Single image super-resolution combined with CNN and sparse representation
2021 International Conference on Computer Information Science and Artificial Intelligence (CISAI)(2021)
摘要
Usually, the high-resolution images reconstructed by the traditional single image super-resolution algorithm often have the problems of insufficient high-frequency information and blurred edges. In order to improve the quality of reconstructed images, we propose a single image super-resolution reconstruction method based on a convolutional neural network and sparse representation. First of all, the dictionary learning method of the convolutional neural network trains the input low-resolution image to obtain LR image blocks. Then, the Learned Iterative Shrinkage and Thresholding Algorithm(LISTA) network trains LR image blocks to generate the corresponding sparse coefficients. Finally, in the reconstruction step, corresponding high-resolution image patches are obtained by Multiplying low-resolution image patches with optimal sparse coefficients. Then corresponding high-resolution image patches are combined to get the final HR image. Experimental results show that the proposed method can effectively combine the two advanced image super-resolution methods to form an efficient and flexible single image super-resolution network.
更多查看译文
关键词
super resolution,CNN,dictionary learning,sparse representation
AI 理解论文
溯源树
样例
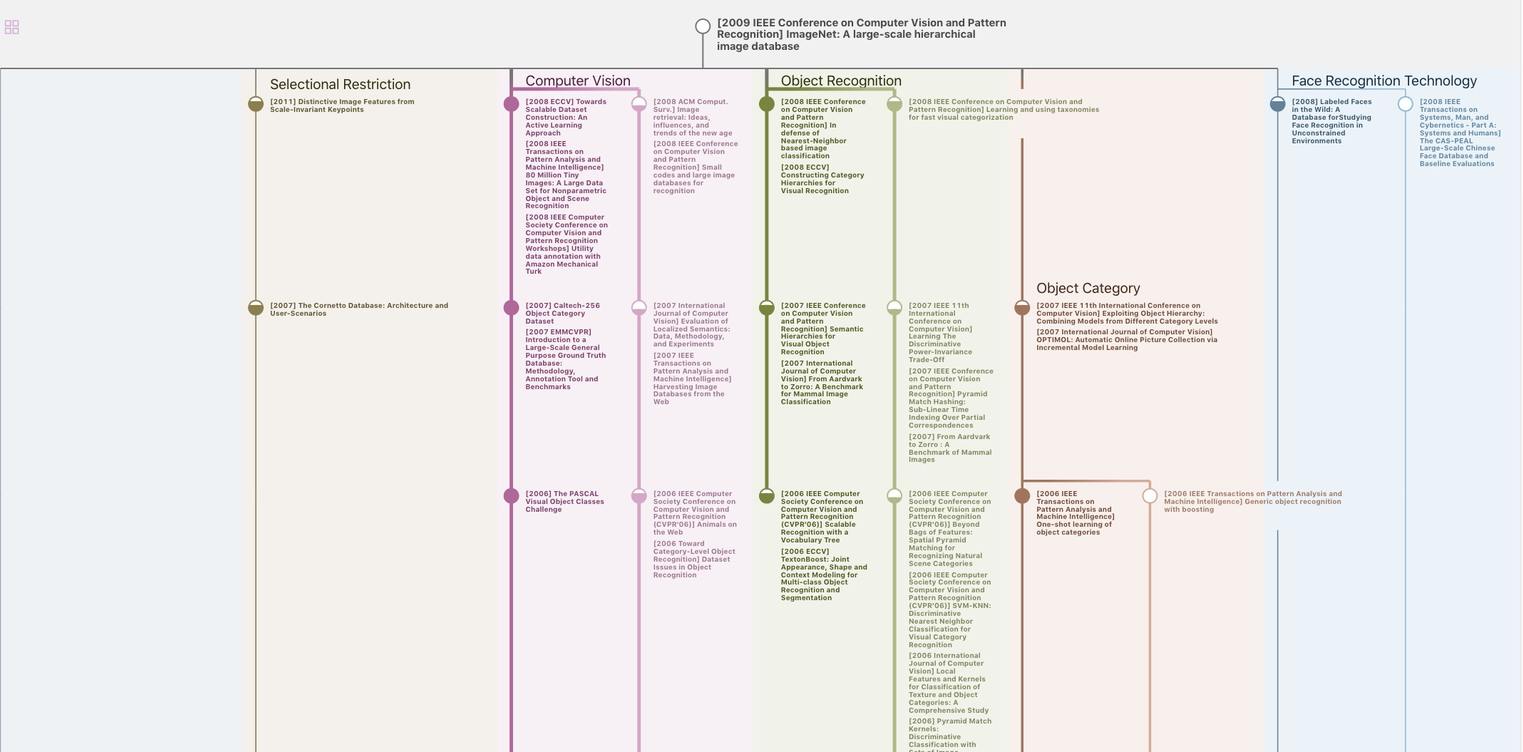
生成溯源树,研究论文发展脉络
Chat Paper
正在生成论文摘要