Analysis of EEG microstates to predict epileptic seizures in an online approach
Research on Biomedical Engineering(2022)
摘要
Purpose Epilepsy is a brain disorder characterized by recurrent unprovoked seizures. The unpredictability of seizures negatively affects the lives of patients, causing insecurity in daily activities and may cause injury or even death. Seizure prediction can prevent, through medication or safe preparation, a series of psychological, social, and physical problems that are direct consequences of this disease, such as accidents and mental disorders. This work aims to present a proposal to generalized seizure prediction online using microstate analysis, a method that has been little explored in epilepsy studies but has great potential to generate good results. Methods This study presents a generalized prediction model applied to the CHB-MIT database. The approach used the leave-one-patient-out (LOPO) cross-validation so that the seizures in the test phase belong to an exclusive patient that is not included in train data. The models are obtained from 48 statistical characteristics of the EEG microstates, and to distinguish the interictal and preictal states of EEG signals from epileptic patients, SVM and kNN classifiers are used. Results The best results achieved in the experiments were using the SVM RBF classifier, with 96.11% of sensitivity and FPR of 0.48 h −1 , and it is possible to predict an epileptic event with an average of 1.96 h in advance. Conclusion Experimental results showed EEG microstate analysis is a promising method for the prediction of epileptic seizures, and further studies with other characteristics and classifiers can allow better results.
更多查看译文
关键词
Epilepsy, EEG, Seizure prediction, Microstates, Machine learning
AI 理解论文
溯源树
样例
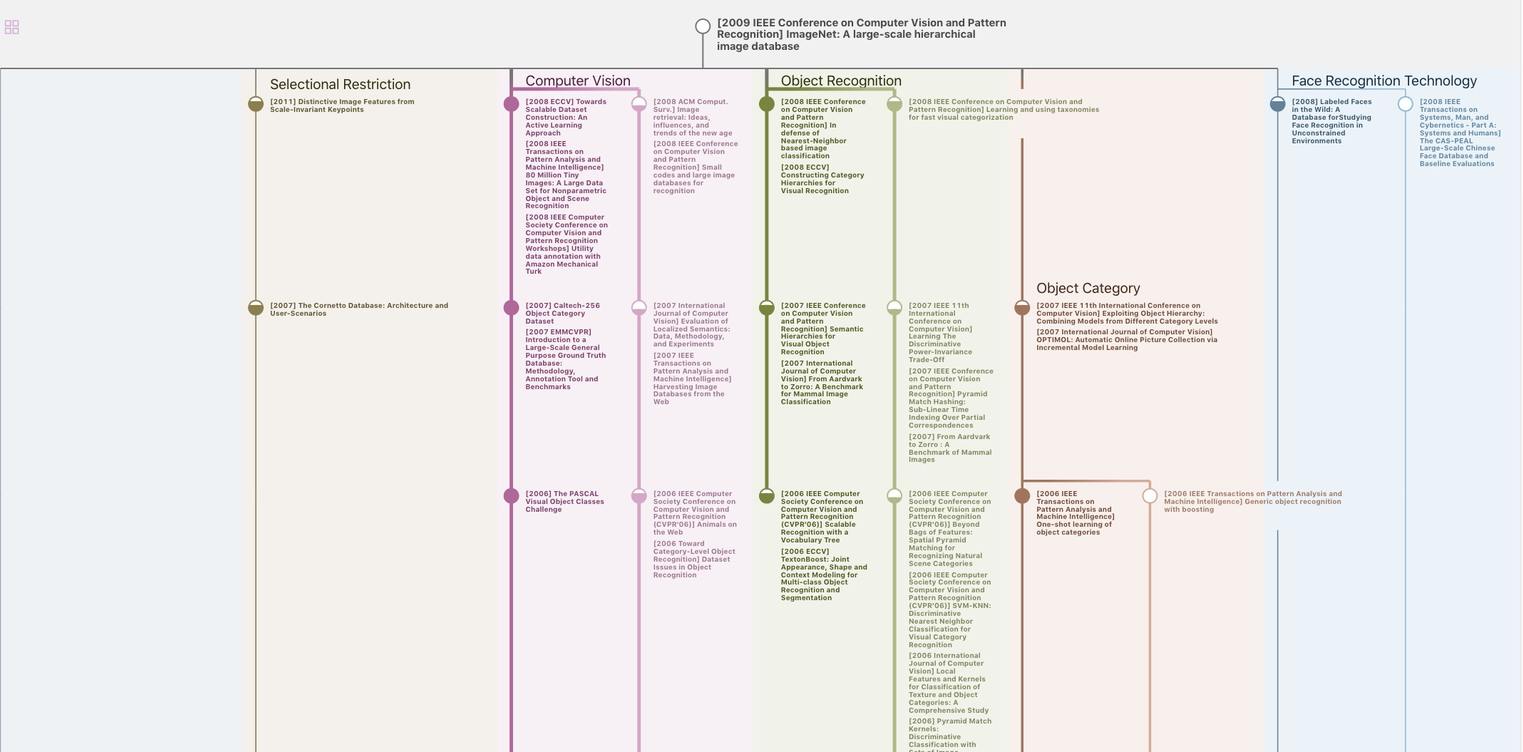
生成溯源树,研究论文发展脉络
Chat Paper
正在生成论文摘要