A machine learning-based approach to determine infection status in recipients of BBV152 whole virion inactivated SARS-CoV-2 vaccine for serological surveys
medRxiv(2021)
Abstract
Data science has been an invaluable part of the COVID-19 pandemic response with multiple applications, ranging from tracking viral evolution to understanding the effectiveness of interventions. Asymptomatic breakthrough infections have been a major problem during the ongoing surge of Delta variant globally. Serological discrimination of vaccine response from infection has so far been limited to Spike protein vaccines used in the higher-income regions. Here, we show for the first time how statistical and machine learning (ML) approaches can discriminate SARS-CoV-2 infection from immune response to an inactivated whole virion vaccine (BBV152, Covaxin, India), thereby permitting real-world vaccine effectiveness assessments from cohort-based serosurveys in Asia and Africa where such vaccines are commonly used. Briefly, we accessed serial data on Anti-S and Anti-NC antibody concentration values, along with age, sex, number of doses, and number of days since the last vaccine dose for 1823 Covaxin recipients. An ensemble ML model, incorporating a consensus clustering approach alongside the support vector machine (SVM) model, was built on 1063 samples where reliable qualifying data existed, and then applied to the entire dataset. Of 1448 self-reported negative subjects, 724 were classified as infected. Since the vaccine contains wild-type virus and the antibodies induced will neutralize wild type much better than Delta variant, we determined the relative ability of a random subset of such samples to neutralize Delta versus wild type strain. In 100 of 156 samples, where ML prediction differed from self-reported uninfected status, Delta variant, was neutralized more effectively than the wild type, which cannot happen without infection. The fraction rose to 71.8% (28 of 39) in subjects predicted to be infected during the surge, which is concordant with the percentage of sequences classified as Delta (75.6%-80.2%) over the same period.
MoreTranslated text
AI Read Science
Must-Reading Tree
Example
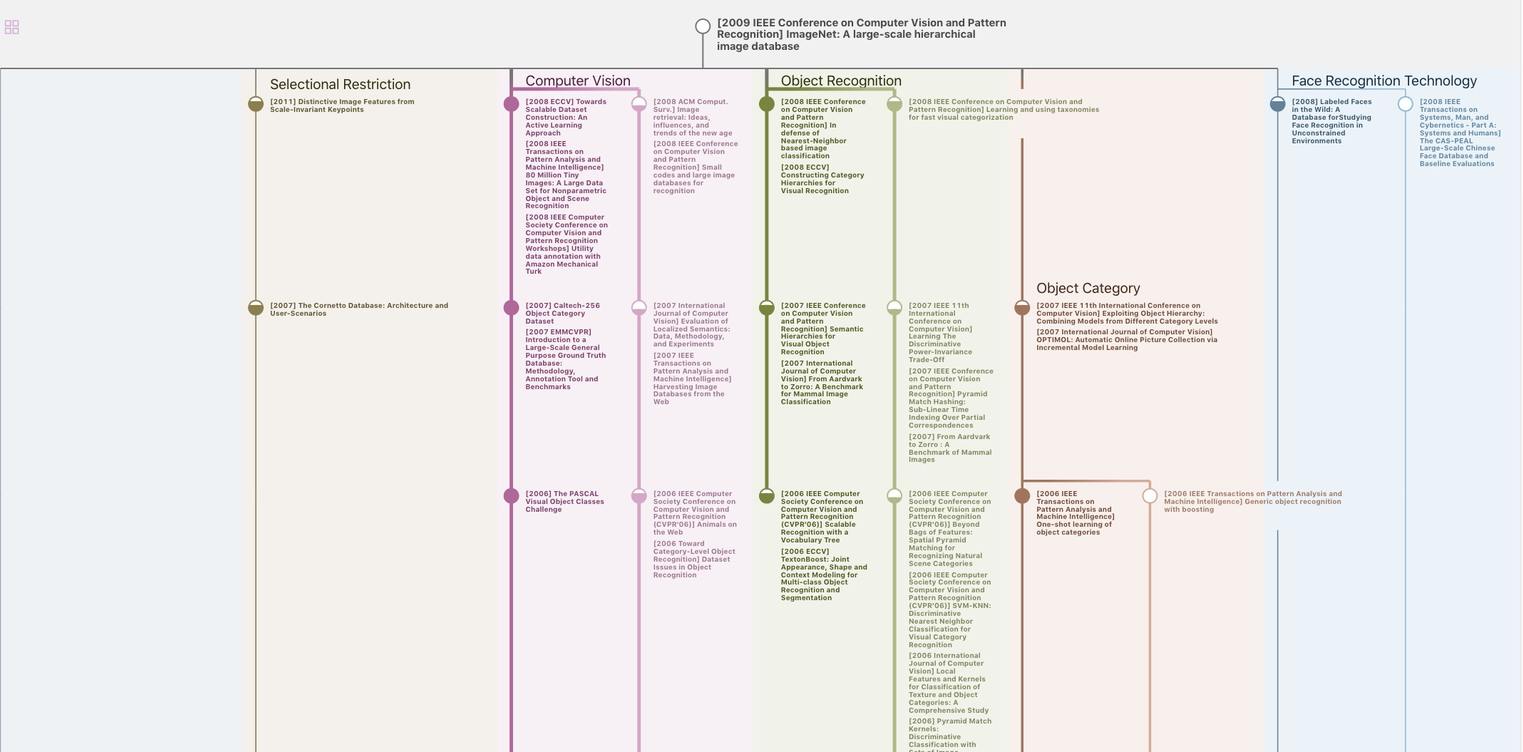
Generate MRT to find the research sequence of this paper
Chat Paper
Summary is being generated by the instructions you defined