Enhancing the Explainability of AI Models in Nuclear Power Plants with Layer-wise Relevance Propagation
semanticscholar(2021)
Abstract
With the rapid advance of artificial intelligence (AI) technology, there have been enormous number of applications across the various fields. Nuclear field is also following this trend, and there have been many studies that utilize AI models for solving problems such as event diagnosis and automated/autonomous operation. However, deep neural network (DNN) which takes the biggest portion of recent AI technology applications has a limitation that it is not transparent and has low explainability. In the case of DNN-based model, it is difficult to know the internal logic of the model or how the model deduces outputs from given input. Due to this limitation, there is a hesitation on practical application of DNN-based models to the safety-critical fields and the fields related to moral/legal issues, although their performances are acceptable. To overcome the limitation of low explainability, a number of explainable AI (XAI) methods have been proposed. XAI methods can provide detail explanations such as model’s internal logics and relations between inputs and outputs. However, although the explainability issue is crucial for safety-critical nuclear field, there is a lack of studies that dealing with XAI. In this study, as an effort to enhance the explainability and the practicality of AI models in nuclear field, layerwise relevance propagation (LRP) [1] was investigated, which is one of the XAI methods that has shown better performance in many applications compared to other XAI methods. The rest of paper is organized as follows. In chapter 2, brief explanations on XAI and LRP are provided. In chapter 3, experiments for feasibility check are described and chapter 4 concludes the paper.
MoreTranslated text
AI Read Science
Must-Reading Tree
Example
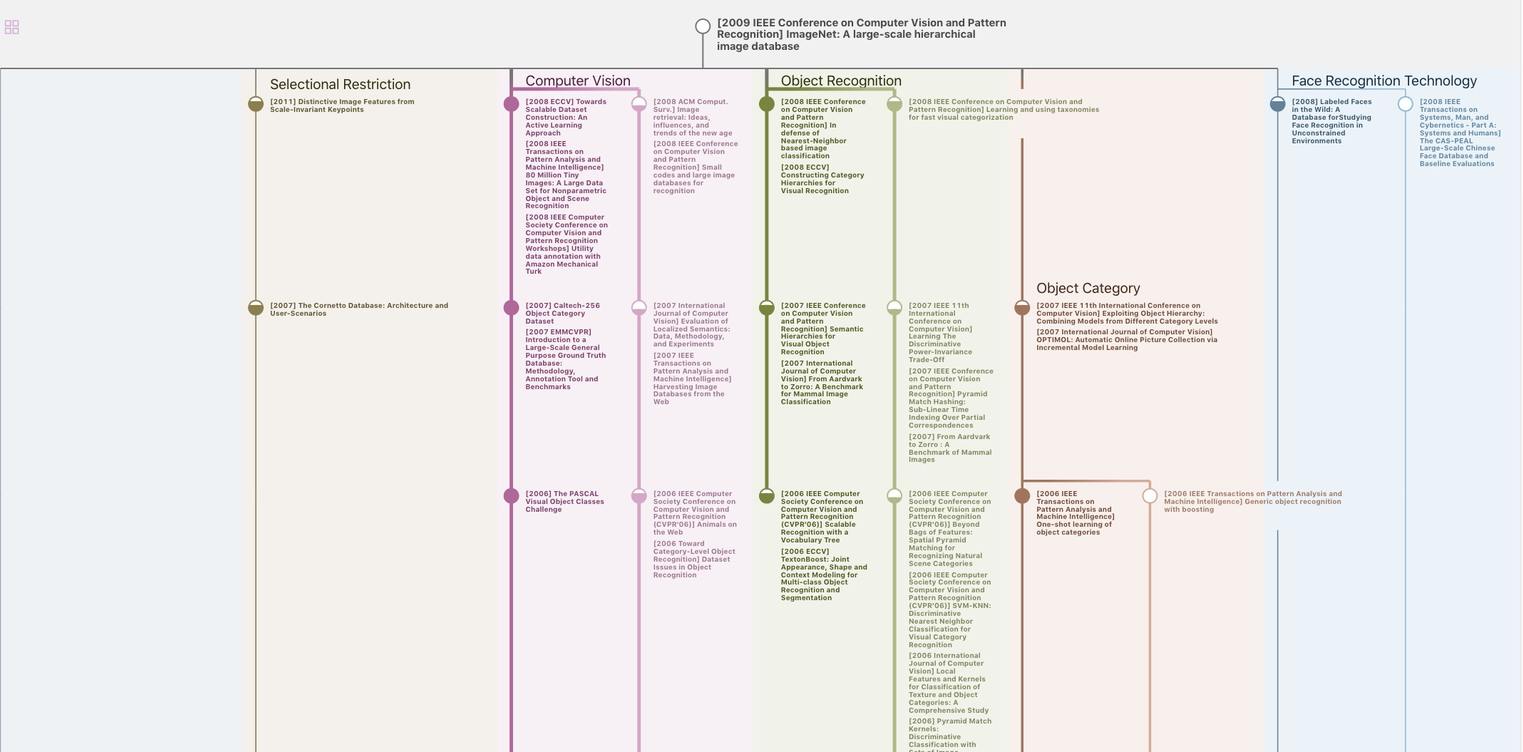
Generate MRT to find the research sequence of this paper
Chat Paper
Summary is being generated by the instructions you defined