Deep Illumination-Enhanced Face Super-Resolution Network for Low-Light Images
ACM TRANSACTIONS ON MULTIMEDIA COMPUTING COMMUNICATIONS AND APPLICATIONS(2022)
摘要
Face images are typically a key component in the fields of security and criminal investigation. However, due to lighting and shooting angles, faces taken under low-light conditions are often difficult to recognize. Face super-resolution (FSR) technology can restore high-resolution faces based on low-resolution inputs. However, existing face super-resolution methods typically rely on prior knowledge of inaccurate faces estimated from low-resolution images. Faces restored by low-light inputs may suffer from problems such as low brightness and many missing details. In this article, we proposed an Illumination-Enhanced Face Super-Resolution (IEFSR) model that can progressively super-resolve low-light faces of 32 x 32 pixels by an upscaling factor of 8. While reconstructing the low-light low-resolution face into a clear and high-quality face, we introduce a coarse low-resolution (LR) restoration network to recover the LR face details hidden in the dark. In the generator, we use a series of style blocks with noise to make the generated faces appear to have a more realistic visual aesthetic. Additionally, we introduce spectrum normalization in the discriminator to improve training stability. Extensive experimental evaluations show that the proposed IEFSR yields visually and metrically more attractive results than existing state-of-the-art FSR methods.
更多查看译文
关键词
Face super-resolution,low-light face images,spectrum normalization,generative adversarial network
AI 理解论文
溯源树
样例
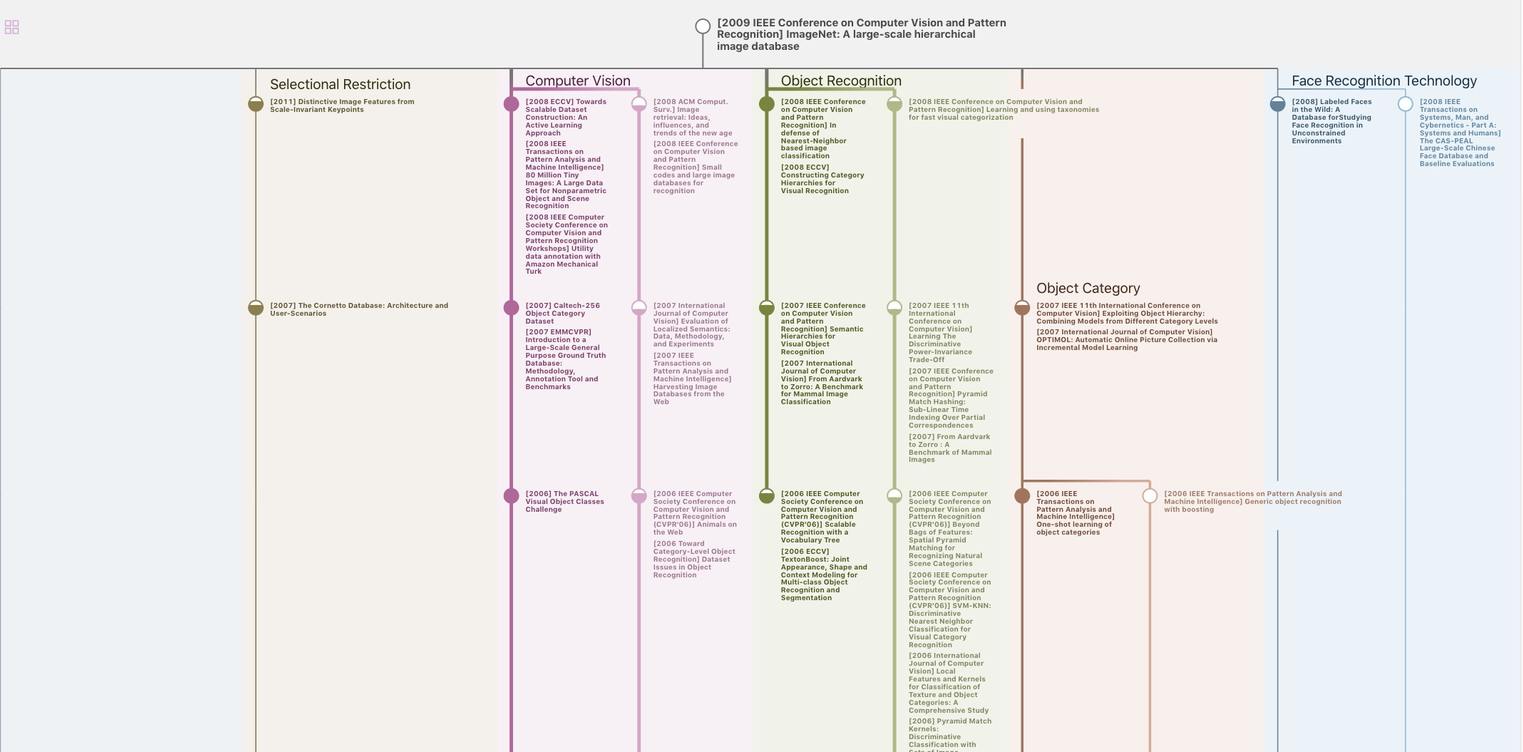
生成溯源树,研究论文发展脉络
Chat Paper
正在生成论文摘要