Trace Weighted Hessian-Aware Quantization
semanticscholar(2019)
摘要
Quantization can efficiently assist the deployment of neural networks on mobile systems with constrained resources. However, directly quantizing a model to ultra low precision could cause significant accuracy degradation. Most of the works addressing this problem use first order information, along with expensive AutoML search methods to find the bit precision for different layers. Here we introduce trace weighted Hessian-aware Quantization, a new second order based method which does not require any expensive search methods. We provide theoretical results to show that the trace of the Hessian, under certain assumption, could be used to determine sensitivity of different layers to quantization, and we use this information to perform Hessian aware fine-tuning. We test our second-order approach, and show that it exceeds industry-scale results which use expensive AutoML search methods. In particular, we present quantization results on ImageNet dataset for Inception-V3 (75.68% with 7.57MB model size) and ResNet50 (75.76% with 7.99MB model size). Both results are state-of-the-art for quantized models.
更多查看译文
AI 理解论文
溯源树
样例
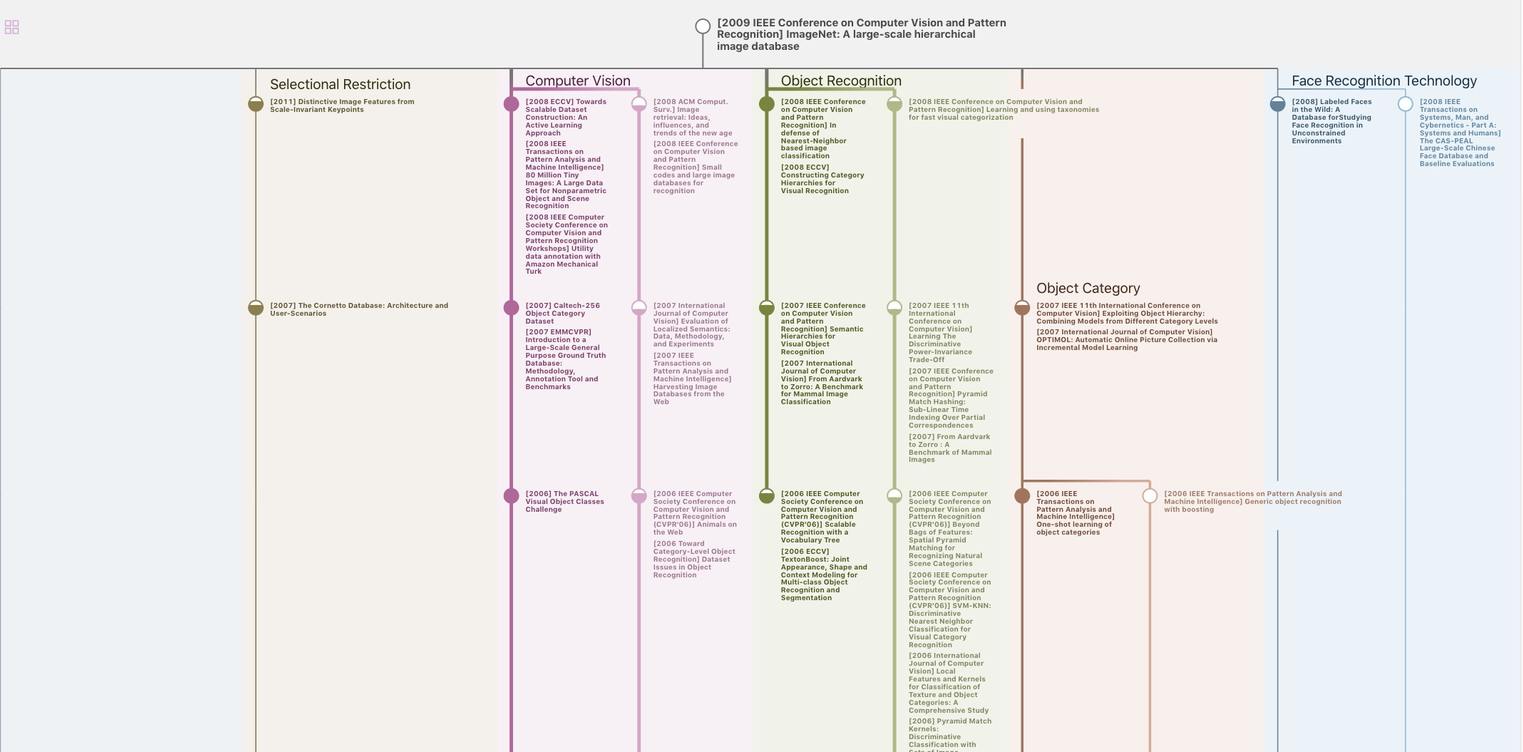
生成溯源树,研究论文发展脉络
Chat Paper
正在生成论文摘要