Improving Edge Elasticity via Decode Offload
semanticscholar(2022)
摘要
Visual analytics on recently-captured data from video cameras has emerged as an important class of workloads in edge computing. These workloads make intense processing demands on cloudlets, whose elasticity is limited by their smaller physical and electrical footprint relative to exascale cloud data centers. In this paper, we show how cloudlet elasticity can be improved by offloading visual data decoding. We define a new data access API that embodies decode offload, thereby avoiding application-level decoding of visual data. Using thermal, power density and data copying considerations, we identify cloudlet storage as the optimal location for placement of the decode function. Using a proof-of-concept implementation, we show that this approach can lower cloudlet CPU utilization by up to 50–80%, and deliver up to 3.5x improvement in the elapsed time of a typical visual analytics pipeline. This research was sponsored by National Science Foundation award number: CNS1518865; by United States Defense Advanced Research Projects Agency award number: HR001117C0051; by Intel Corporation award number: A018540296213611; and by a Croucher Foundation Scholarship for Doctoral Study. Additional support was provided by Vodafone, Deutsche Telekom, Crown Castle, InterDigital, Seagate, Microsoft, VMware, and the Conklin Kistler family fund. The views and conclusions contained in this document are those of the authors and should not be interpreted as representing the official policies, either expressed or implied, of any sponsoring institution, the U.S. government or any other entity.
更多查看译文
AI 理解论文
溯源树
样例
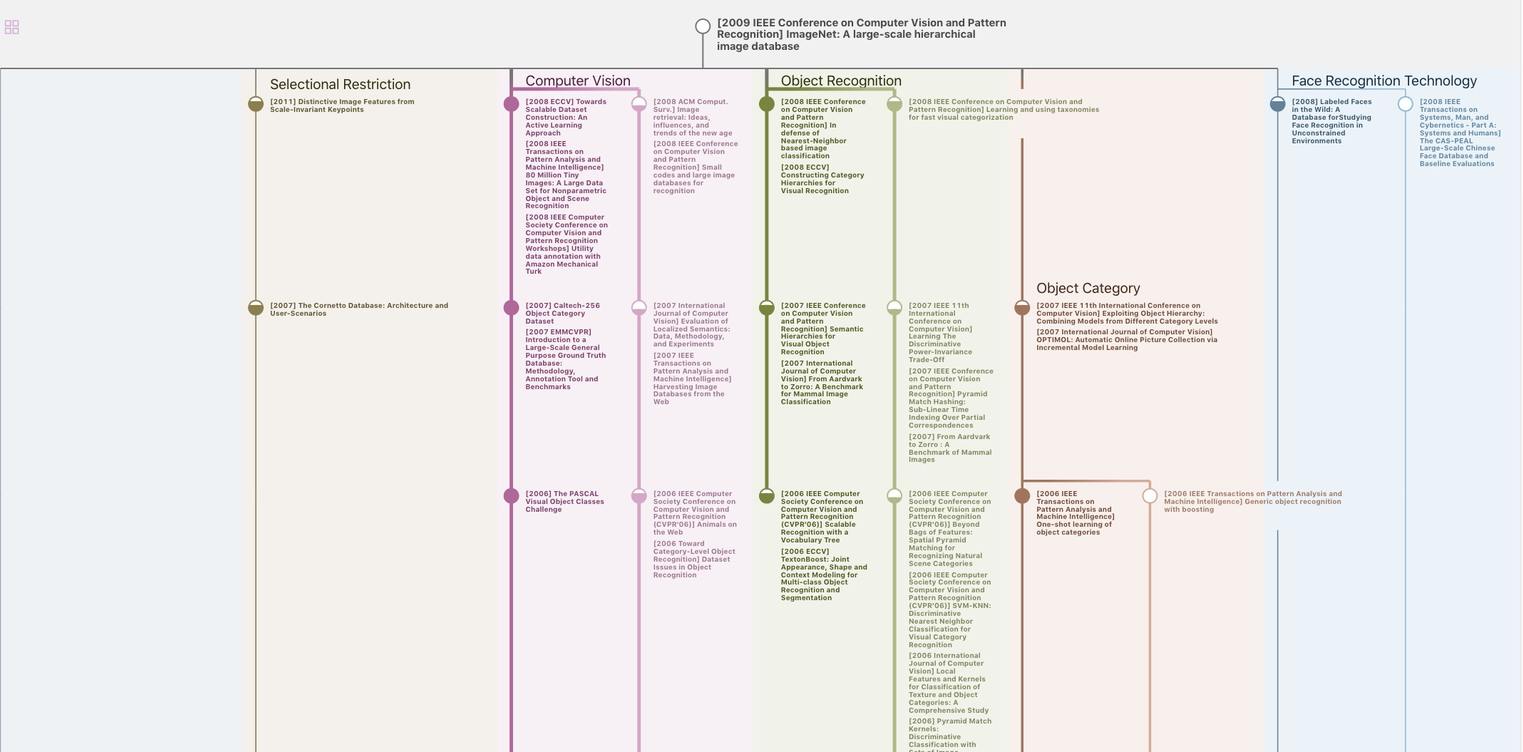
生成溯源树,研究论文发展脉络
Chat Paper
正在生成论文摘要