Results from feasibility and acceptability testing of Behavioral and Environmental Sensing and Intervention for Cancer (BESI-C): A smart health sensing system to support patients and family caregivers in managing cancer pain. (Preprint)
semanticscholar(2022)
摘要
BACKGROUND
Distressing cancer pain remains a serious symptom management issue for patients and family caregivers, especially in the home setting. Technology can support home-based cancer symptom management but must consider the experience of both patients and family caregivers, as well as the broader environmental context.
OBJECTIVE
To test feasibility and acceptability of a smart health sensing system, Behavioral and Environmental Sensing and Intervention for Cancer (BESI-C), designed to monitor, and ultimately support management of, cancer pain in the home setting.
METHODS
Dyads of patients with cancer and their primary family caregivers were recruited from an outpatient palliative care clinic at an academic medical center in the Southeastern U.S. BESI-C was deployed in dyad homes for approximately two weeks each. Data were collected via: 1) environmental sensors that measure ambient noise, humidity, barometric pressure, light, and temperature; 2) Bluetooth beacons to help localize dyad position; and 3) smart watches worn by both patients and caregivers equipped with heartrate monitors, accelerometers, and a custom application to deliver ecological momentary assessments (EMAs). EMAs enabled dyads to record and characterize pain events from both their own and their partner’s perspective. Sensor data streams were integrated to describe and explore the context for cancer pain events. Feasibility was assessed technically (by scoring each deployment regarding fidelity of data capture across system components) and procedurally (e.g., deployment process, participant recruitment). Acceptability was assessed by post-deployment surveys and structured interviews with participants.
RESULTS
5 deployments (n=10 participants; 5 dyads of patients and primary family caregivers) were completed, and a total of 283 unique pain events were recorded. Using our ‘BESI-C Performance Scoring Instrument’, the overall feasibility technical score for deployments was 86.4/100. Procedural feasibility challenges included: rural location of dyads; smart watch battery life and EMA reliability; and length of time required for deployment installation. Post-deployment acceptability Likert surveys (1, strongly disagree; 5, strongly agree), found dyads disagreed that BESI-C was a burden (1.7/5) or compromised their privacy (1.9/5) and agreed the system collected helpful information to better manage cancer pain (4.6/5). Participants also expressed interest in seeing their own individual data (4.4/5) and strongly agreed it is important that data collected by BESI-C are shared with their respective partner (4.8/5) and healthcare provider (4.8/5). Qualitative feedback from participants suggests BESI-C positively improved communication regarding pain management. Importantly, we demonstrated proof of concept that seriously ill patients and their caregivers will mark pain events in real time using a smart watch.
CONCLUSIONS
It is feasible to deploy BESI-C, and dyads find the system acceptable. Leveraging human-centered design and the collection of heterogenous environmental, physiological, and behavioral data, BESI-C offers an innovative approach to monitor cancer pain, mitigate escalation of pain and distress, and improve symptom management self-efficacy. Future work will: (shorter-term) 1) test BESI-C in a larger, more diverse sample; 2) continue to streamline system architecture; 3) deploy a no-contact, self-installation system in response to the COVID-19 pandemic and to enhance scalability; 4) explore how to best share data visualizations of collected data with key stakeholders; and 5) (longer-term) inform design of and deliver just-in-time personalized pain management interventions to patients and caregivers.
CLINICALTRIAL
n/a
INTERNATIONAL REGISTERED REPORT
RR2-10.2196/16178
更多查看译文
AI 理解论文
溯源树
样例
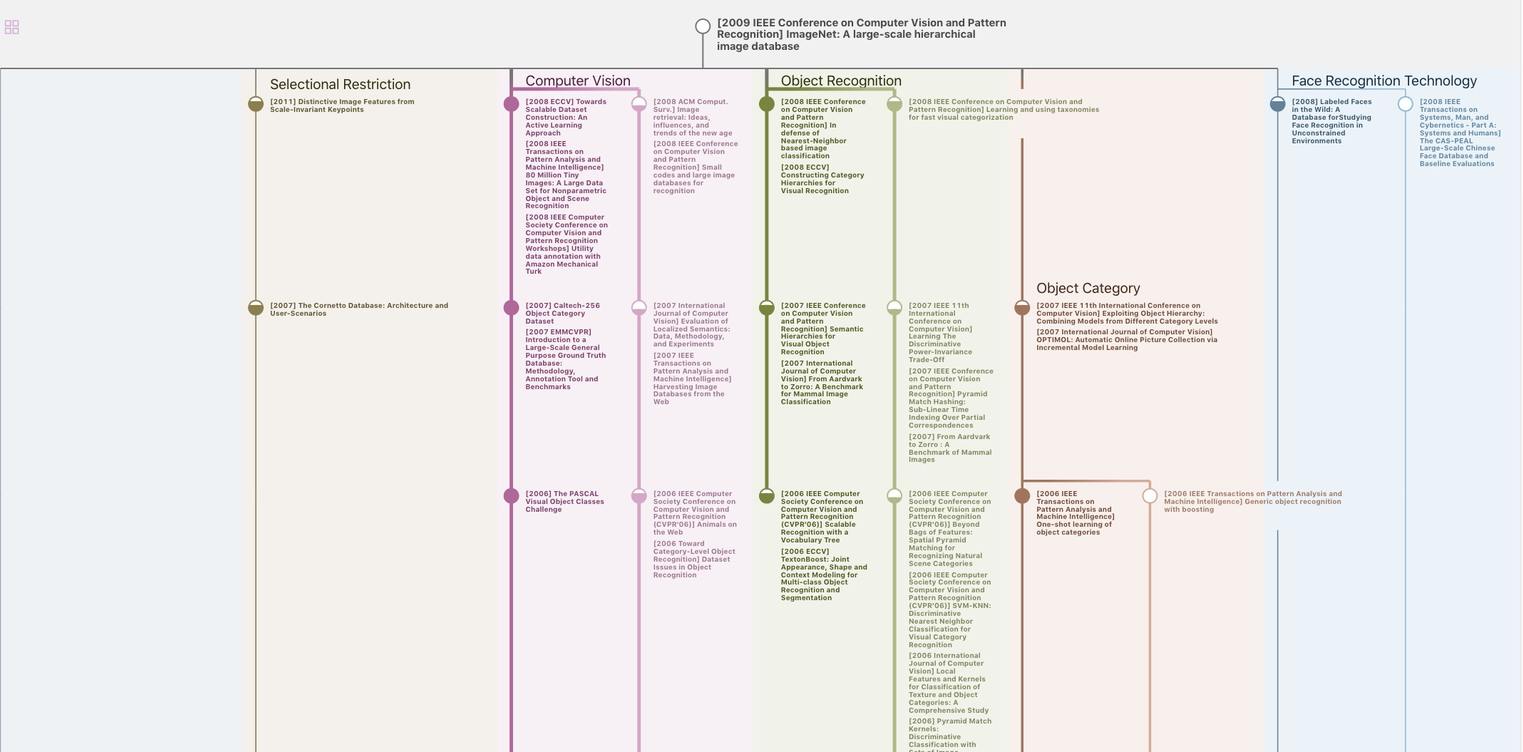
生成溯源树,研究论文发展脉络
Chat Paper
正在生成论文摘要