A novel dependency-oriented mixed-attribute data classification method
Expert Systems with Applications(2022)
摘要
How to design an efficient method to handle mixed-attribute data classification (MADC) problems has become a hot topic in data mining and machine learning. Current MADC methods mostly transform mixed-attribute data into discrete-attribute data or continuous-attribute data before classification algorithms are trained. The discretization of continuous-attribute data usually results in information loss, while the binarization of discrete-attribute data generally yield more discrete-attributes. To address these issues, this paper proposes a novel MADC method abbreviated as DO-RVFL-NBC, which is a Dependency-Oriented aggregation model of random vector functional link (RVFL) network and naive Bayes classifier (NBC). First, the method transforms the original mixed-attribute set into a dependent attribute set and an independent attribute set by considering the variation rates of dependence and independence, respectively. Second, a RVFL network is trained based on the dependent attribute set where each attribute has a weight to represent its dependence importance degree. Third, a weighted NBC is constructed by assigning the independence importance degrees as weights for the calculation of class-conditional probability. Finally, exhaustive experiments are conducted to validate the feasibility, rationality, and effectiveness of the DO-RVFL-NBC method using 22 benchmark mixed-attribute data sets. Experimental results show that (1) dependence and independence exist in the original mixed-attribute set and can be effectively explored; (2) changes of attribute dependences can improve the generalization capabilities of the RVFL network and NBC; and (3) a statistical analysis indicates that DO-RVFL-NBC can obtain considerably better testing accuracies on the benchmark mixed-attribute data sets in comparison with 13 other MADC methods. This demonstrates that DO-RVFL-NBC is a viable approach for MADC problems.
更多查看译文
关键词
Mixed-attribute data classification,Attribute independence,Random vector functional link network,Naive Bayes classifier,One-hot encoding,Attribute discretization
AI 理解论文
溯源树
样例
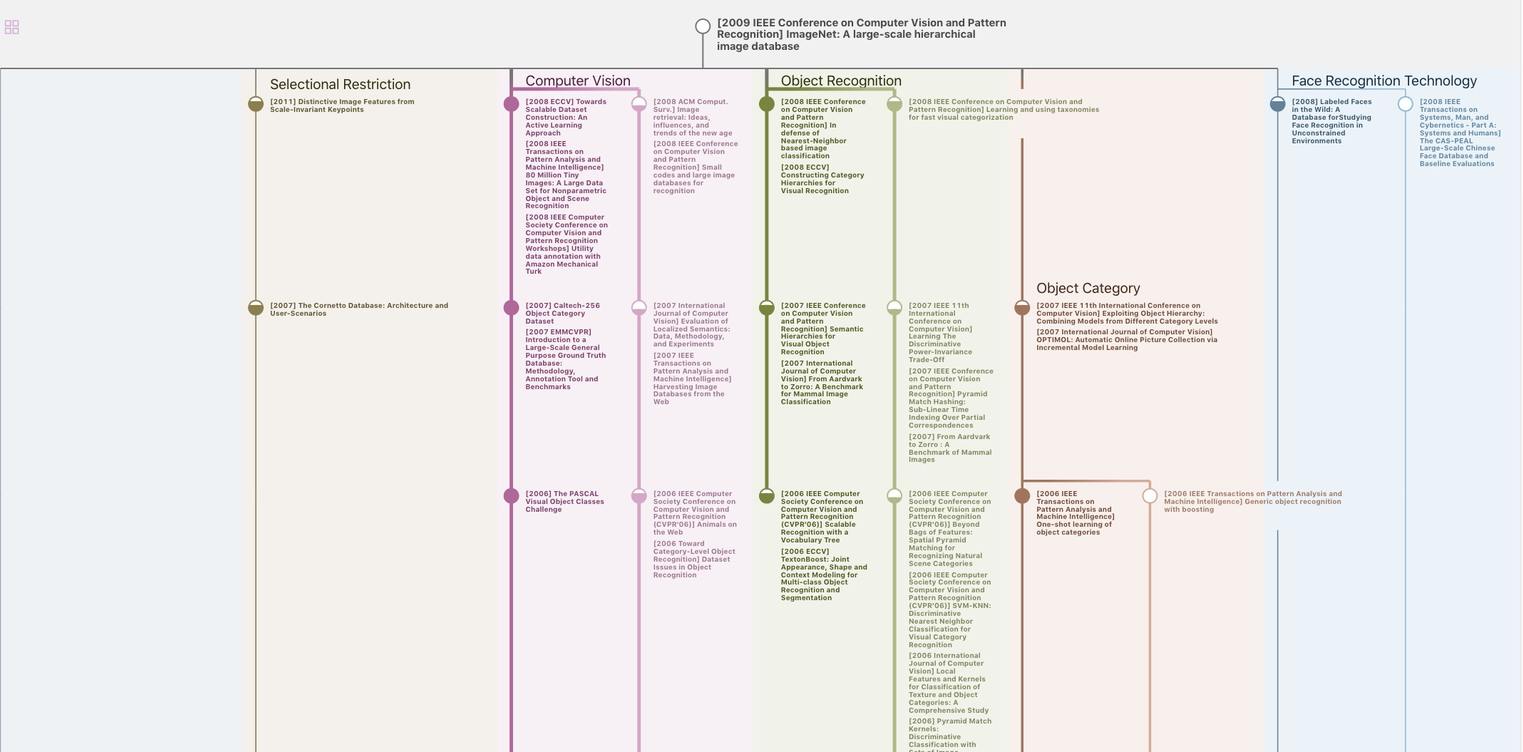
生成溯源树,研究论文发展脉络
Chat Paper
正在生成论文摘要