Joint Segmentation and Identification Feature Learning for Occlusion Face Recognition
IEEE transactions on neural networks and learning systems(2023)
摘要
The existing occlusion face recognition algorithms almost tend to pay more attention to the visible facial components. However, these models are limited because they heavily rely on existing face segmentation approaches to locate occlusions, which is extremely sensitive to the performance of mask learning. To tackle this issue, we propose a joint segmentation and identification feature learning framework for end-to-end occlusion face recognition. More particularly, unlike employing an external face segmentation model to locate the occlusion, we design an occlusion prediction module supervised by known mask labels to be aware of the mask. It shares underlying convolutional feature maps with the identification network and can be collaboratively optimized with each other. Furthermore, we propose a novel channel refinement network to cast the predicted single-channel occlusion mask into a multi-channel mask matrix with each channel owing a distinct mask map. Occlusion-free feature maps are then generated by projecting multi-channel mask probability maps onto original feature maps. Thus, it can suppress the representation of occlusion elements in both the spatial and channel dimensions under the guidance of the mask matrix. Moreover, in order to avoid misleading aggressively predicted mask maps and meanwhile actively exploit usable occlusion-robust features, we aggregate the original and occlusion-free feature maps to distill the final candidate embeddings by our proposed feature purification module. Lastly, to alleviate the scarcity of real-world occlusion face recognition datasets, we build large-scale synthetic occlusion face datasets, totaling up to 980193 face images of 10574 subjects for the training dataset and 36721 face images of 6817 subjects for the testing dataset, respectively. Extensive experimental results on the synthetic and real-world occlusion face datasets show that our approach significantly outperforms the state-of-the-art in both 1:1 face verification and 1:N face identification.
更多查看译文
关键词
Face recognition,Training,Feature extraction,Image segmentation,Representation learning,Deep learning,Learning systems,Occlusion face dataset,occlusion face recognition,occlusion segmentation,occlusion synthesis
AI 理解论文
溯源树
样例
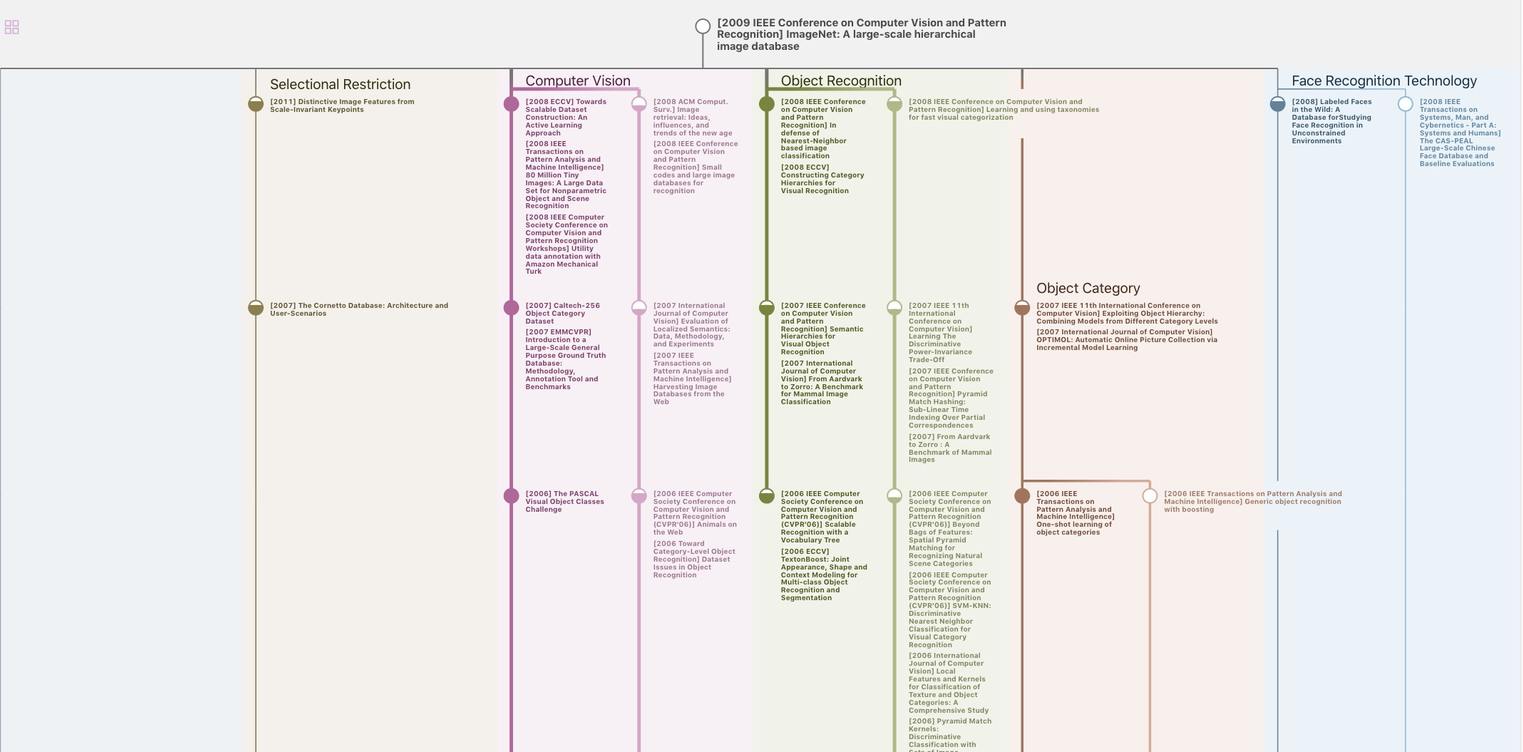
生成溯源树,研究论文发展脉络
Chat Paper
正在生成论文摘要