Deep learning of a bacterial and archaeal universal language of life enables transfer learning and illuminates microbial dark matter
NATURE COMMUNICATIONS(2022)
摘要
The majority of microbial genomes have yet to be cultured, and most proteins identified in microbial genomes or environmental sequences cannot be functionally annotated. As a result, current computational approaches to describe microbial systems rely on incomplete reference databases that cannot adequately capture the functional diversity of the microbial tree of life, limiting our ability to model high-level features of biological sequences. Here we present LookingGlass, a deep learning model encoding contextually-aware, functionally and evolutionarily relevant representations of short DNA reads, that distinguishes reads of disparate function, homology, and environmental origin. We demonstrate the ability of LookingGlass to be fine-tuned via transfer learning to perform a range of diverse tasks: to identify novel oxidoreductases, to predict enzyme optimal temperature, and to recognize the reading frames of DNA sequence fragments. LookingGlass enables functionally relevant representations of otherwise unknown and unannotated sequences, shedding light on the microbial dark matter that dominates life on Earth.
更多查看译文
关键词
Bioinformatics,Computational models,Machine learning,Metagenomics,Microbial biooceanography,Science,Humanities and Social Sciences,multidisciplinary
AI 理解论文
溯源树
样例
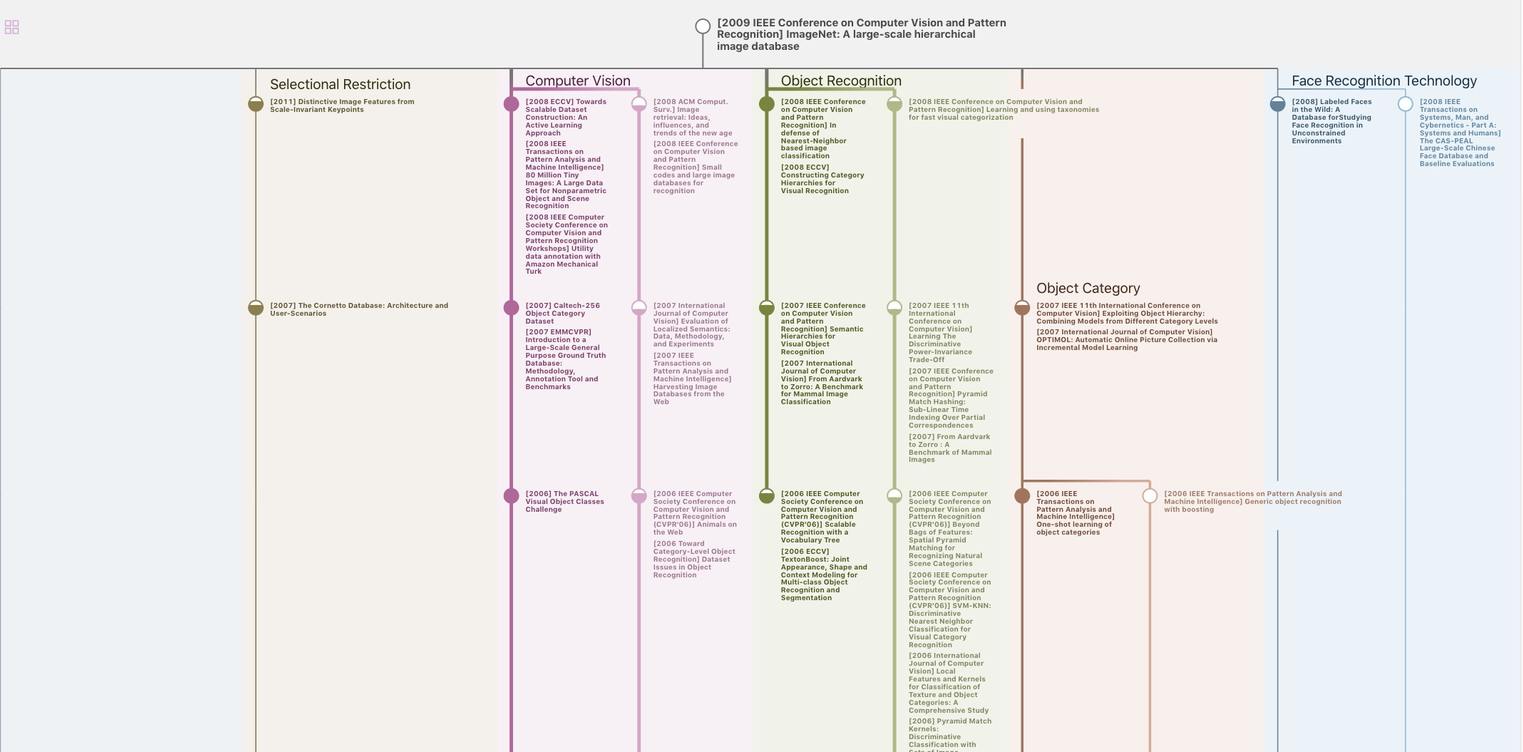
生成溯源树,研究论文发展脉络
Chat Paper
正在生成论文摘要