Demonstration-Guided Q-Learning
semanticscholar(2021)
Abstract
In many challenging reinforcement learning (RL) settings, demonstrations are used to assist with exploration by allowing policies or value functions to directly learn from successful experience. In this work, we explore additional ways to utilize expert demonstrations to expedite training in value-based RL. In particular, we propose Demonstration-Guided Q-Learning (DGQL), an algorithm that noisily replays expert demonstrations to guide exploration and enable more efficient Q-value propagation in value-based RL methods. Contrary to common methods that utilize demonstrations in the context of value-based RL, we show that DGQL effectively leverages demonstrations to guide exploration via a replaying curriculum that relaxes common assumptions in simulated environments. In addition to analyzing the empirical benefits of more efficient value propagation, we show that DGQL is able to scale to difficult vision-based robotic manipulation tasks.
MoreTranslated text
AI Read Science
Must-Reading Tree
Example
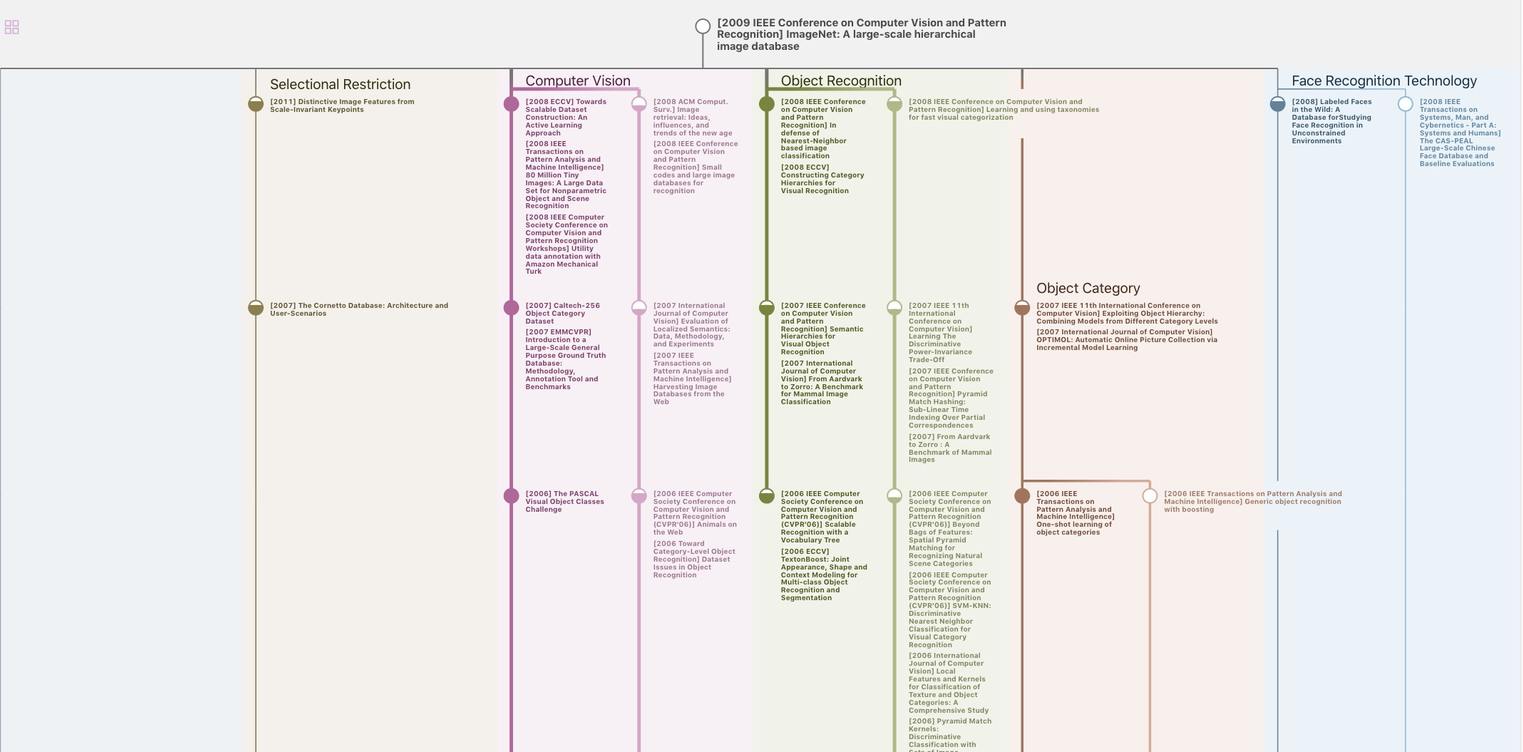
Generate MRT to find the research sequence of this paper
Chat Paper
Summary is being generated by the instructions you defined