Temporal Features-Based Anomaly Detection from Surveillance Videos using Deep Learning Techniques
2022 Second International Conference on Artificial Intelligence and Smart Energy (ICAIS)(2022)
摘要
Automatic video surveillance is an active research area in recent times to enhance security features. Based on the crowd behavior, the normal and abnormal scenarios can be detected lively which might help someone to get support to safeguard their lives and belongings. Numerous techniques are evolved for anomaly detection from video frames however the accuracy or detection probability lags due to improperly selected features. Optimal feature selection will enhance the accuracy of the system. So, in this research work, a temporal features-based anomaly detection from surveillance video is presented using deep learning techniques. In the proposed model, the keyframes are extracted using the HSV histogram and k-means clustering technique. From the keyframes, the temporal features are extracted using a convolutional neural network and finally a concatenated Convolutional long-short term memory (ConvLSTM) model is presented to learn the sequences to recognize the activities in the video frame. Experiment results using the UCFCrime dataset demonstrate the better performance of the proposed model compared to state of art methods.
更多查看译文
AI 理解论文
溯源树
样例
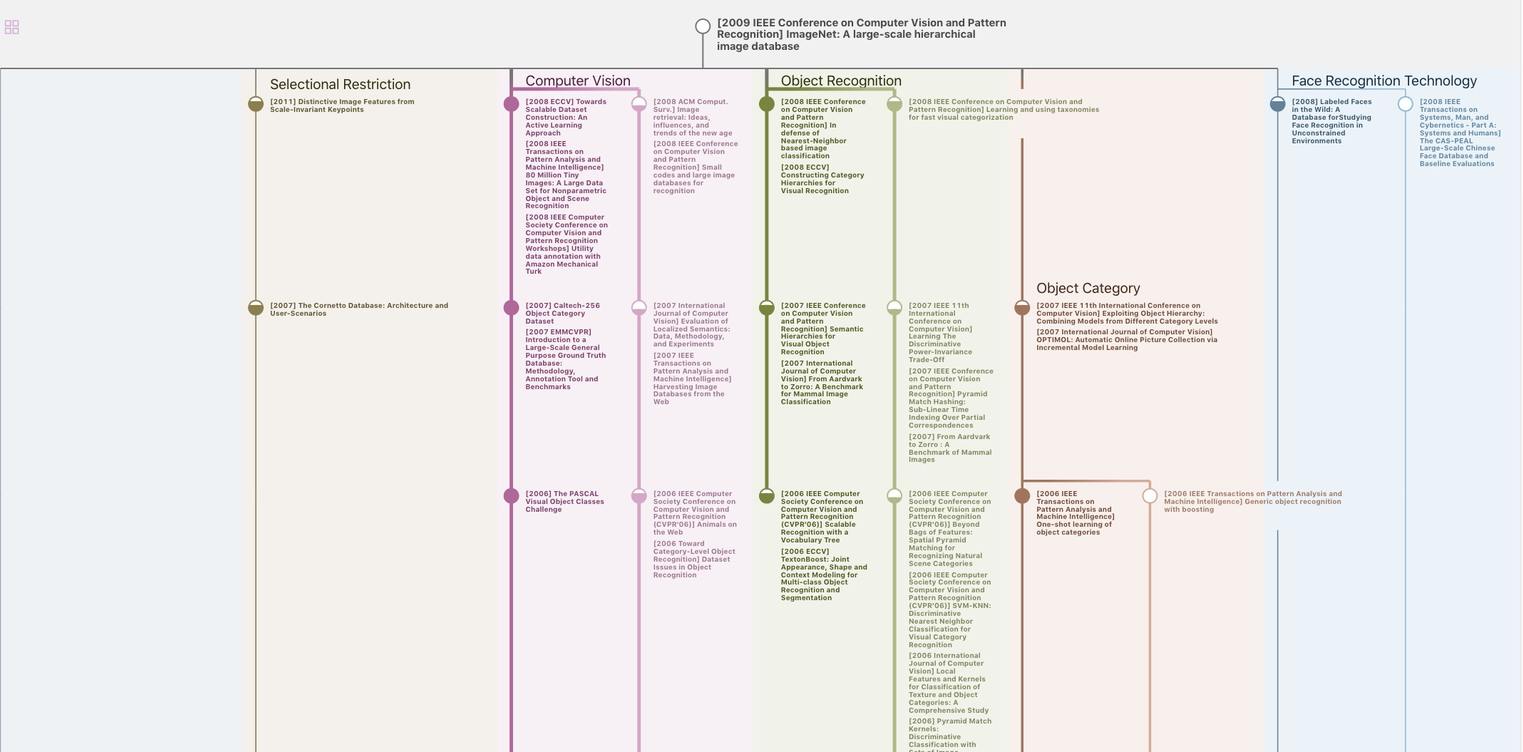
生成溯源树,研究论文发展脉络
Chat Paper
正在生成论文摘要