A fast collapsed Gibbs sampler for frequency domain operational modal analysis
Mechanical Systems and Signal Processing(2022)
Abstract
This paper introduces a fast Gibbs sampler for solving a fully Bayesian problem in operational modal analysis. The proposed method is able to infer modal properties from the FFT of well-separated modes. System identification and related uncertainties are captured by a posterior distribution. The classical resonance description is wrapped into a hierarchical probabilistic model. The model is sampled through an enhanced Gibbs sampler including a Metropolis–Hasting step. The entire sampling scheme is new and the fast convergence of the algorithm is enabled by two strategies. First, the use of a collapsed Gibbs algorithm allows for an efficient sampling of the mode shape. Second, the use of adequate candidate distributions in the Metropolis–Hasting step provides excellent acceptance ratios, around 75%. Eventually, the numerical inference procedure is compared to the state-of-the-art Fast Bayesian FFT Algorithm (Fast-BFFTA), which is the most commonly used algorithm for Bayesian operational modal analysis. The sampler surpasses the Fast-BFFTA for small data-based identification, while remaining sustainable in terms of computing requirements.
MoreTranslated text
Key words
Operational modal analysis,Bayesian inference,Gibbs sampling,Uncertainty quantification,FFT approach
AI Read Science
Must-Reading Tree
Example
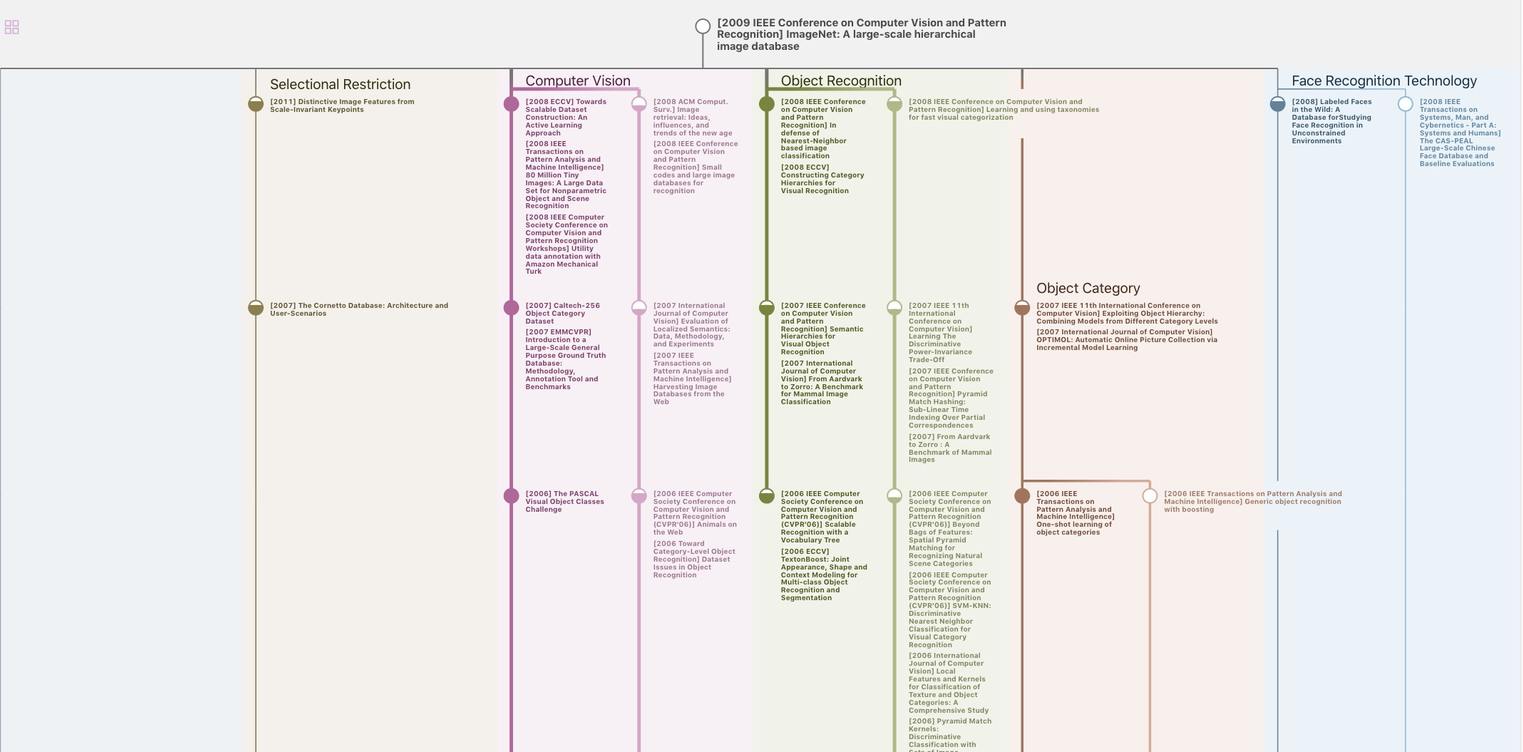
Generate MRT to find the research sequence of this paper
Chat Paper
Summary is being generated by the instructions you defined