Outlier detection for multi-network data
BIOINFORMATICS(2022)
摘要
Motivation It has become routine in neuroscience studies to measure brain networks for different individuals using neuroimaging. These networks are typically expressed as adjacency matrices, with each cell containing a summary of connectivity between a pair of brain regions. There is an emerging statistical literature describing methods for the analysis of such multi-network data in which nodes are common across networks but the edges vary. However, there has been essentially no consideration of the important problem of outlier detection. In particular, for certain subjects, the neuroimaging data are so poor quality that the network cannot be reliably reconstructed. For such subjects, the resulting adjacency matrix may be mostly zero or exhibit a bizarre pattern not consistent with a functioning brain. These outlying networks may serve as influential points, contaminating subsequent statistical analyses. We propose a simple Outlier DetectIon for Networks (ODIN) method relying on an influence measure under a hierarchical generalized linear model for the adjacency matrices. An efficient computational algorithm is described, and ODIN is illustrated through simulations and an application to data from the UK Biobank. Results ODIN was successful in identifying moderate to extreme outliers. Removing such outliers can significantly change inferences in downstream applications. Availability and implementation ODIN has been implemented in both Python and R and these implementations along with other code are publicly available at github.com/pritamdey/ODIN-python and github.com/pritamdey/ODIN-r, respectively. Supplementary information are available at Bioinformatics online.
更多查看译文
关键词
detection,multi-network
AI 理解论文
溯源树
样例
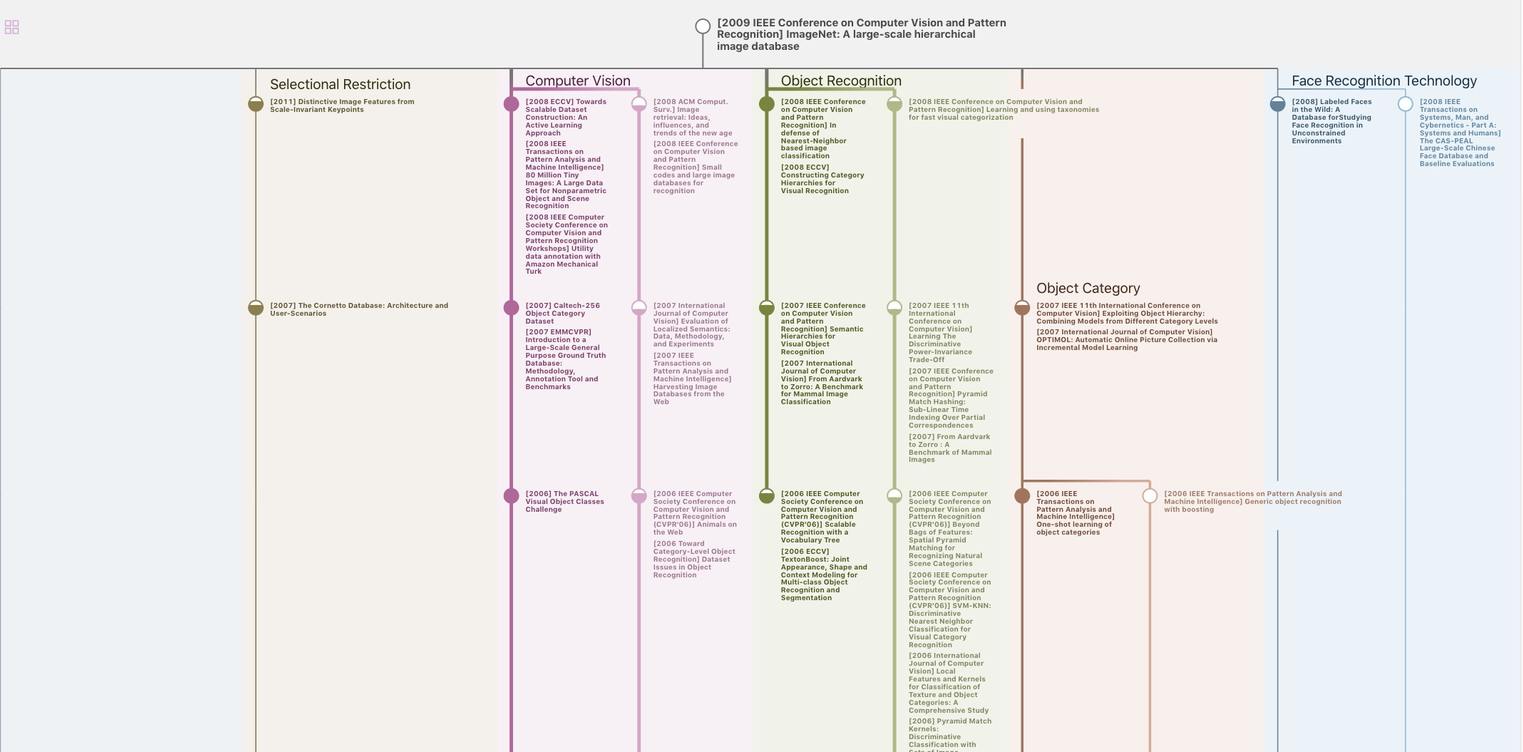
生成溯源树,研究论文发展脉络
Chat Paper
正在生成论文摘要