SAMCNet for Spatial-configuration-based Classification: A Summary of Results
arXiv (Cornell University)(2021)
摘要
The goal of spatial-configuration-based classification is to build a classifier to distinguish two classes (e.g., responder, non-responder) based on the spatial arrangements (e.g., spatial interactions between different point categories) given multi-category point data from two classes. This problem is important for generating hypotheses in medical pathology towards discovering new immunotherapies for cancer treatment as well as for other applications in biomedical research and microbial ecology. This problem is challenging due to an exponential number of category subsets which may vary in the strength of spatial interactions. Most prior efforts on using human selected spatial association measures may not be sufficient for capturing the relevant (e.g., surrounded by) spatial interactions which may be of biological significance. In addition, the related deep neural networks are limited to category pairs and do not explore larger subsets of point categories. To overcome these limitations, we propose a Spatial-interaction Aware Multi-Category deep neural Network (SAMCNet) architecture and contribute novel local reference frame characterization and point pair prioritization layers for spatial-configuration-based classification. Extensive experimental results on multiple cancer datasets show that the proposed architecture provides higher prediction accuracy over baseline methods.
更多查看译文
关键词
classification,spatial-configuration-based
AI 理解论文
溯源树
样例
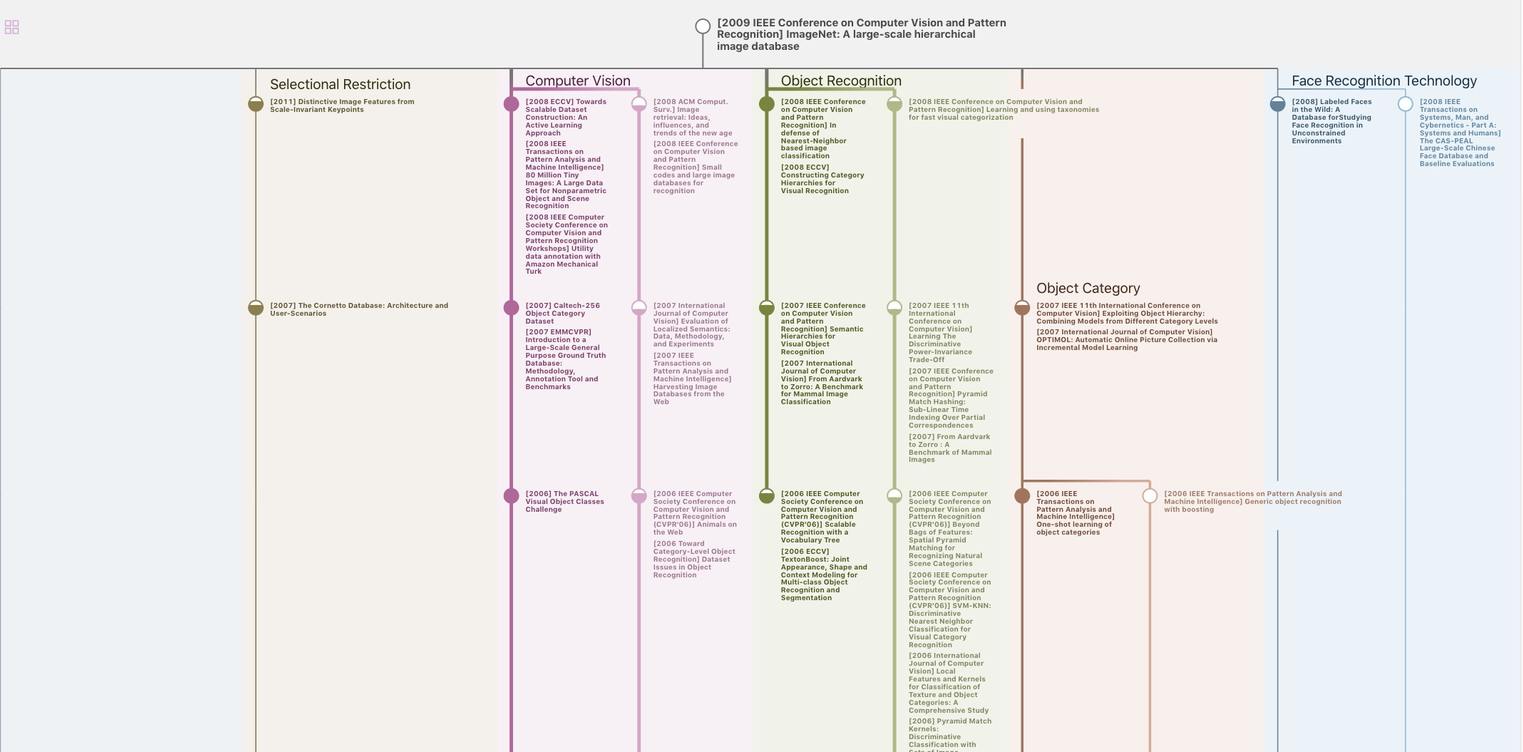
生成溯源树,研究论文发展脉络
Chat Paper
正在生成论文摘要