CMINet: an improved RGBT tracking via cross-modality interaction
International Conference on Computational Modeling, Simulation, and Data Analysis (CMSDA 2021)(2022)
摘要
Visible light and thermal infrared (RGBT) data contain different levels of information about the target, and how to use them effectively plays a crucial role in the representation of the target appearance in RGBT tracking. Existing work has focused on the integration of information from modality-shared features and modality-specific features. These approaches effectively deploy modality-shared cues and modality-specific attributes, ignoring the potential value of multi-layer shared cues of different modalities. To this end, a new multi-feature extraction-based infrared and visible target tracking algorithm is proposed. The tracking algorithm consists of a multi-layer shared fusion network, modal complementary network and target regression network that performs multi-layer modality-sharing, modality-specific and target probability prediction feature learning. Extensive experiments are conducted on the RGBT tracking benchmark dataset to achieve real-time tracking in terms of tracking speed and also show superior performance in comparison with other advanced RGB and RGBT tracking algorithms.
更多查看译文
AI 理解论文
溯源树
样例
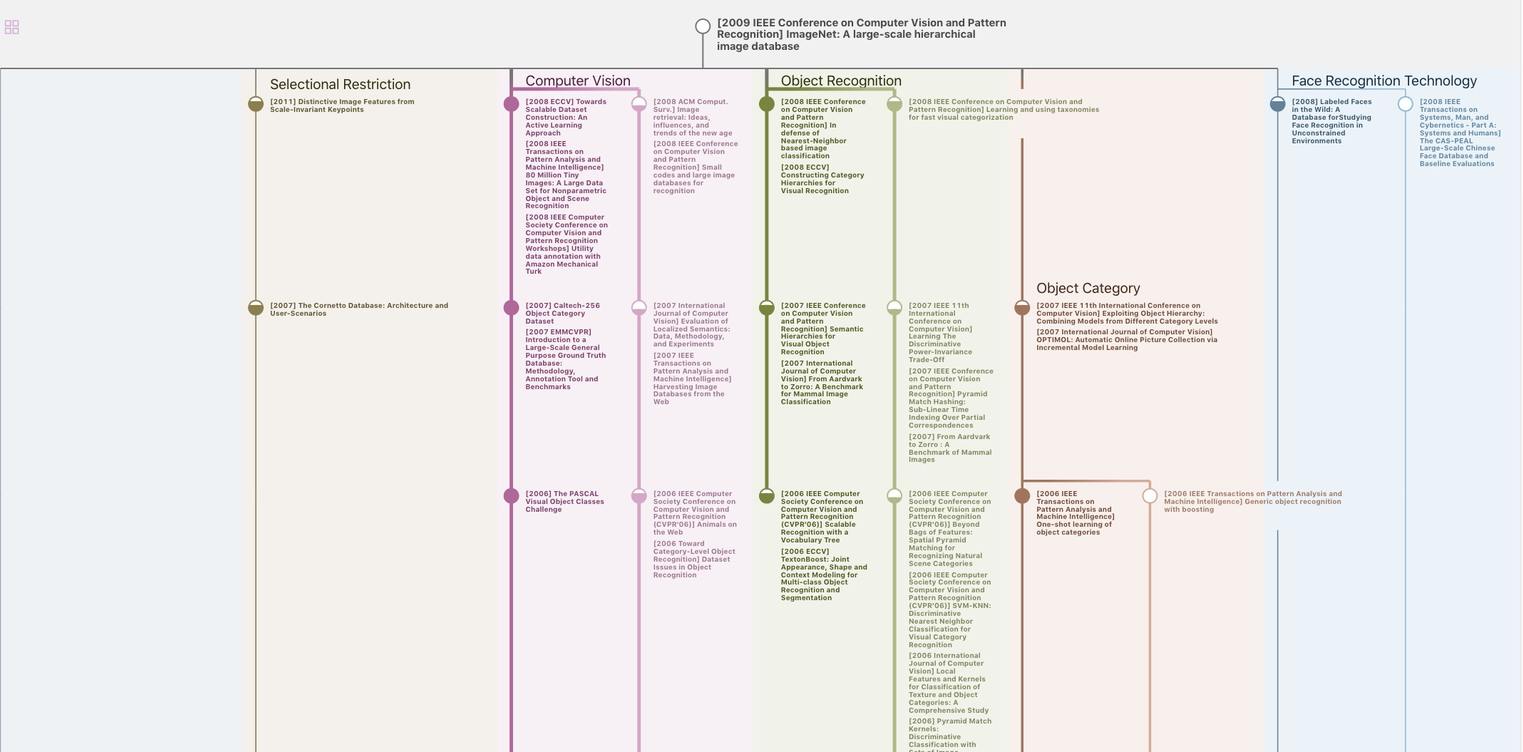
生成溯源树,研究论文发展脉络
Chat Paper
正在生成论文摘要