MICNet: Prediction of antibiotic susceptibility from microscopic images using transfer learning
medRxiv(2022)
摘要
Rapid susceptibility testing of bacterial isolates is crucial for anti-infective therapy, especially in critical cases such as bacteriaemia and sepsis. Nevertheless, empiric therapy is often initiated immediately and without testing because two days and more pass between a positive blood culture and a susceptibility profile, so in the meantime, the most likely pathogens are treated. However, current empiric recommendations are very generic. They often remain unmodified even in light of incoming, early data specific to a patient's case, such as positive blood culture microscopy. Part of the hesitancy to change treatments presumably stems from a lack of systematic integration of early information beyond expert intuition. To enable targeted antimicrobial therapy earlier in a case's progression, we developed a method to predict antimicrobial susceptibility from microscopy images of bacteria alone. Our proof-of-concept MICNet combines two neural nets in a new chimerical architecture. It is pre-trained on about 100 thousand antibiograms and fine-tuned with only five thousand microscopic images through transfer learning. Predicting susceptibility profiles of four representative species, we show high predictive performance with a mean F-score of nearly 85%. In addition, several qualitative assessments show that our chimerical net has learned substantial expert knowledge. Therefore, MICNet is the first step towards personalized empiric therapy, combining prior pathogen probabilities with patient-specific data.
更多查看译文
关键词
antibiotic susceptibility,transfer learning,microscopic images,prediction
AI 理解论文
溯源树
样例
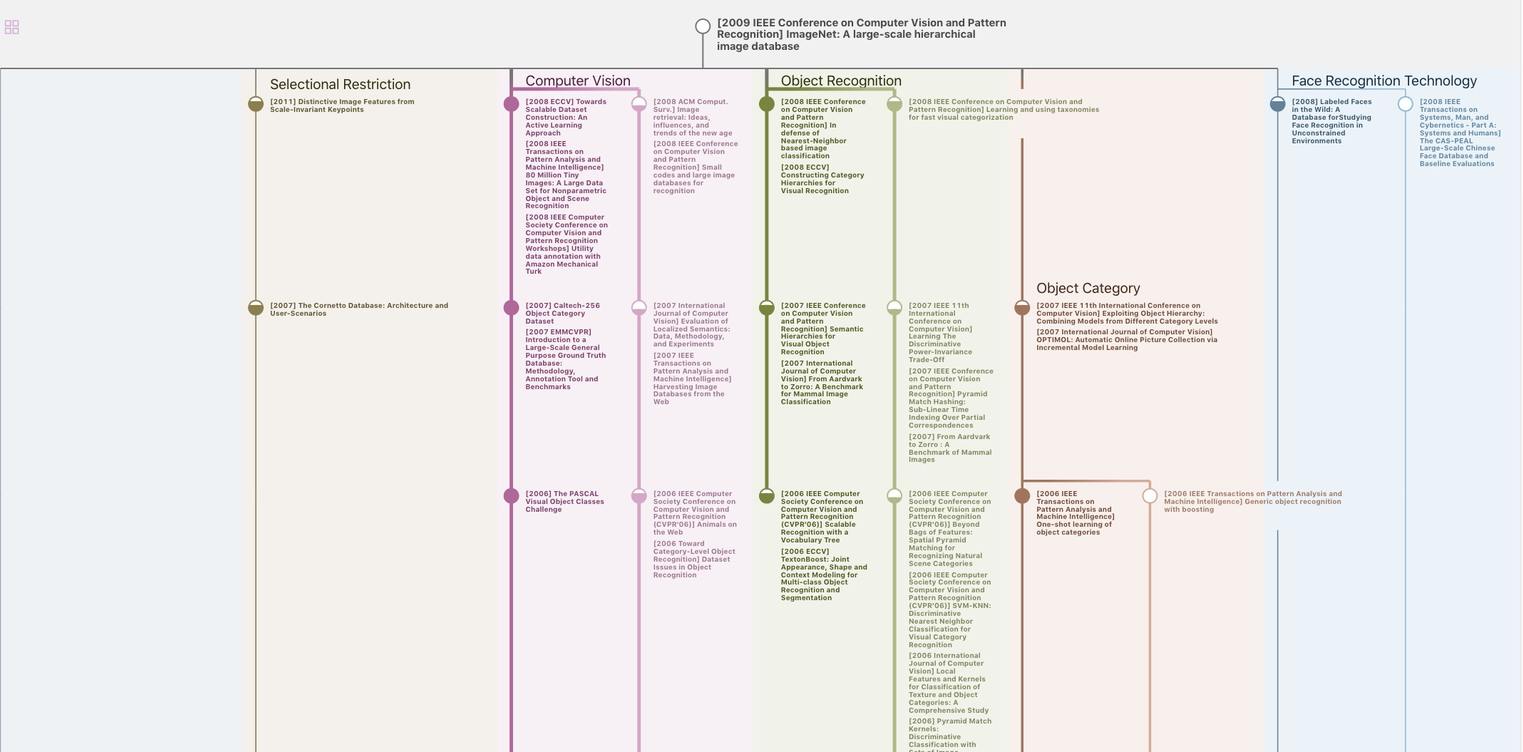
生成溯源树,研究论文发展脉络
Chat Paper
正在生成论文摘要