Semi-asynchronous personalized federated learning for short-term photovoltaic power forecasting
DIGITAL COMMUNICATIONS AND NETWORKS(2023)
Abstract
Accurate forecasting for photovoltaic power generation is one of the key enablers for the integration of solar photovoltaic systems into power grids. Existing deep-learning-based methods can perform well if there are sufficient training data and enough computational resources. However, there are challenges in building models through centralized shared data due to data privacy concerns and industry competition. Federated learning is a new distributed machine learning approach which enables training models across edge devices while data reside locally. In this paper, we propose an efficient semi-asynchronous federated learning framework for short-term solar power forecasting and evaluate the framework performance using a CNN-LSTM model. We design a personalization technique and a semi-asynchronous aggregation strategy to improve the efficiency of the proposed federated forecasting approach. Thorough evaluations using a real-world dataset demonstrate that the federated models can achieve significantly higher forecasting performance than fully local models while protecting data privacy, and the proposed semi-asynchronous aggregation and the personalization technique can make the forecasting framework more robust in real-world scenarios.
MoreTranslated text
Key words
Photovoltaic power forecasting,Federated learning,Edge computing,CNN-LSTM
AI Read Science
Must-Reading Tree
Example
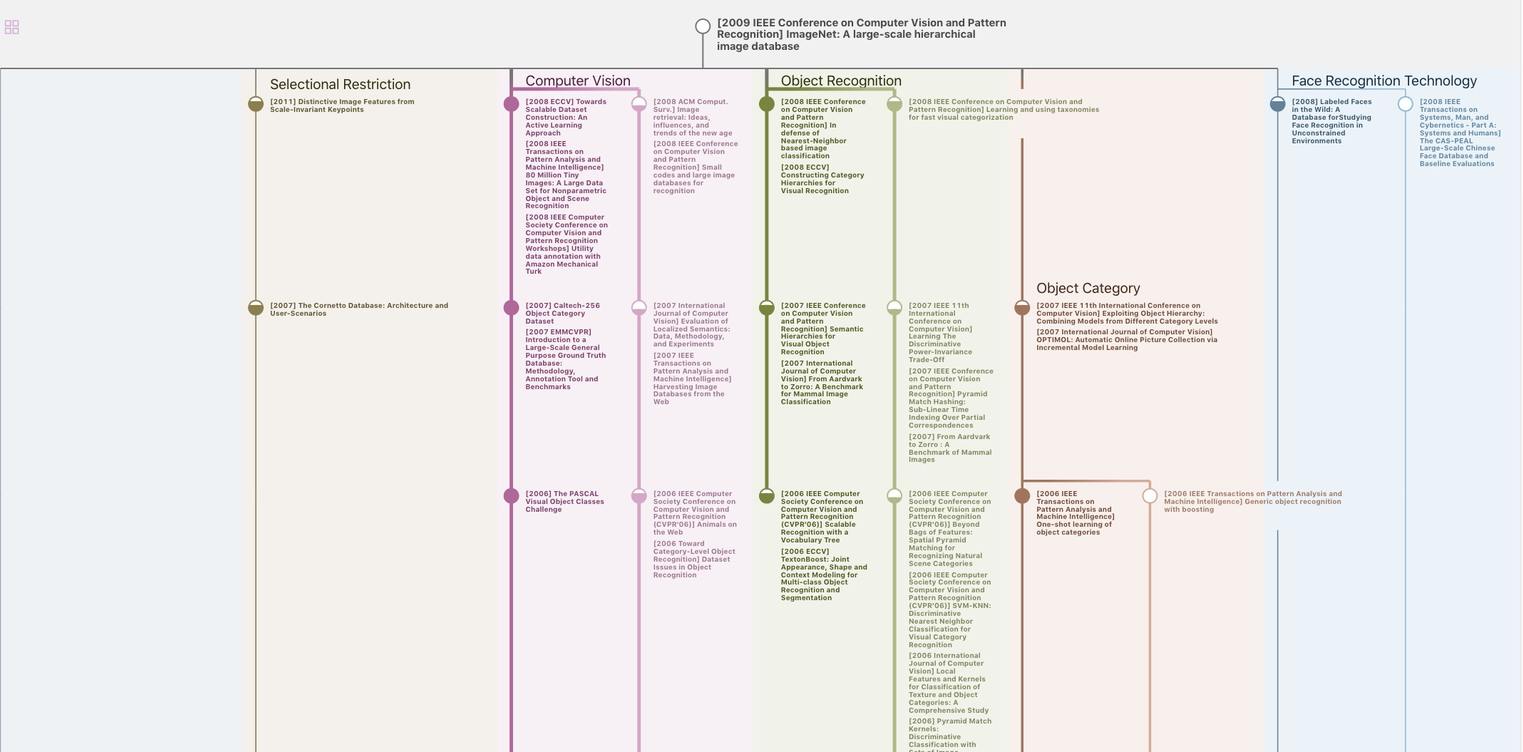
Generate MRT to find the research sequence of this paper
Chat Paper
Summary is being generated by the instructions you defined