Modelling Within-Season Variation in Light Use Efficiency Enhances Productivity Estimates for Cropland
REMOTE SENSING(2022)
摘要
Gross Primary Productivity (GPP) for cropland is often estimated using a fixed value for maximum light use efficiency (LUEmax) which is reduced to light use efficiency (LUE) by environmental stress scalars. This may not reflect variation in LUE within a crop season, and environmental stress scalars developed for ecosystem scale modelling may not apply linearly to croplands. We predicted LUE on several vegetation indices, crop type, and agroclimatic predictors using supervised random forest regression with training data from flux towers. Using a fixed LUEmax and environmental stress scalars produced an overestimation of GPP with a root mean square error (RMSE) of 6.26 gC/m(2)/day, while using predicted LUE from random forest regression produced RMSEs of 0.099 and 0.404 gC/m(2)/day for models with and without crop type as a predictor, respectively. Prediction uncertainty was greater for the model without crop type. These results show that LUE varies between crop type, is dynamic within a crop season, and LUE models that reflect this are able to produce much more accurate estimates of GPP over cropland than using fixed LUEmax with stress scalars. Therefore, we suggest a paradigm shift from setting the LUE variable in cropland productivity models based on environmental stress to focusing more on the variation of LUE within a crop season.
更多查看译文
关键词
light use efficiency,Gross Primary Productivity,random forest
AI 理解论文
溯源树
样例
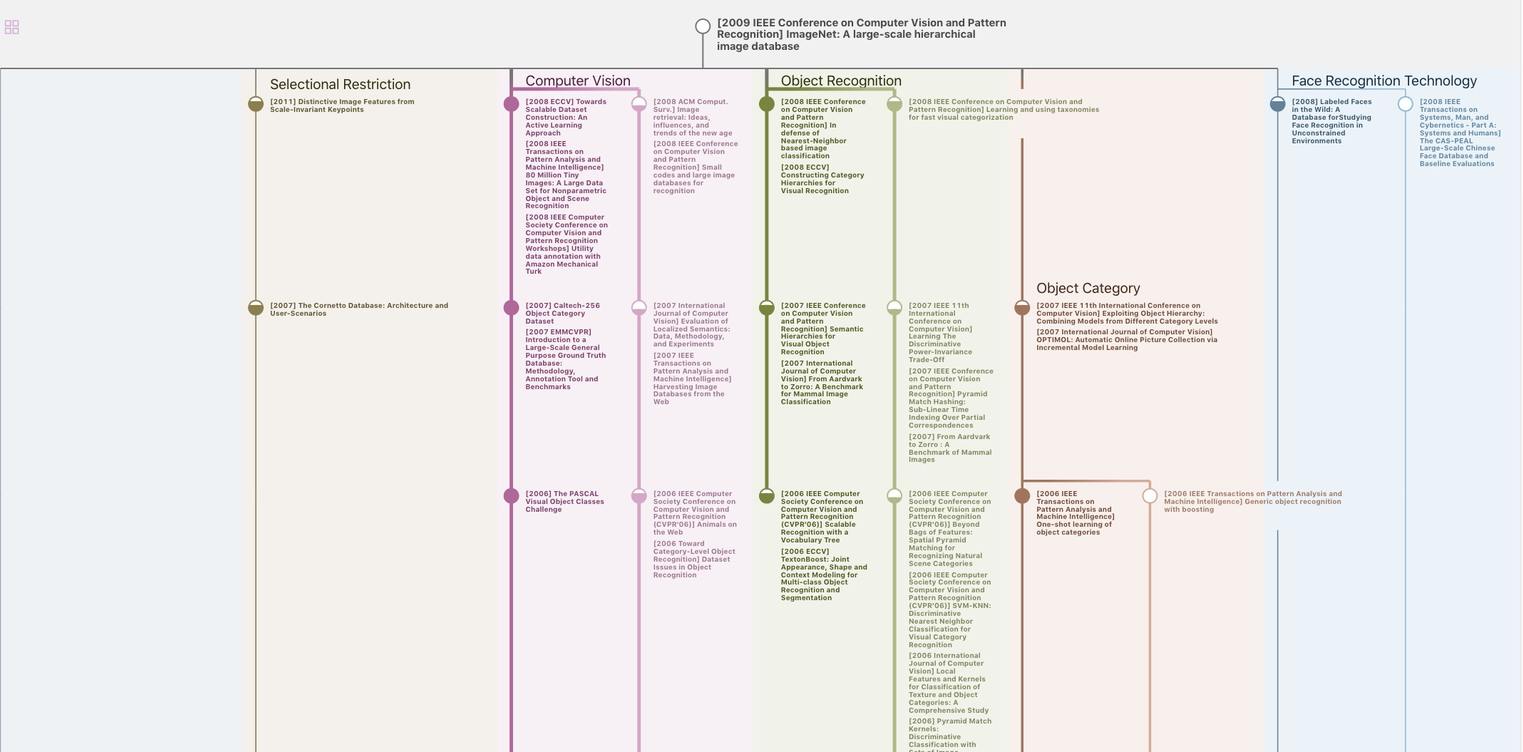
生成溯源树,研究论文发展脉络
Chat Paper
正在生成论文摘要