Self-adjusting population sizes for the (1, )-EA on monotone functions
CoRR(2023)
摘要
We study the (1, lambda)-EA with mutation rate c/n for c <= 1, where the population size is adaptively controlled with the (1 : s + 1)-success rule. Recently, Hevia Fajardo and Sudholt have shown that this setup with c =1 is efficient on ONEMAX for s < 1, but inefficient if s >= 18. Surprisingly, the hardest part is not close to the optimum, but rather at linear distance. We show that this behaviour is not specific to ONEMAX. If s is small, then the algorithm is efficient on all monotone functions, and if s is large, then it needs super polynomial time on all monotone functions. In the former case, for c < 1 we show a O (n) upper bound for the number of generations and O (n log n) for the number of function evaluations, and for c =1 we show O (n log n) generations and O (n(2) log log n) evaluations. We also show formally that optimization is always fast, regardless of s, if the algorithm starts in proximity of the optimum. All results also hold in a dynamic environment where the fitness function changes in each generation. An extended abstract, containing only the results without proofs, has been published at the PPSN conference [1]. (c) 2023 The Author(s). Published by Elsevier B.V. This is an open access article under the CC BY license (http://creativecommons .org /licenses /by /4 .0/).
更多查看译文
关键词
Parameter control,Self-adaptation,(1,lambda)-EA,One-fifth rule,Monotone functions,Dynamic environments,Evolutionary algorithm
AI 理解论文
溯源树
样例
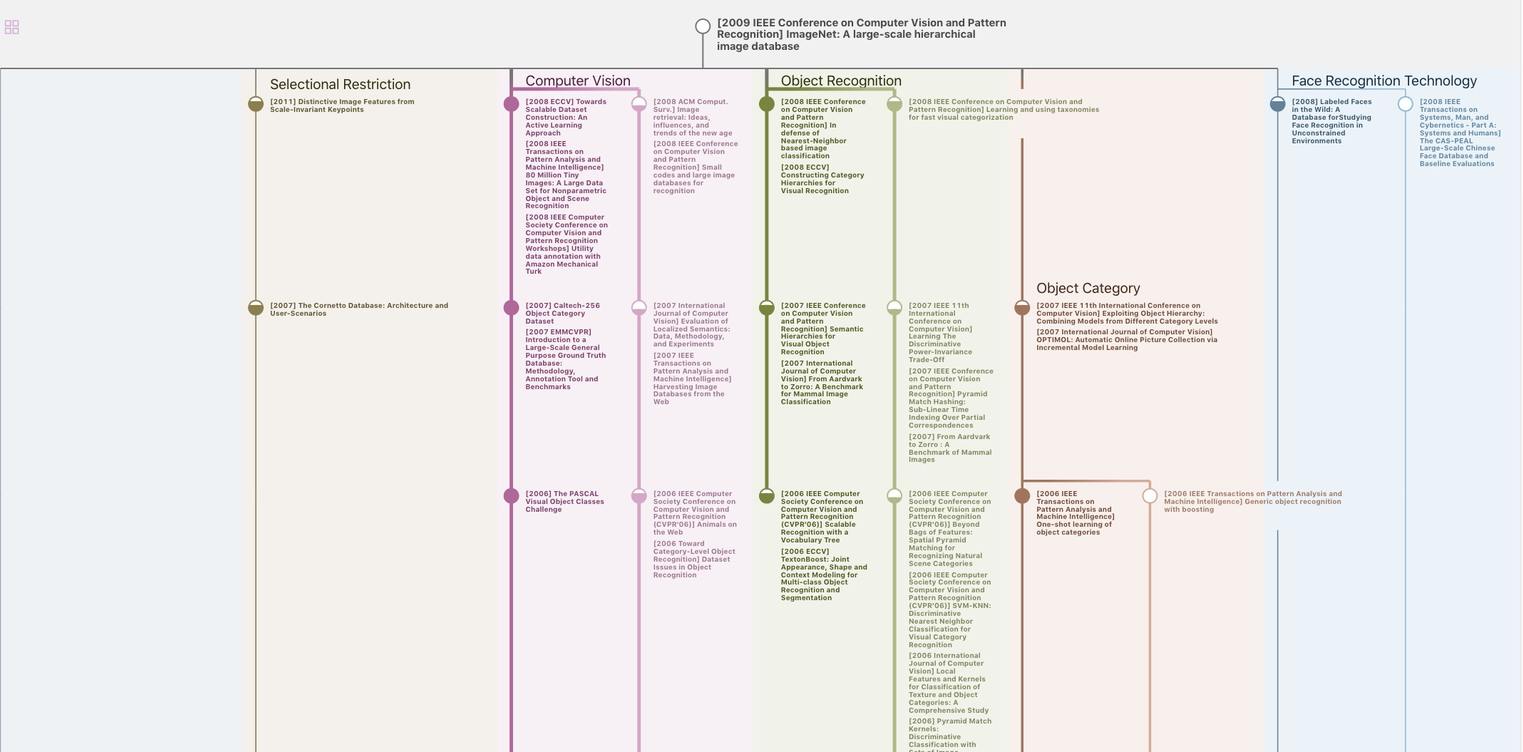
生成溯源树,研究论文发展脉络
Chat Paper
正在生成论文摘要