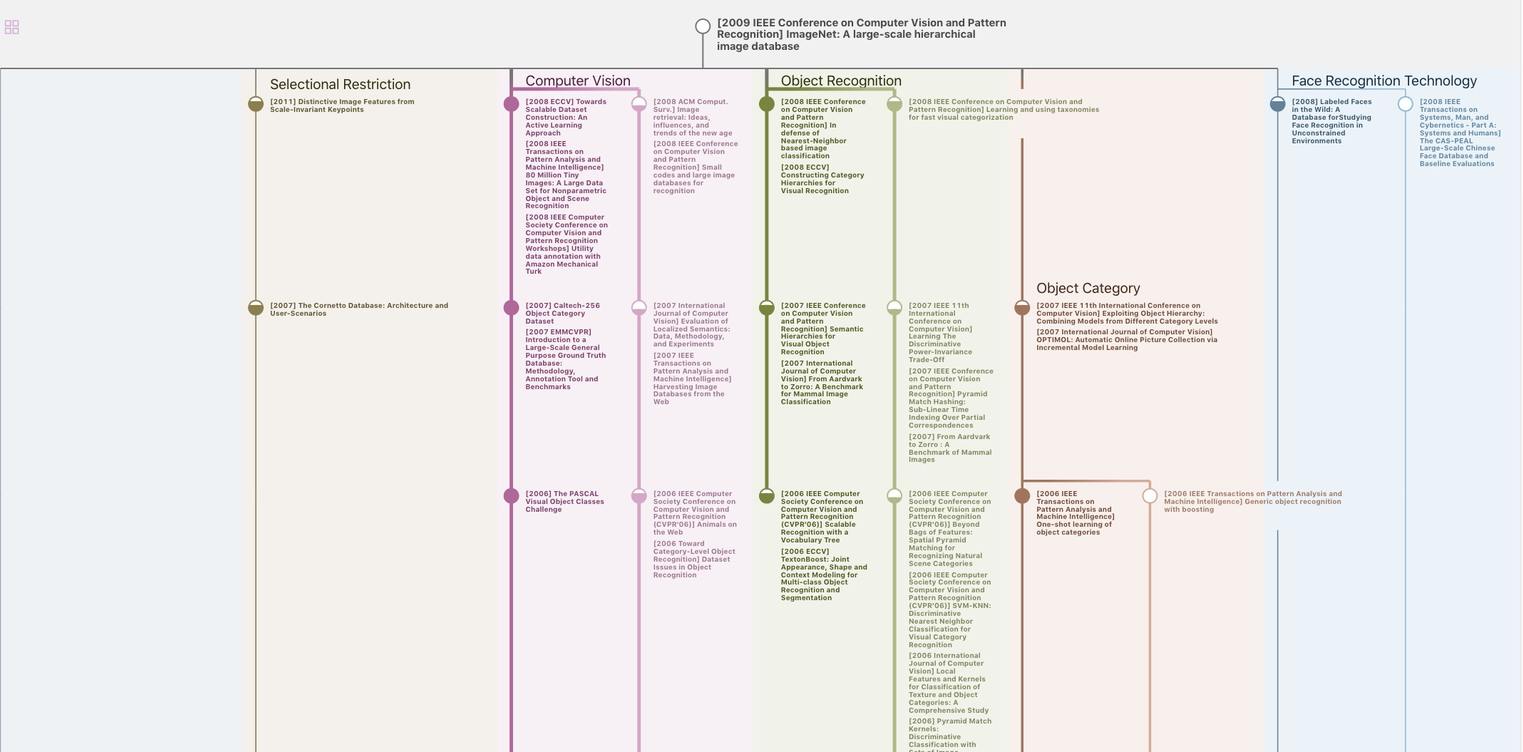
生成溯源树,研究论文发展脉络
Chat Paper
正在生成论文摘要