Generative adversarial network with hybrid attention and compromised normalization for multi-scene image conversion
Neural Computing and Applications(2022)
Abstract
In order to generate high-quality realistic images, this paper proposes an image conversion algorithm based on hybrid attention generation adversarial network. This network is composed of the generator and discriminator, both of which are jointly trained through the loss function. The generator is constructed by using a three-stage structure of down-sampling, residual and up-sampling blocks where the residual block uses a hybrid attention mechanism. The compromised instance and layer normalization is also proposed by weighting the output of the fully connected layer. The multi-scale PatchGAN is introduced as the discriminator. The proposed network can produce more realistic images using a new loss function, which comprises four iterms: generation adversarial loss, L_1 regularization loss, VGG loss and feature matching loss. The experimental results demonstrated that the proposed method can produce more realistic and detailed images than the state-of-the-art methods.
MoreTranslated text
Key words
Image conversion, Deep learning, Generative adversarial networks, Image generation
AI Read Science
Must-Reading Tree
Example
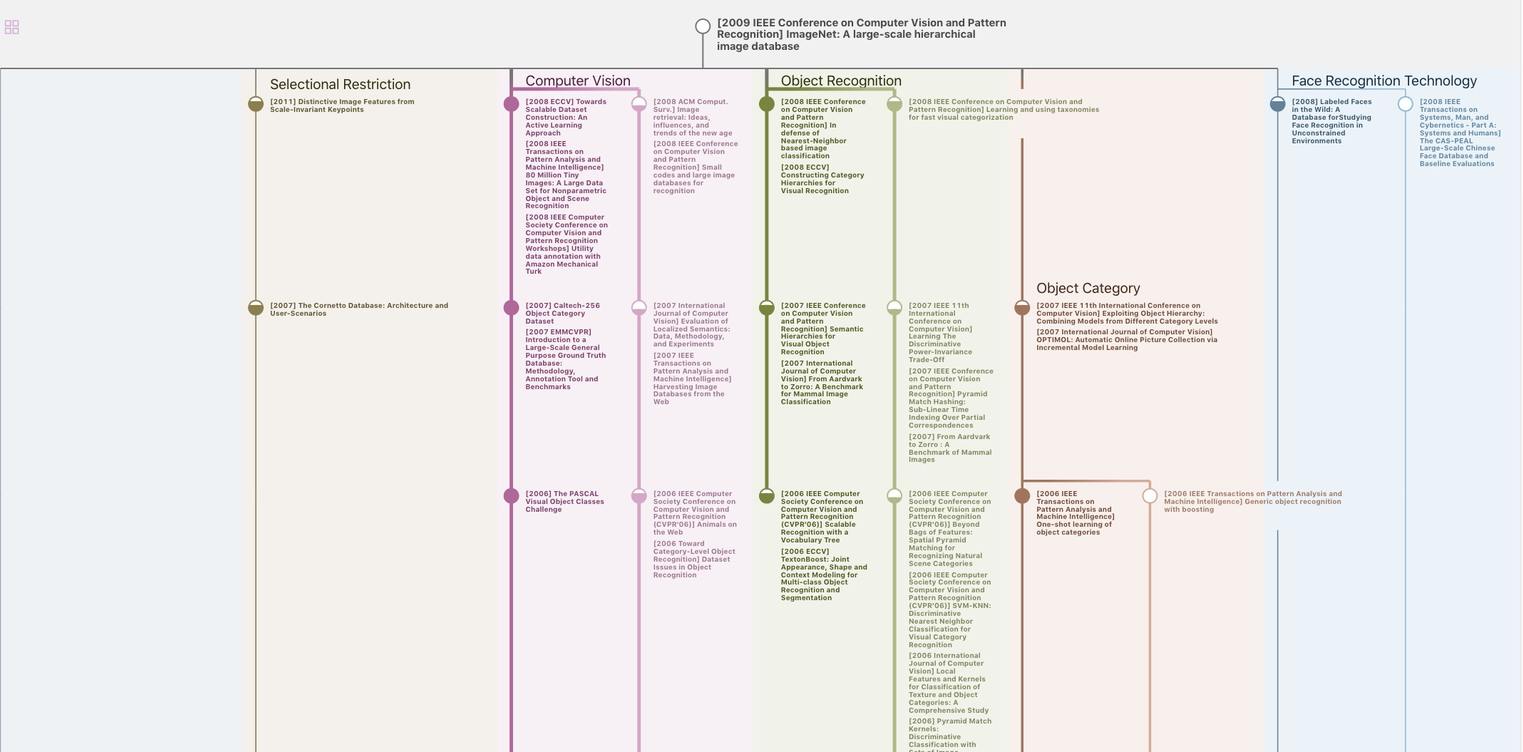
Generate MRT to find the research sequence of this paper
Chat Paper
Summary is being generated by the instructions you defined