Piezoresistor defect classification using convolutional neural networks based on incremental branch growth
Multimedia Tools and Applications(2022)
摘要
Surface defects significantly deteriorate piezoresistor quality. We propose an accurate end-to-end classification method for piezoresistor surface defects based on an incremental branch growth convolutional neural network (IBG-CNN) for automatic CNN construction. First, an incremental branch (IB) is proposed to grow a CNN dynamically. Then, IBG-CNN establishes and trains a starting network based on IB, which is then grown continuously using the IBG algorithm. The next generation of the growing network is trained using the pretrained previous generation, which significantly accelerates the search process of the network model. A CNN based on IBG-CNN for classifying six piezoresistor defect types was automatically built on one GPU in only approximately 16 h. A test dataset of 6248 images was evaluated using the mean average precision (mAP). The experimental results demonstrate that the classification accuracy of our algorithm (mAP = 0.935) is higher than or very close to those of state-of-the-art methods, i.e., conventional CNN-based methods and the efficient neural architecture search (ENAS).
更多查看译文
关键词
Neural architecture search,Incremental branch growth,Convolutional neural networks,Surface defect classification
AI 理解论文
溯源树
样例
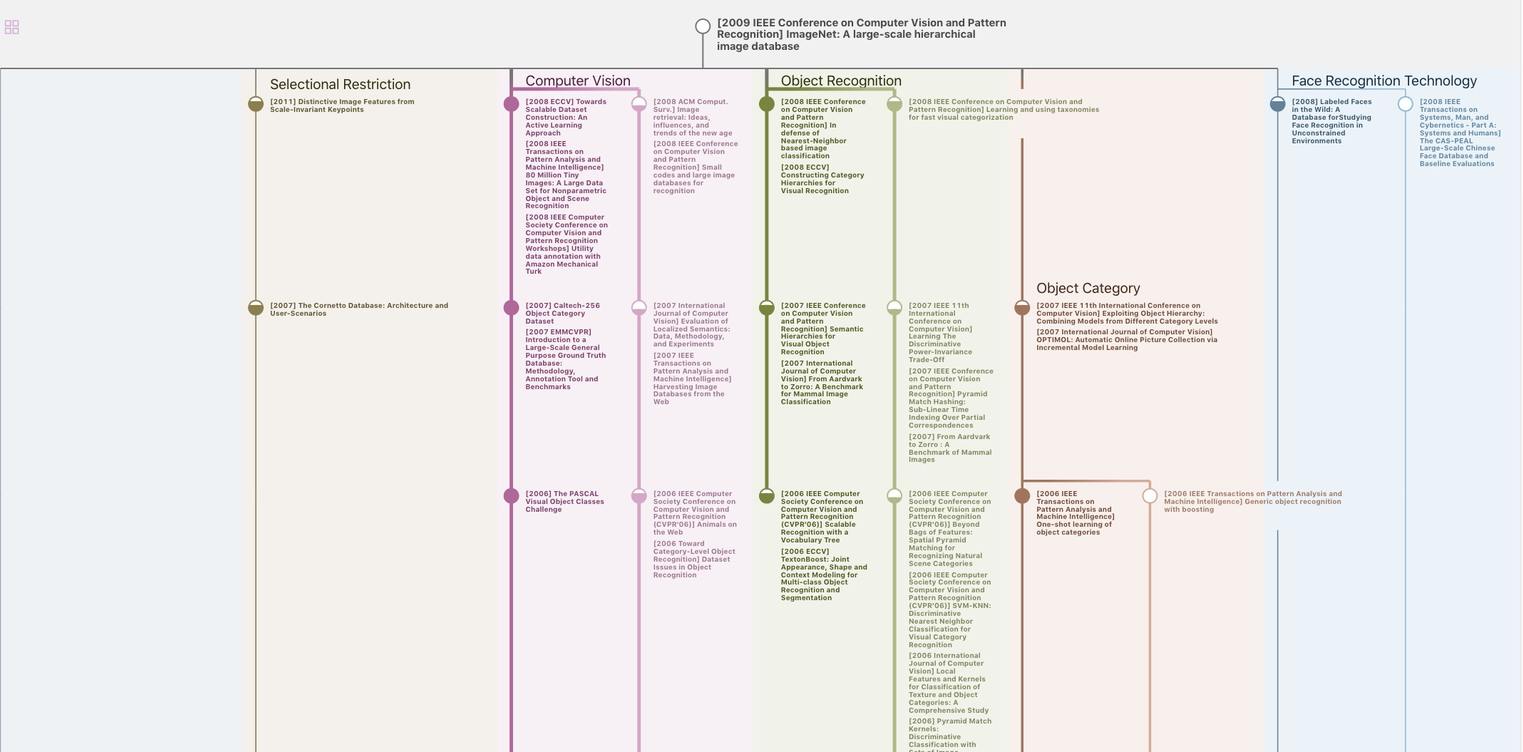
生成溯源树,研究论文发展脉络
Chat Paper
正在生成论文摘要