Benchmarking Robustness to Natural Distribution Shifts for Facial Analysis
semanticscholar(2021)
Abstract
During the deployment of machine learning models, performance degradation can occur compared to the training and validation data. This generalization gap can appear for a variety of reasons and be particularly critical in applications where certain groups of people are disadvantaged by the outcome, e.g. facial analysis. Literature provides a vast amount of methods to either perform robust classification under distribution shifts or at least to express the uncertainty caused by the shifts. However, there is still a need for data that exhibit different natural distribution shifts considering specific subgroups to test these methods. We use a balanced dataset for facial analysis and introduce subpopulation shifts, spurious correlations, and subpopulation-specific label noise. This forms our basis to investigate to what extent known approaches for calibrating neural networks remain reliable under these specified shifts. Each of the modifications leads to performance degradation, but the combination of ensembles and temperature scaling is particularly useful to stabilize the calibration over the shifts.
MoreTranslated text
AI Read Science
Must-Reading Tree
Example
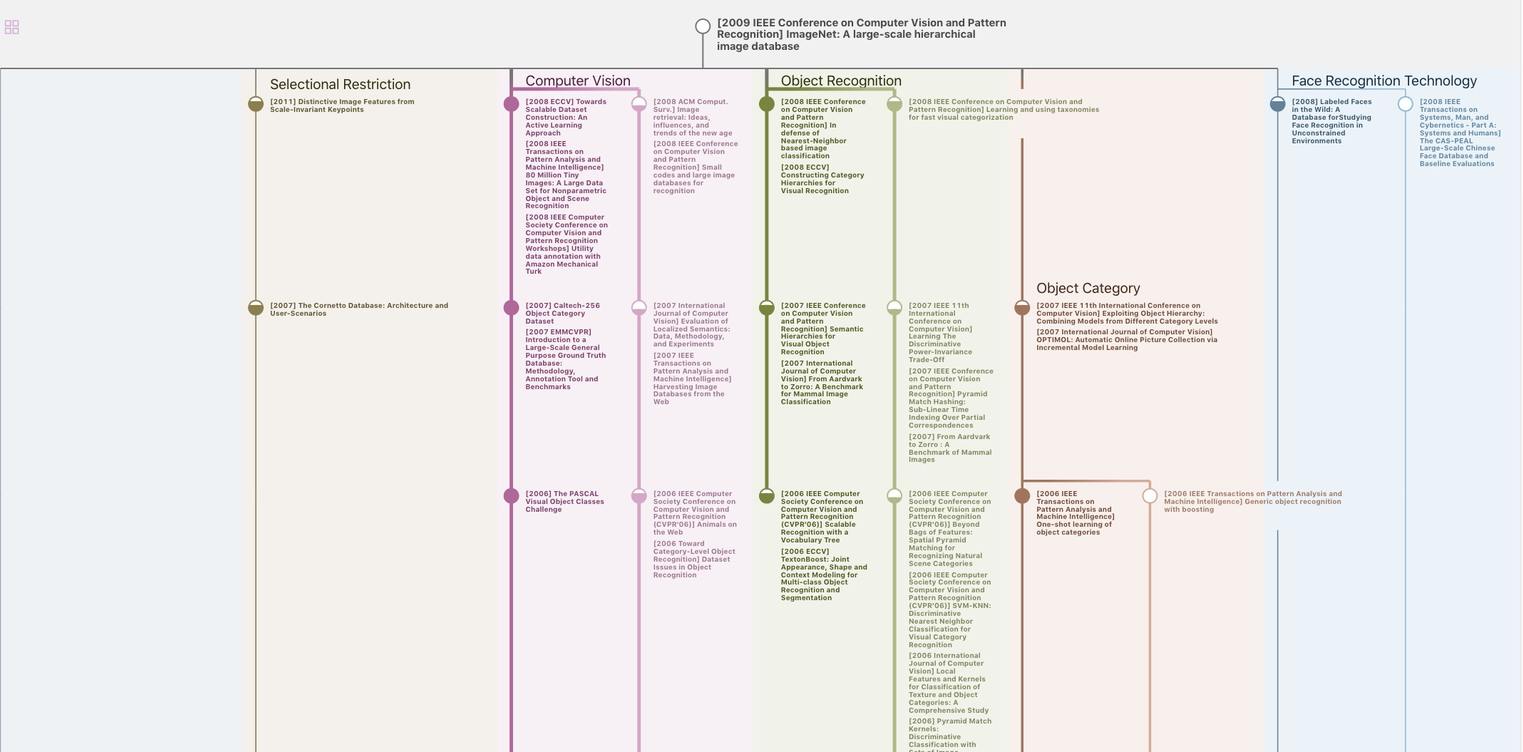
Generate MRT to find the research sequence of this paper
Chat Paper
Summary is being generated by the instructions you defined