An iterative solution for improving the generalization ability of unsupervised skeleton motion retargeting
Computers & Graphics(2022)
Abstract
In this paper, a deep learning-based iterative solving method for unsupervised motion retargeting is proposed. Unlike existing methods, we do not utilize generative-adversarial-network-based (GANbased) models as a solution for unsupervised motion retargeting, but utilize an iterative solution to yield retargeted motions based on a trained motion autoencoder. The proposed method includes a training phase and an execution phase. In the training phase, an autoencoder named the iterative motion retargeting network (ItMRnet) learns a continuous latent space of natural motion data. At the execution phase, the autoencoder-based hidden units are iteratively adjusted to yield retargeted motion sequences by minimizing the loss functions defined in motion space. Existing GAN-based methods need to train discriminators to distinguish whether the output of the generator is real or fake motion data. Obviously, the discriminator would have a poor discrimination ability for characters and motions that are not included in the training dataset. The proposed method does not use adversarial training which hurt generalization ability, but yields motion from a continuous latent space that represents a motion manifold, and can generate motions for those characters not included in the training dataset. That is, our method generalizes well to unseen motions and characters. The experimental results demonstrate that our method clearly outperforms state-of-the-art methods by reducing the motion retargeting error and motion deformation phenomenon, especially when the source or target character is not seen in the training dataset. (c) 2022 Elsevier Ltd. All rights reserved.
MoreTranslated text
Key words
Motion retargeting,Latent space,Iterative solving,Skeleton motion,Generalization ability
AI Read Science
Must-Reading Tree
Example
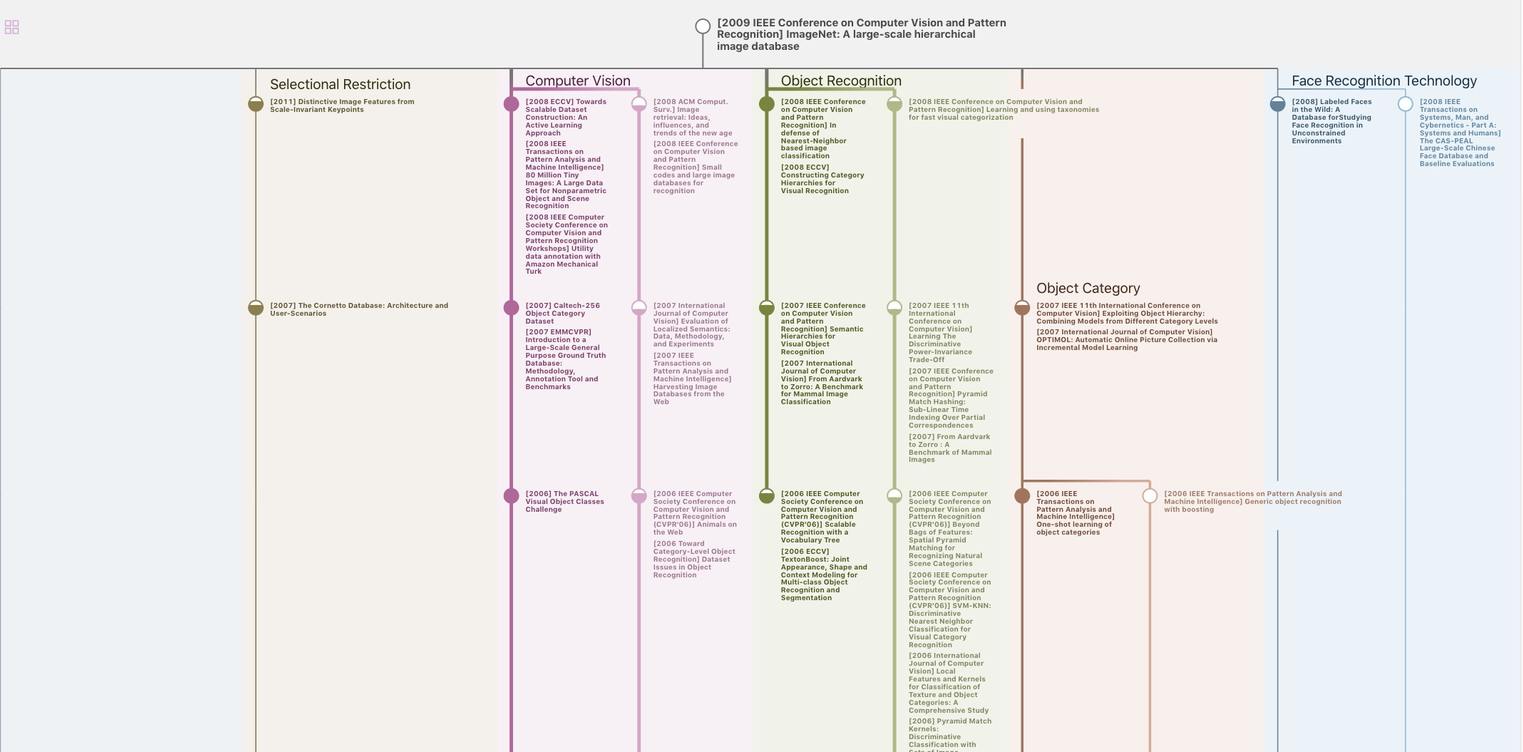
Generate MRT to find the research sequence of this paper
Chat Paper
Summary is being generated by the instructions you defined