Semi-supervised echo state network with temporal-spatial graph regularization for dynamic soft sensor modeling of industrial processes
ISA TRANSACTIONS(2022)
摘要
Echo state network (ESN) has been successfully applied to industrial soft sensor field because of its strong nonlinear and dynamic modeling capability. Nevertheless, the traditional ESN is intrinsically a supervised learning technique, which only depends on labeled samples, but omits a large number of unlabeled samples. In order to eliminate this limitation, this work proposes a semi-supervised ESN method assisted by a temporal-spatial graph regularization (TSG-SSESN) for constructing soft sensor model with all the available samples. Firstly, the traditional supervised ESN is enhanced to construct the semi-supervised ESN (SSESN) model by integrating both unlabeled and labeled samples in the reservoir computing procedure. The SSESN computes the reservoir states under high sampling rate for better process dynamic information mining. Furthermore, the SSESN's output optimization objective is modified by applying the local adjacency graph of all training samples as a regularization term. Especially, in view of the dynamic data characteristic, a temporal-spatial graph is constructed by considering both the temporal relationship and the spatial distances. The applications to a debutanizer column process and a wastewater treatment plant demonstrate that the TSG-SSESN model can build much smoother model and has better generalization capability than the basic ESN models in terms of soft sensor prediction results.(c) 2022 ISA. Published by Elsevier Ltd. All rights reserved.
更多查看译文
关键词
Dynamic process,Soft sensor,Echo state network,Temporal -structure graph,Semi -supervised model
AI 理解论文
溯源树
样例
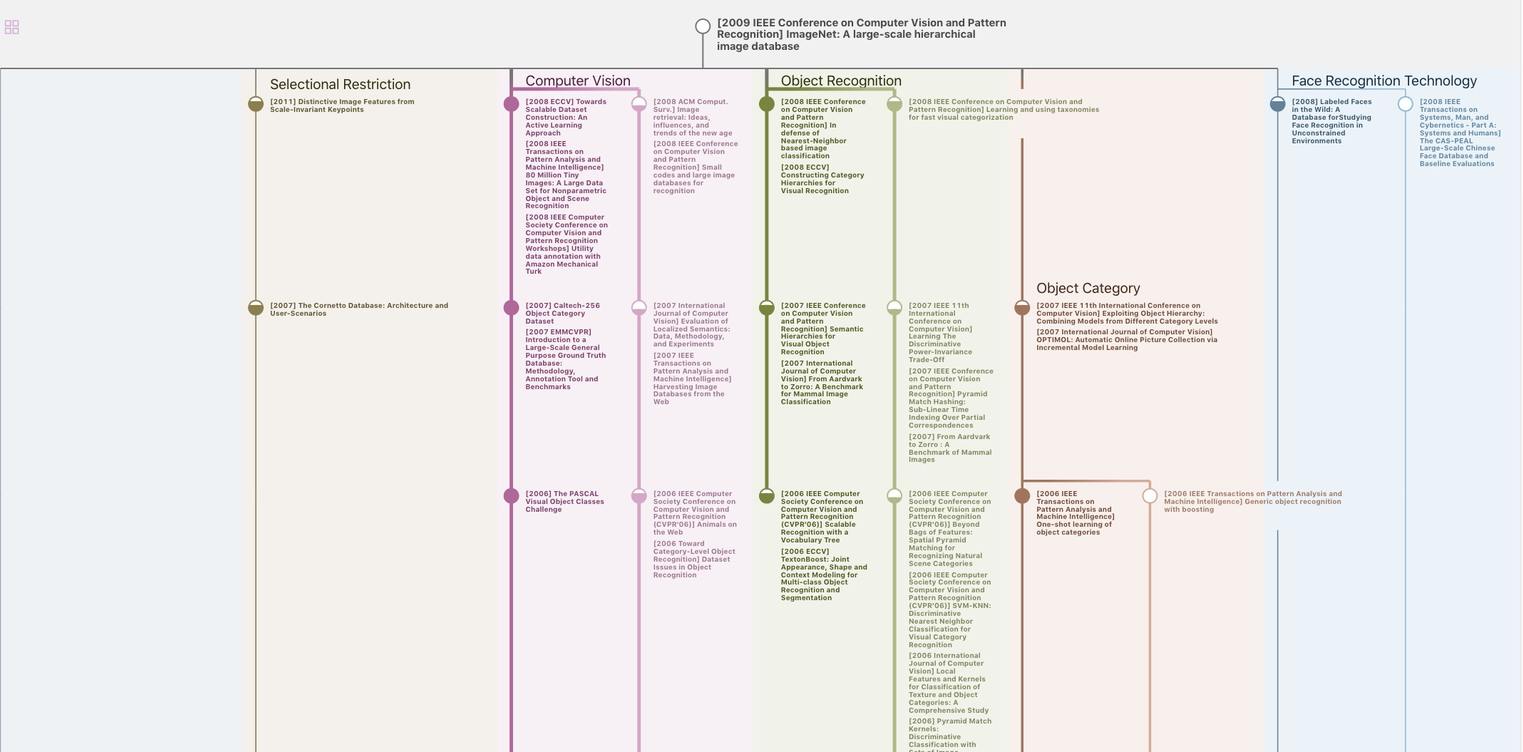
生成溯源树,研究论文发展脉络
Chat Paper
正在生成论文摘要